概率论中指数分布介绍及C++11中std::exponential_distribution的使用
指数分布:在深度学习中,我们经常会需要一个在x=0点处取得边界点(sharp point)的分布。为了实现这一目的,我们可以使用指数分布(exponential distribution):
p(x;λ)= λlx≥0exp(-λx)
指数分布使用指示函数(indicator function) lx≥0来使得当x取负值时的概率为零。
指数分布:在概率论和统计学中,指数分布(Exponential distribution)是一种连续概率分布。指数分布可以用来表示独立随机事件发生的时间间隔,比如旅客进入机场的时间间隔、打进客服中心电话的时间间隔、中文维基百科新条目出现的时间间隔等等。
一个指数分布的概率密度函数是:
其中λ>0是分布的一个参数,常被称为率参数(rate parameter)。即每单位时间发生该事件的次数。指数分布的区间是[0,∞)。如果一个随机变量X呈指数分布,则可以写作:X∽Exponential(λ)。
指数分布的累积分布函数可以写成:
随机变量X(X的率参数是λ)的期望值是:E[X]=1/λ,比方说:如果你平均每个小时接到2次电话,那么你预期等待每一次电话的时间是半个小时。X的方差是:D[X]=1/λ2,X的偏离系数是:V[X]=1.
以下简单介绍Laplace分布、Dirac分布、经验分布、混合分布:
Laplace分布:一个联系紧密的概率分布是Laplace分布(Laplace distribution),它允许我们在任意一点μ处设置概率质量的峰值:
拉普拉斯分布:在概率论与统计学中,拉普拉斯分布是以皮埃尔-西蒙·拉普拉斯的名字命名的一种连续概率分布。由于它可以看作是两个不同位置的指数分布背靠背拼接在一起,所以它也叫做双指数分布。两个相互独立同概率分布指数随机变量之间的差别是按照指数分布的随机时间布朗运动,所以它遵循拉普拉斯分布。
如果随机变量的概率密度函数分布为:
那么它就是拉普拉斯分布。其中,μ是位置参数,b>0是尺度参数。如果μ=0,那么,正半部分恰好是尺度为1/2的指数分布。
拉普拉斯分布的概率密度函数让我们联想到正态分布,但是,正态分布是用相对于μ平均值的差的平方来表示,而拉普拉斯概率密度函数用相对于平均值的差的绝对值来表示。因此,拉普拉斯分布的尾部比正态分布更加平坦。
Boost 1.64.0库中实现了对Laplace分布的实现,在math/distributions/laplace.hpp文件中,对应类为laplace_distribution。
Dirac分布:在一些情况下,我们希望概率分布中的所有质量都集中在一个点上。这可以通过Dirac delta函数(Dirac delta function)δ(x)定义概率密度函数来实现:p(x)= δ(x-μ)
Dirac delta函数被定义成在除了0以外的所有点的值都为0,但是积分为1。Dirac delta函数不像普通函数一样对x的每一个值都有一个实数值的输出,它是一种不同类型的数学对象,被称为广义函数(generalized function),广义函数是依据积分性质定义的数学对象。我们可以把Dirac delta函数想成一系列函数的极限点,这一系列函数把除0以外的所有点的概率密度越变越小。
通过把p(x)定义成δ函数左移-μ个单位,我们得到了一个在x=μ处具有无限窄也无限高的峰值的概率质量。
狄拉克δ函数:在科学和数学中,狄拉克δ函数或简称δ函数是在实数线上定义的一个广义函数或分布。它在除零以外的点上都等于零,且其在整个定义域上的积分等于1。δ函数有时可看作是在原点处无限高、无限细,但是总面积为1 的一个尖峰,在物理上代表了理想化的质点或点电荷的密度。
从纯数学的观点来看,狄拉克δ函数并非严格意义上的函数,因为任何在扩展实数线上定义的函数,如果在一个点以外的地方都等于零,其总积分必须为零。δ函数只有在出现在积分以内的时候才有实质的意义。根据这一点,δ函数一般可以当做普通函数一样使用。它形式上所遵守的规则属于运算微积分的一部分,是物理学和工程学的标准工具。
δ函数的图形通常可以视为整条x轴和正y轴。虽然称为函数,但δ函数并非真正的函数,至少它的值域不在实数以内。
Dirac分布经常作为经验分布(empirical distribution)的一个组成部分出现:
经验分布将概率密度1/m赋给m个点x(1),…,x(m)中的每一个,这些点是给定的数据集或者采样的集合。只有在定义连续型随机变量的经验分布时,Dirac delta函数才是必要的。对于离散型随机变量,情况更加简单:经验分布可以被定义成一个Multinoulli分布,对于每一个可能的输入,其概率可以简单地设为在训练集上那个输入值的经验频率(empirical frequency)。
当我们在训练集上训练模型时,我们可以认为从这个训练集上得到的经验分布指明了我们采样来源的分布。关于经验分布另外一个重要的观点是,它是训练数据的似然最大的那个概率密度函数。
Empirical distribution function: In statistics, an empirical distribution function is the distribution function associated with the empirical measure of a sample. This cumulative distribution function is a step function that jumps up by 1/n at each of the n data points. Its value at any specified value of the measured variable is the fraction of observations of the measured variable that are less than or equal to the specified value.
