K-最近邻法(KNN) C++实现
关于KNN的介绍可以参考: http://blog.csdn.net/fengbingchun/article/details/78464169
这里给出KNN的C++实现,用于分类。训练数据和测试数据均来自MNIST,关于MNIST的介绍可以参考: http://blog.csdn.net/fengbingchun/article/details/49611549 , 从MNIST中提取的40幅图像,0,1,2,3四类各20张,每类的前10幅来自于训练样本,用于训练,后10幅来自测试样本,用于测试,如下图:
实现代码如下:
knn.hpp:
#ifndef FBC_NN_KNN_HPP_
#define FBC_NN_KNN_HPP_#include <memory>
#include <vector>namespace ANN {template<typename T>
class KNN {
public:KNN() = default;void set_k(int k);int set_train_samples(const std::vector<std::vector<T>>& samples, const std::vector<T>& labels);int predict(const std::vector<T>& sample, T& result) const;private:int k = 3;int feature_length = 0;int samples_number = 0;std::unique_ptr<T[]> samples;std::unique_ptr<T[]> labels;
};} // namespace ANN#endif // FBC_NN_KNN_HPP_
knn.cpp:#include "knn.hpp"
#include <limits>
#include <algorithm>
#include <functional>
#include "common.hpp"namespace ANN {template<typename T>
void KNN<T>::set_k(int k)
{this->k = k;
}template<typename T>
int KNN<T>::set_train_samples(const std::vector<std::vector<T>>& samples, const std::vector<T>& labels)
{CHECK(samples.size() == labels.size());this->samples_number = samples.size();if (this->k > this->samples_number) this->k = this->samples_number;this->feature_length = samples[0].size();this->samples.reset(new T[this->feature_length * this->samples_number]);this->labels.reset(new T[this->samples_number]);T* p = this->samples.get();for (int i = 0; i < this->samples_number; ++i) {T* q = p + i * this->feature_length;for (int j = 0; j < this->feature_length; ++j) {q[j] = samples[i][j];}this->labels.get()[i] = labels[i];}
}template<typename T>
int KNN<T>::predict(const std::vector<T>& sample, T& result) const