The empirical distribution function is an estimate of the cumulative distribution function that generated the points in the sample. It converges with probability 1 to that underlying distribution, according to the Glivenko–Cantelli theorem.A number of results exist to quantify the rate of convergence of the empirical distribution function to the underlying cumulative distribution function.
分布的混合:通过组合一些简单的概率分布来定义新的概率分布也是很常见的。一种通用的组合方法是构造混合分布(mixture distribution)。混合分布由一些组件(component)分布构成。每次实验,样本是由哪个组件分布产生的取决于从一个Multinoulli分布中采样的结果:这里P(c)是对各组件的一个Multinoulli分布。
一个非常强大且常见的混合模型是高斯混合模型(Gaussian Mixture Model),它的组件p(x|c=i)是高斯分布。每个组件都有各自的参数,均值μ(i)和协方差矩阵∑(i)。有一些混合可以有更多的限制。和单个高斯分布一样,高斯混合模型有时会限制每个组件的协方差矩阵为对角的或者各向同性的(标量乘以单位矩阵)。
除了均值和协方差以外,高斯混合模型的参数指明了给每个组件i的先验概率(prior probability)αi=P(c=i)。”先验”一词表明了在观测到x之前传递给模型关于c的信念(belief)。作为对比,P(c|x)是后验概率(posterior probability),因为它是在观测到x之后进行计算的。高斯混合模型是概率密度的万能近似器(universal approximator),在这种意义上,任何平滑的概率密度都可以用具有足够多组件的高斯混合模型以任意精度来逼近。
Mixture distribution: In probability and statistics, a mixture distribution is the probability distribution of a random variable that is derived from a collection of other random variables as follows: first, a random variable is selected by chance from the collection according to given probabilities of selection, and then the value of the selected random variable is realized. The underlying random variables may be random real numbers, or they may be random vectors (each having the same dimension), in which case the mixture distribution is a multivariate distribution.
以上内容摘自:《深度学习中文版》和 维基百科
以下是对C++11中的指数分布模板类std::exponential_distribution的介绍:
C++11中引入了指数分布(Exponential distribution)模板类std::exponential_distribution.指数分布是一个连续概率分布,产生随机非负浮点数,可以用来表示独立随机事件发生的时间间隔。
std::exponential_distribution:Random number distribution that produces floating-point values according to an exponential distribution, which is described by the following probability density function: p(x|λ)= λe-λx, x>0
This distribution produces random numbers where each value represents the interval between two random events that are independent but statistically defined by a constant average rate of occurrence (its lambda, λ).The distribution parameter, lambda, is set on construction. To produce a random value following this distribution, call its member function operator().Its analogous discrete distribution is the geometric_distribution.
下面是从其它文章中copy的std::exponential_distribution测试代码,详细内容介绍可以参考对应的reference:
#include "exponential_distribution.hpp"
#include <iostream>
#include <random>
#include <string>
#include <chrono>
#include <thread>
#include <iomanip>
#include <map>///
// reference: http://www.cplusplus.com/reference/random/exponential_distribution/
int test_exponential_distribution_1()
{
{const int nrolls = 10000; // number of experimentsconst int nstars = 100; // maximum number of stars to distributeconst int nintervals = 10; // number of intervalsstd::default_random_engine generator;std::exponential_distribution<double> distribution(3.5);int p[nintervals] = {};for (int i = 0; i<nrolls; ++i) {double number = distribution(generator);if (number<1.0) ++p[int(nintervals*number)];}std::cout << "exponential_distribution (3.5):" << std::endl;std::cout << std::fixed; std::cout.precision(1);for (int i = 0; i<nintervals; ++i) {std::cout << float(i) / nintervals << "-" << float(i + 1) / nintervals << ": ";std::cout << std::string(p[i] * nstars / nrolls, '*') << std::endl;}
}{ // (1)、exponential_distribution::exponential_distribution: Construct exponential distribution,
// Constructs an exponential_distribution object, adopting the distribution parameters specified either by lambda or by object parm.
// (2)、exponential_distribution::lambda: Returns the parameter lambda (λ) associated with the exponential_distribution.
// This parameter represents the number of times the random events are observed by interval, on average.
// This parameter is set on construction.
// (3)、exponential_distribution::max: Maximum value
// Returns the least upper bound of the range of values potentially returned by member operator().
// (4)、exponential_distribution::min: Minimum value
// Returns the greatest lower bound of the range of values potentially returned by member operator(),
// which for exponential_distribution is always zero.
// (5)、exponential_distribution::operator(): Generate random number
// Returns a new random number that follows the distribution's parameters associated to the object (version 1)
// or those specified by parm (version 2).// construct a trivial random generator engine from a time-based seed:int seed = std::chrono::system_clock::now().time_since_epoch().count();std::default_random_engine generator(seed);std::exponential_distribution<double> distribution(1.0);std::cout << "ten beeps, spread by 1 second, on average: " << std::endl;for (int i = 0; i<10; ++i) {double number = distribution(generator);std::chrono::duration<double> period(number);std::this_thread::sleep_for(std::chrono::seconds(1)/*period*/);std::cout << "beep!" << std::endl;}std::cout << "lambda: " << distribution.lambda() << std::endl;std::cout << "max: " << distribution.max() << std::endl;std::cout << "min: " << distribution.min() << std::endl;
}{ // exponential_distribution::param: Distribution parameters
// The first version(1) returns an object with the parameters currently associated with the distribution object.
// The second version(2) associates the parameters in object parm to the distribution object.std::default_random_engine generator;std::exponential_distribution<double> d1(0.8);std::exponential_distribution<double> d2(d1.param());// print two independent values:std::cout << d1(generator) << std::endl;std::cout << d2(generator) << std::endl;
}{ // exponential_distribution::reset: Resets the distribution, so that subsequent uses of the object do not depend on values already produced by it.
// This function may have no effect if the library implementation for this distribution class produces independent values.std::default_random_engine generator;std::exponential_distribution<double> distribution(1.0);// print two independent values:std::cout << distribution(generator) << std::endl;distribution.reset();std::cout << distribution(generator) << std::endl;
}return 0;
}// reference: http://en.cppreference.com/w/cpp/numeric/random/exponential_distribution
int test_exponential_distribution_2()
{std::random_device rd;std::mt19937 gen(rd());// if particles decay once per second on average,// how much time, in seconds, until the next one?std::exponential_distribution<> d(1);std::map<int, int> hist;for (int n = 0; n<10000; ++n) {++hist[2 * d(gen)];}for (auto p : hist) {std::cout << std::fixed << std::setprecision(1)<< p.first / 2.0 << '-' << (p.first + 1) / 2.0 <<' ' << std::string(p.second / 200, '*') << '\n';}return 0;