{if (sample.size() != this->feature_length) {fprintf(stderr, "their feature length dismatch: %d, %d", sample.size(), this->feature_length);return -1;}typedef std::pair<T, T> value;std::vector<value> info;for (int i = 0; i < this->k + 1; ++i) {info.push_back(std::make_pair(std::numeric_limits<T>::max(), (T)-1.));}for (int i = 0; i < this->samples_number; ++i) {T s{ 0. };const T* p = this->samples.get() + i * this->feature_length;for (int j = 0; j < this->feature_length; ++j) {s += (p[j] - sample[j]) * (p[j] - sample[j]);}info[this->k] = std::make_pair(s, this->labels.get()[i]);std::stable_sort(info.begin(), info.end(), [](const std::pair<T, T>& p1, const std::pair<T, T>& p2) {return p1.first < p2.first; });}std::vector<T> vec(this->k);for (int i = 0; i < this->k; ++i) {vec[i] = info[i].second;}std::sort(vec.begin(), vec.end(), std::greater<T>());vec.erase(std::unique(vec.begin(), vec.end()), vec.end());std::vector<std::pair<T, int>> ret;for (int i = 0; i < vec.size(); ++i) {ret.push_back(std::make_pair(vec[i], 0));}for (int i = 0; i < this->k; ++i) {for (int j = 0; j < ret.size(); ++j) {if (info[i].second == ret[j].first) {++ret[j].second;break;}}}int max = -1, index = -1;for (int i = 0; i < ret.size(); ++i) {if (ret[i].second > max) {max = ret[i].second;index = i;}}result = ret[index].first;return 0;
}template class KNN<float>;
template class KNN<double>;} // namespace ANN
测试代码如下:#include "funset.hpp"
#include <iostream>
#include "perceptron.hpp"
#include "BP.hpp""
#include "CNN.hpp"
#include "linear_regression.hpp"
#include "naive_bayes_classifier.hpp"
#include "logistic_regression.hpp"
#include "common.hpp"
#include "knn.hpp"
#include <opencv2/opencv.hpp>// =========================== KNN(K-Nearest Neighbor) ======================
int test_knn_classifier_predict()