}// reference: https://msdn.microsoft.com/en-us/library/bb982914.aspx
static void test(const double l, const int s)
{// uncomment to use a non-deterministic generator// std::random_device gen;std::mt19937 gen(1701);std::exponential_distribution<> distr(l);std::cout << std::endl;std::cout << "min() == " << distr.min() << std::endl;std::cout << "max() == " << distr.max() << std::endl;std::cout << "lambda() == " << std::fixed << std::setw(11) << std::setprecision(10) << distr.lambda() << std::endl;// generate the distribution as a histogram std::map<double, int> histogram;for (int i = 0; i < s; ++i) {++histogram[distr(gen)];}// print results std::cout << "Distribution for " << s << " samples:" << std::endl;int counter = 0;for (const auto& elem : histogram) {std::cout << std::fixed << std::setw(11) << ++counter << ": "<< std::setw(14) << std::setprecision(10) << elem.first << std::endl;}std::cout << std::endl;
}
int test_exponential_distribution_3()
{double l_dist = 0.5;int samples = 10;std::cout << "Use CTRL-Z to bypass data entry and run using default values." << std::endl;std::cout << "Enter a floating point value for the 'lambda' distribution parameter (must be greater than zero): ";//std::cin >> l_dist;std::cout << "Enter an integer value for the sample count: ";//std::cin >> samples;test(l_dist, samples);return 0;
}
GitHub: https://github.com/fengbingchun/Messy_Test 相关文章:
肖仰华:知识图谱构建的三要素、三原则和九大策略 | AI ProCon 2019
演讲嘉宾 | 肖仰华(复旦大学教授、博士生导师,知识工场实验室负责人) 编辑 | Jane 出品 | AI科技大本营(ID:rgznai100) 近两年,知识图谱技术得到了各行各业的关注,无论是企业公司还…
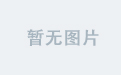
Docker mongo副本集环境搭建
1、MongoDB Docker 镜像安装 docker pull mongo 2、Docker容器创建 MongoDB Docker 容器创建有以下几个问题: 1- MongoDB 容器基本创建方法和数据目录挂载 2- MongoDB 容器的数据迁移 3- MongoDB 设置登录权限问题docker run -p 27017:27017 -v <LocalDirectoryP…
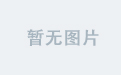
菜鸟学习HTML5+CSS3(一)
主要内容: 1.新的文档类型声明(DTD) 2.新增的HTML5标签 3.删除的HTML标签 4.重新定义的HTML标签 一、新的文档类型声明(DTD) HTML 5的DTD声明为:<!doctype html>、<!DOCTYPE html>、<!DOCTY…
激活函数之logistic sigmoid函数介绍及C++实现
logistic sigmoid函数:logistic sigmoid函数通常用来产生Bernoulli分布中的参数,因为它的范围是(0,1),处在的有效取值范围内。logisitic sigmoid函数在变量取绝对值非常大的正值或负值时会出现饱和(saturate)现象,意味着函数会变得…
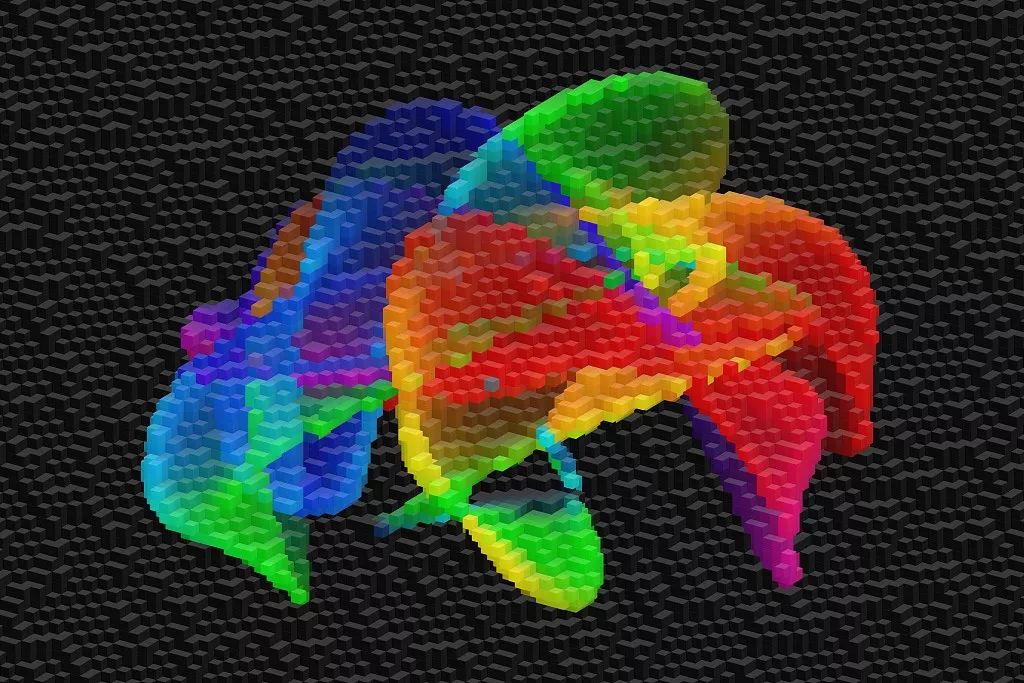
NLP重要模型详解,换个方式学(内附资源)
(图片有AI科技大本营付费下载自视觉中国)作者 | Jaime Zornoza,马德里技术大学译者 | 陈之炎校对 | 王威力编辑 | 黄继彦来源 | 数据派THU(ID:DatapiTHU)【导语】本文带你以前所未有的方式了解深度学习神经…
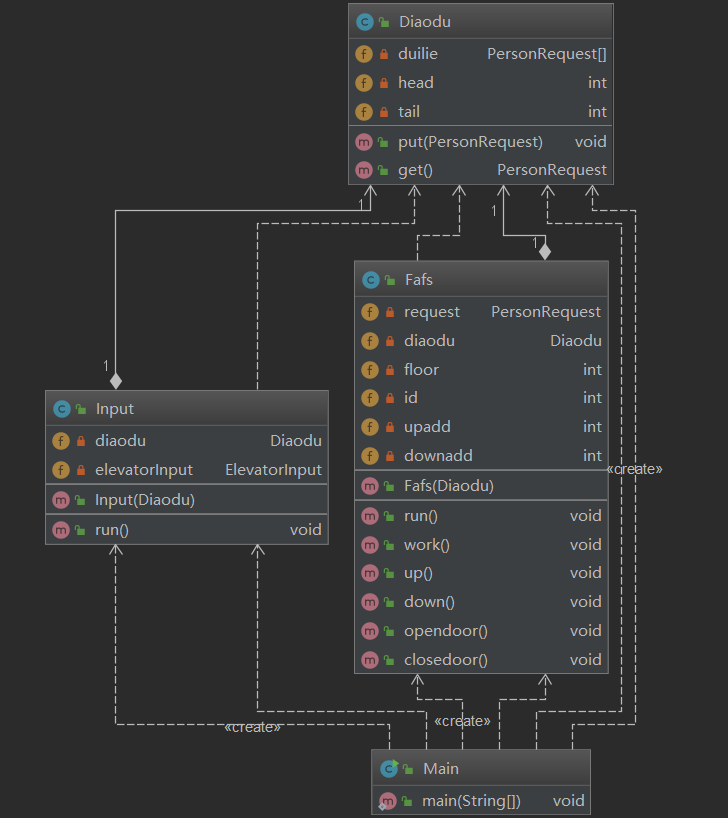
大闸蟹的OO第二单元总结
OO的第二单元是讲多线程的协作与控制,三次作业分别为FAFS电梯,ALS电梯和三部需要协作的电梯。三次作业由浅入深,让我们逐渐理解多线程的工作原理和运行状况。 第一次作业: 第一次作业是傻瓜电梯,也就是完全不需要考虑捎…

构建ASP.NET MVC4+EF5+EasyUI+Unity2.x注入的后台管理系统(31)-MVC使用RDL报表
原文:构建ASP.NET MVC4EF5EasyUIUnity2.x注入的后台管理系统(31)-MVC使用RDL报表这次我们来演示MVC3怎么显示RDL报表,坑爹的微软把MVC升级到5都木有良好的支持报表,让MVC在某些领域趋于短板 我们只能通过一些方式来使用rdl报表。 Razor视图不支持asp.net…
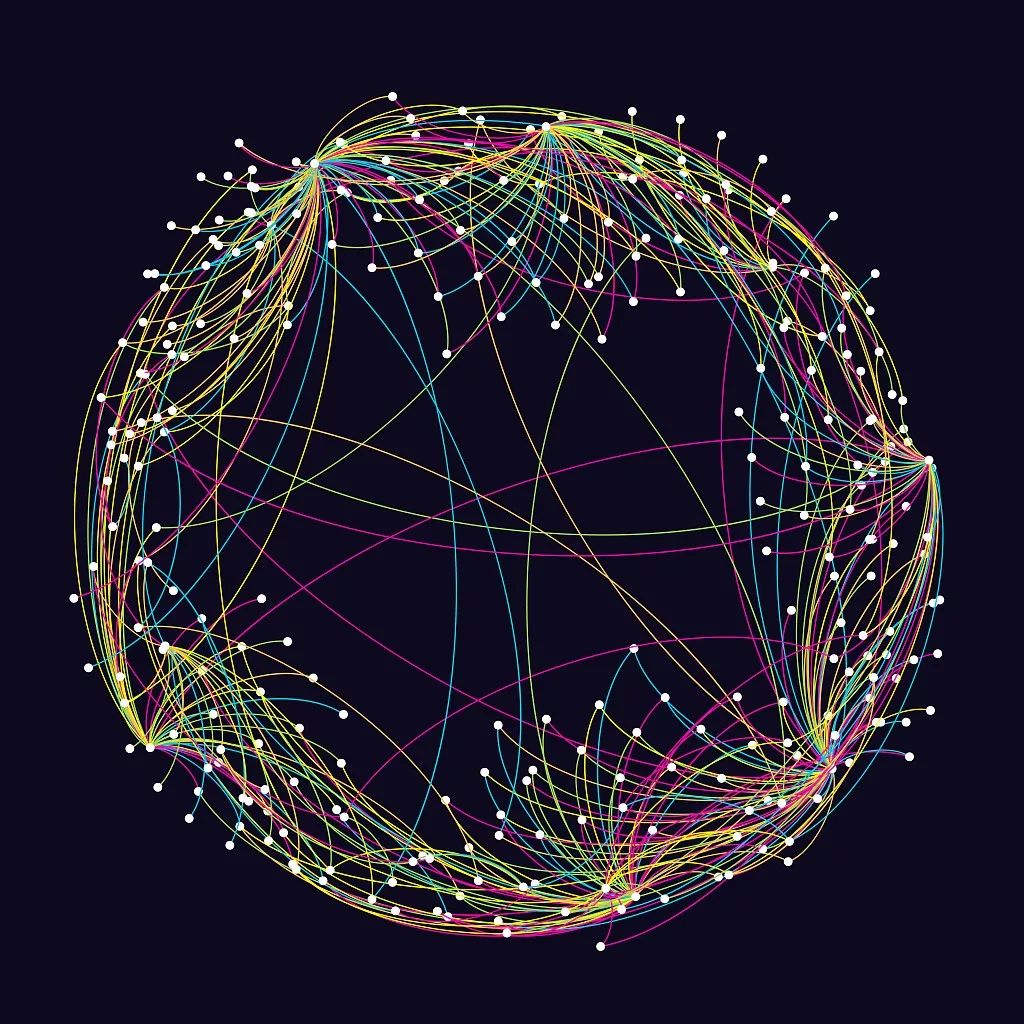
18段代码带你玩转18个机器学习必备交互工具
(图片有AI科技大本营付费下载自视觉中国)作者 | 曼纽尔阿米纳特吉(Manuel Amunategui)、迈赫迪洛佩伊(Mehdi Roopaei)来源 | 大数据(ID:hzdashuju)【导读】本文简要介绍将…
激活函数之ReLU/softplus介绍及C++实现
softplus函数(softplus function):ζ(x)ln(1exp(x)).softplus函数可以用来产生正态分布的β和σ参数,因为它的范围是(0,∞)。当处理包含sigmoid函数的表达式时它也经常出现。softplus函数名字来源于它是另外一个函数的平滑(或”软化”)形式,这…
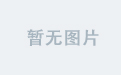
windows server 2012 用sysdba登录报错 ORA-01031
报错显示:C:\Users\Administrator>sqlplus / as sysdba SQL*Plus: Release 11.2.0.1.0 Production on 星期三 4月 24 09:09:33 2019 Copyright (c) 1982, 2010, Oracle. All rights reserved. ERROR:ORA-01031: 权限不足 请输入用户名: 1、查看本地用户和组确认权…

[SignalR]初步认识以及安装
原文:[SignalR]初步认识以及安装1.什么是ASP.NET SignalR? ASP .NET SignalR是一个 ASP .NET 下的类库,可以在ASP .NET 的Web项目中实现实时通信。什么是实时通信的Web呢?就是让客户端(Web页面)和服务器端可以互相通知…
CUDA Samples:Vector Add
以下CUDA sample是分别用C和CUDA实现的两向量相加操作,参考CUDA 8.0中的sample:C:\ProgramData\NVIDIA Corporation\CUDA Samples\v8.0\0_Simple,并对其中使用到的CUDA函数进行了解说,各个文件内容如下:common.hpp:#ifndef FBC_CU…
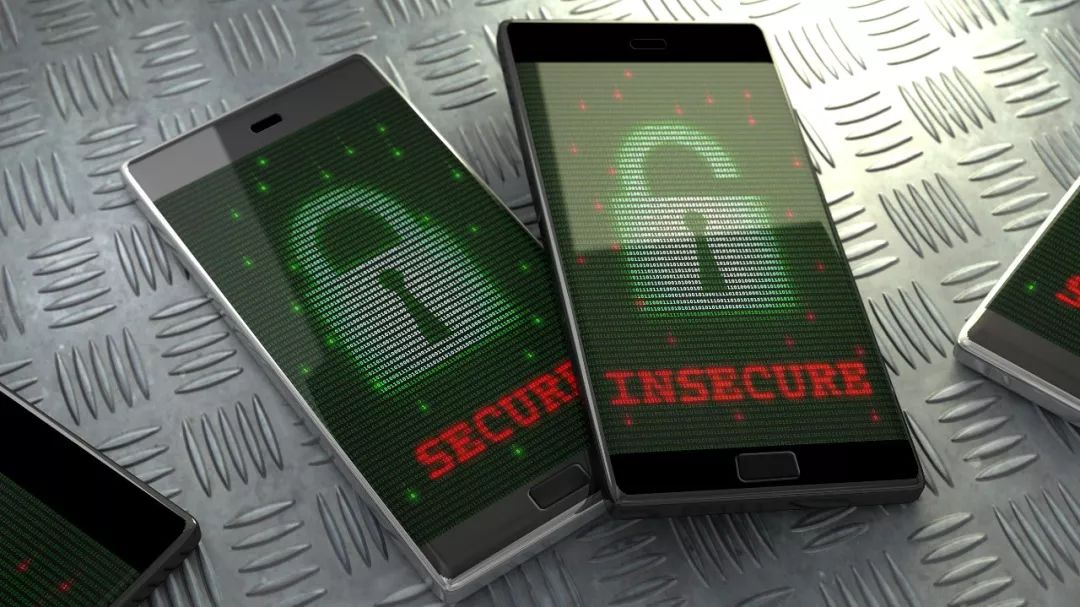
你和人工智能的对话,正在被人工收听
(图片有AI科技大本营付费下载自视觉中国)作者 | 周晶晶编辑 | 阿伦来源 | 燃财经(ID:rancaijing)如今,智能设备越来越多地出现在每个人的生活中,在享受它们带来的便利时,很多人或许没有意识到&a…

python数据结构与算法总结
python常用的数据结构与算法就分享到此处,本月涉及数据结构与算法的内容有如下文章: 《数据结构和算法对python意味着什么?》 《顺序表数据结构在python中的应用》 《python实现单向链表数据结构及其基本方法》 《python实现单向循环链表数据…

自定义classloader中的接口调用
2019独角兽企业重金招聘Python工程师标准>>> 注意其中转型异常的描述,左边声明和强转括号内都是appclassloader加载的,而让自定义加载类的接口也由appclassloader加载,所以转型成功 转载于:https://my.oschina.net/heatonn1/blog/…
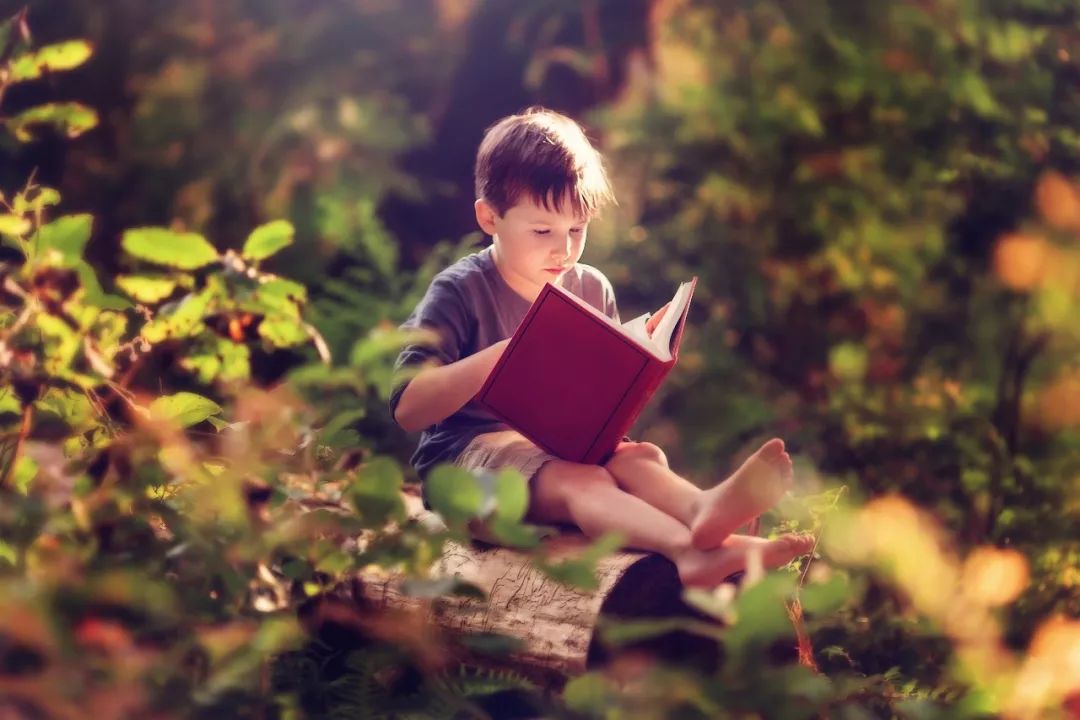
学点基本功:机器学习常用损失函数小结
(图片付费下载自视觉中国)作者 | 王桂波转载自知乎用户王桂波【导读】机器学习中的监督学习本质上是给定一系列训练样本 ,尝试学习 的映射关系,使得给定一个 ,即便这个不在训练样本中,也能够得到尽量接近…

python生成简单的FTP弱口令扫描
2019独角兽企业重金招聘Python工程师标准>>> 前言 Ftp这个类实现了Ftp客户端的大多数功能,比如连接Ftp服务器、查看服务器中的文件、上传、下载文件等功能,Ftp匿名扫描器的实现,需要使用FTP这个类,首先用主机名构造了一个Ftp对象(即ftp),然后用这个ftp调…
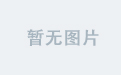
C++中const指针用法汇总
这里以int类型为例,进行说明,在C中const是类型修饰符:int a; 定义一个普通的int类型变量a,可对此变量的值进行修改。const int a 3;与 int const a 3; 这两条语句都是有效的code,并且是等价的,说明a是一个…
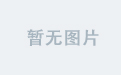
mongodb基础应用
一些概念 一个mongod服务可以有建立多个数据库,每个数据库可以有多张表,这里的表名叫collection,每个collection可以存放多个文档(document),每个文档都以BSON(binary json)的形式存…
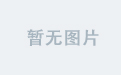
【leetcode】1030. Matrix Cells in Distance Order
题目如下: We are given a matrix with R rows and C columns has cells with integer coordinates (r, c), where 0 < r < R and 0 < c < C. Additionally, we are given a cell in that matrix with coordinates (r0, c0). Return the coordinates of…
深度学习面临天花板,亟需更可信、可靠、安全的第三代AI技术|AI ProCon 2019
整理 | 夕颜 出品 | AI科技大本营(ID:rgznai100) 在人工智能领域中,深度学习掀起了最近一次浪潮,但在实践和应用中也面临着诸多挑战,特别是关系到人的生命,如医疗、自动驾驶等领域场景时,黑盒…

java robot类自动截屏
直接上代码:package robot;import java.awt.Rectangle;import java.awt.Robot;import java.awt.event.InputEvent;import java.awt.p_w_picpath.BufferedImage;import java.io.File;import java.io.IOException;import javax.p_w_picpathio.ImageIO;import com.sun.glass.event…
激活函数之softmax介绍及C++实现
下溢(underflow):当接近零的数被四舍五入为零时发生下溢。许多函数在其参数为零而不是一个很小的正数时才会表现出质的不同。例如,我们通常要避免被零除或避免取零的对数。上溢(overflow):当大量级的数被近似为∞或-∞时发生上溢。进一步的运…
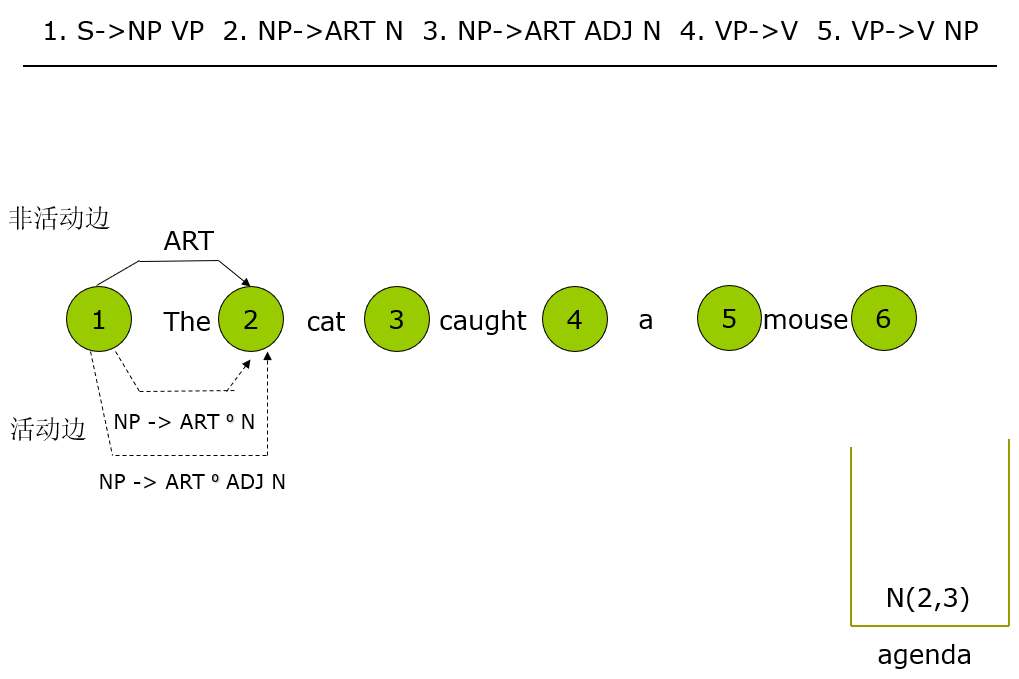
parsing:NLP之chart parser句法分析器
已迁移到我新博客,阅读体验更佳parsing:NLP之chart parser句法分析器 完整代码实现放在我的github上:click me 一、任务要求 实现一个基于简单英语语法的chart句法分析器。二、技术路线 采用自底向上的句法分析方法,简单的自底向上句法分析效率不高,常常…
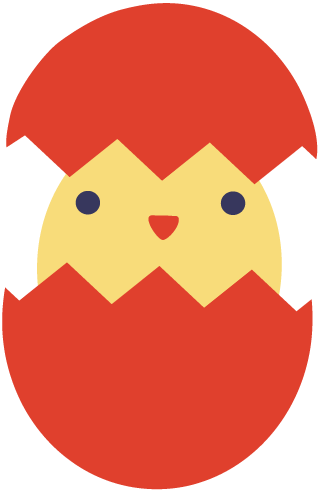
图解Python算法
普通程序员,不学算法,也可以成为大神吗?对不起,这个,绝对不可以。可是算法好难啊~~看两页书就想睡觉……所以就不学了吗?就一直当普通程序员吗?如果有一本算法书,看着很轻松……又有…
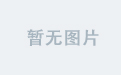
详解SSH框架的原理和优点
Struts的原理和优点. Struts工作原理 MVC即Model-View-Controller的缩写,是一种常用的设计模式。MVC 减弱了业务逻辑接口和数据接口之间的耦合,以及让视图层更富于变化。MVC的工作原理,如下图1所示:Struts 是MVC的一种实现࿰…

Numpy and Matplotlib
Numpy介绍 编辑 一个用python实现的科学计算,包括:1、一个强大的N维数组对象Array;2、比较成熟的(广播)函数库;3、用于整合C/C和Fortran代码的工具包;4、实用的线性代数、傅里叶变换和随机数生成…
梯度下降法简介
条件数表征函数相对于输入的微小变化而变化的快慢程度。输入被轻微扰动而迅速改变的函数对于科学计算来说可能是有问题的,因为输入中的舍入误差可能导致输出的巨大变化。大多数深度学习算法都涉及某种形式的优化。优化指的是改变x以最小化或最大化某个函数f(x)的任务…
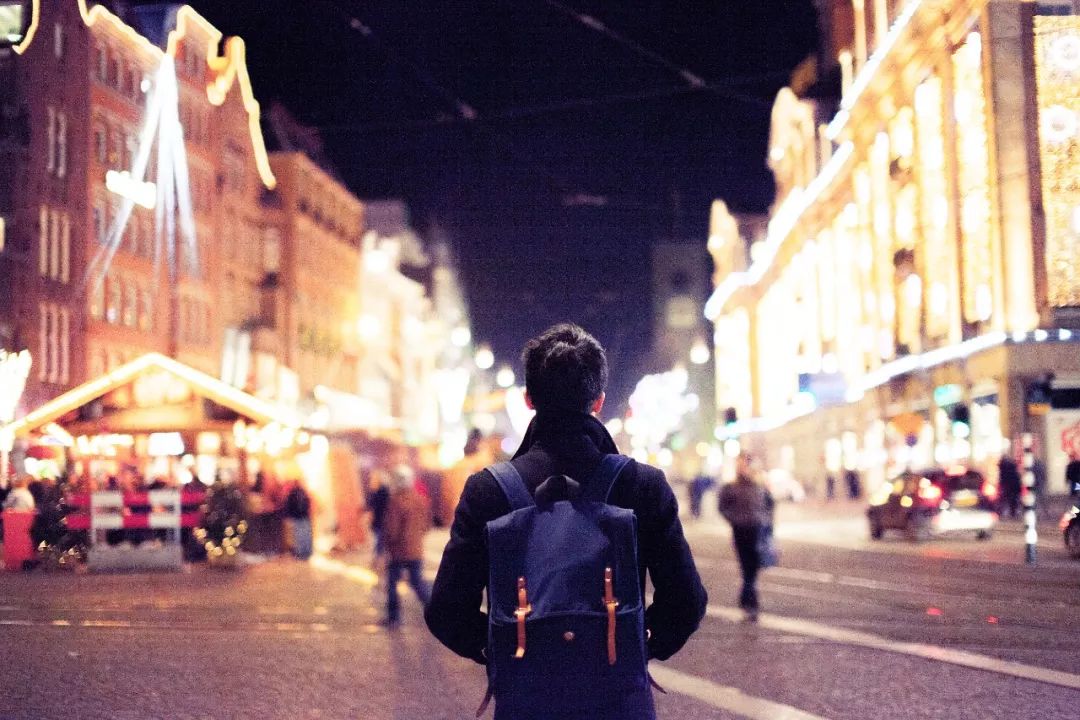
微软亚研院CV大佬代季峰跳槽商汤为哪般?
整理 | 夕颜出品 | AI科技大本营(ID:rgznai100)近日,知乎上一篇离开关于MSRA(微软亚洲研究院)和MSRA CV未来发展的帖子讨论热度颇高,这个帖子以MSRA CV执行研究主任代季峰离职加入商汤为引子,引…

iOS Block实现探究
2019独角兽企业重金招聘Python工程师标准>>> 使用clang的rewrite-objc filename 可以将有block的c代码转换成cpp代码。从中可以看到block的实现。 #include <stdio.h> int main() {void (^blk)(void) ^{printf("Block\n");};blk();return 0; } 使…