{const std::string image_path{ "E:/GitCode/NN_Test/data/images/digit/handwriting_0_and_1/" };const int K{ 3 };cv::Mat tmp = cv::imread(image_path + "0_1.jpg", 0);const int train_samples_number{ 40 }, predict_samples_number{ 40 };const int every_class_number{ 10 };cv::Mat train_data(train_samples_number, tmp.rows * tmp.cols, CV_32FC1);cv::Mat train_labels(train_samples_number, 1, CV_32FC1);float* p = (float*)train_labels.data;for (int i = 0; i < 4; ++i) {std::for_each(p + i * every_class_number, p + (i + 1)*every_class_number, [i](float& v){v = (float)i; });}// train datafor (int i = 0; i < 4; ++i) {static const std::vector<std::string> digit{ "0_", "1_", "2_", "3_" };static const std::string suffix{ ".jpg" };for (int j = 1; j <= every_class_number; ++j) {std::string image_name = image_path + digit[i] + std::to_string(j) + suffix;cv::Mat image = cv::imread(image_name, 0);CHECK(!image.empty() && image.isContinuous());image.convertTo(image, CV_32FC1);image = image.reshape(0, 1);tmp = train_data.rowRange(i * every_class_number + j - 1, i * every_class_number + j);image.copyTo(tmp);}}ANN::KNN<float> knn;knn.set_k(K);std::vector<std::vector<float>> samples(train_samples_number);std::vector<float> labels(train_samples_number);const int feature_length{ tmp.rows * tmp.cols };for (int i = 0; i < train_samples_number; ++i) {samples[i].resize(feature_length);const float* p1 = train_data.ptr<float>(i);float* p2 = samples[i].data();memcpy(p2, p1, feature_length * sizeof(float));}const float* p1 = (const float*)train_labels.data;float* p2 = labels.data();memcpy(p2, p1, train_samples_number * sizeof(float));knn.set_train_samples(samples, labels);// predict dattacv::Mat predict_data(predict_samples_number, tmp.rows * tmp.cols, CV_32FC1);for (int i = 0; i < 4; ++i) {static const std::vector<std::string> digit{ "0_", "1_", "2_", "3_" };static const std::string suffix{ ".jpg" };for (int j = 11; j <= every_class_number + 10; ++j) {std::string image_name = image_path + digit[i] + std::to_string(j) + suffix;cv::Mat image = cv::imread(image_name, 0);CHECK(!image.empty() && image.isContinuous());image.convertTo(image, CV_32FC1);image = image.reshape(0, 1);tmp = predict_data.rowRange(i * every_class_number + j - 10 - 1, i * every_class_number + j - 10);image.copyTo(tmp);}}cv::Mat predict_labels(predict_samples_number, 1, CV_32FC1);p = (float*)predict_labels.data;for (int i = 0; i < 4; ++i) {std::for_each(p + i * every_class_number, p + (i + 1)*every_class_number, [i](float& v){v = (float)i; });}std::vector<float> sample(feature_length);int count{ 0 };for (int i = 0; i < predict_samples_number; ++i) {float value1 = ((float*)predict_labels.data)[i];float value2;memcpy(sample.data(), predict_data.ptr<float>(i), feature_length * sizeof(float));CHECK(knn.predict(sample, value2) == 0);fprintf(stdout, "expected value: %f, actual value: %f\n", value1, value2);if (int(value1) == int(value2)) ++count;}fprintf(stdout, "when K = %d, accuracy: %f\n", K, count * 1.f / predict_samples_number);return 0;
}
执行结果如下:与OpenCV中KNN结果相似。GitHub: https://github.com/fengbingchun/NN_Test
相关文章:
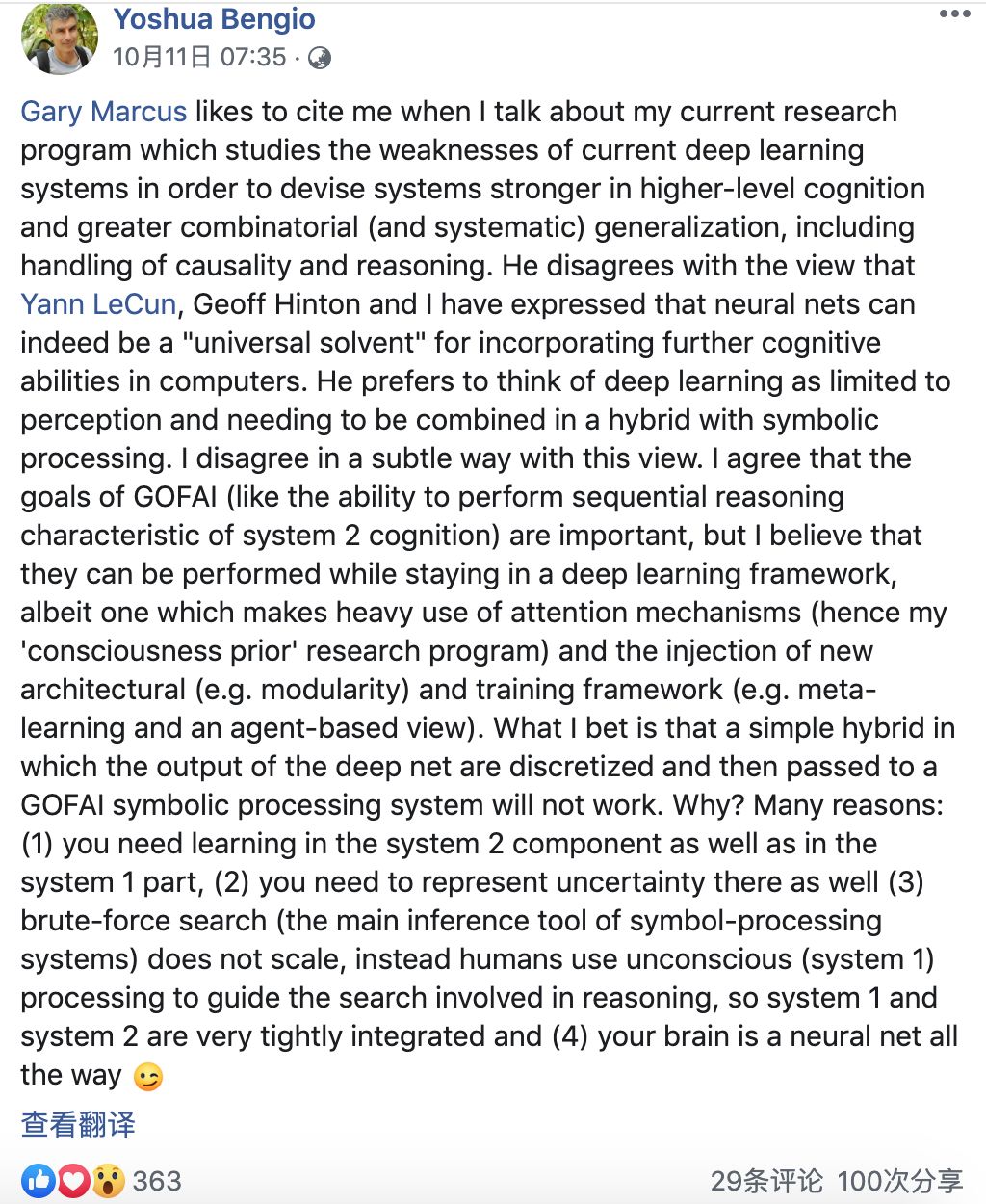
AI大佬“互怼”:Bengio和Gary Marcus隔空对谈深度学习发展现状
编译 | AI科技大本营编辑部出品 | AI科技大本营(ID:rgznai100)去年以来,由于纽约大学教授 Gary Marcus 对深度学习批评,导致他在社交媒体上与许多知名的 AI 研究人员如 Facebook 首席 AI 科学家 Yann LeCun 进行了一场论战。不止 …
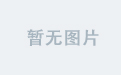
Centos7多内核情况下修改默认启动内核方法
1.1 进入grub.cfg配置文件存放目录/boot/grub2/并备份grub.cfg配置文件 [rootlinux-node1 ~]# cd /boot/grub2/ [rootlinux-node1 grub2]# cp -p grub.cfg grub.cfg.bak [rootlinux-node1 grub2]# ls -ld grub.cfg* -rw-r--r--. 1 root root 5162 Aug 11 2018 grub.cfg -rw-r…
TensorRT Samples: MNIST
关于TensorRT的介绍可以参考: http://blog.csdn.net/fengbingchun/article/details/78469551以下是参考TensorRT 2.1.2中的sampleMNIST.cpp文件改写的实现对手写数字0-9识别的测试代码,各个文件内容如下:common.hpp:#ifndef FBC_TENSORRT_TE…
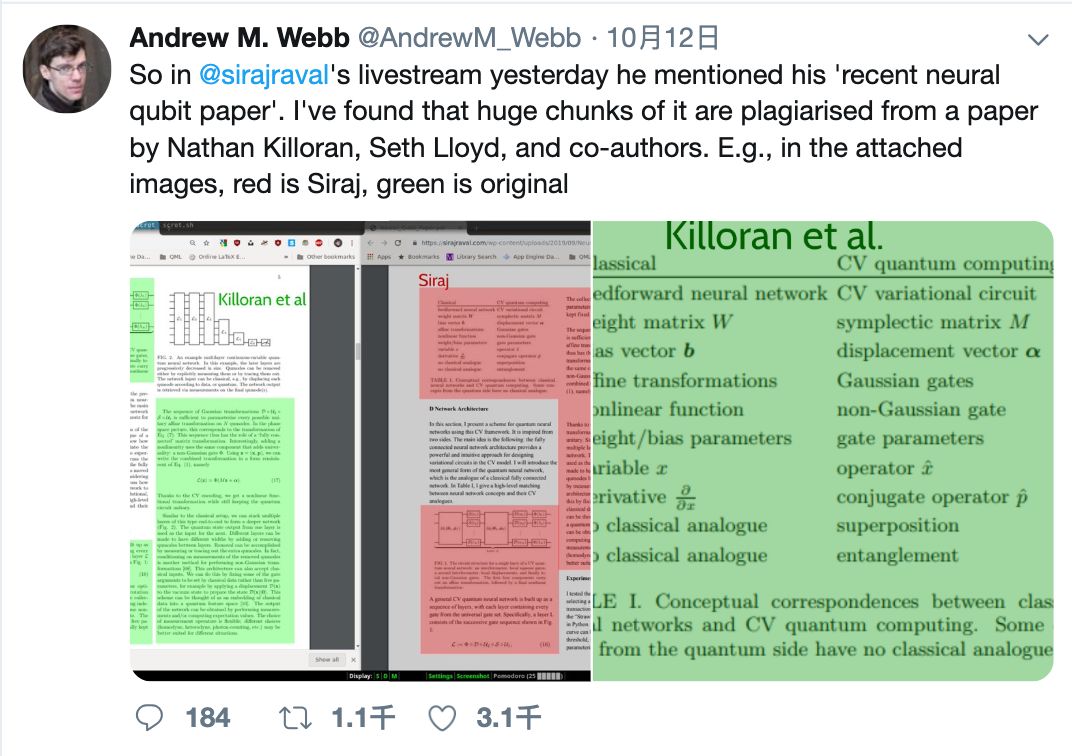
网红“AI大佬”被爆论文剽窃,Jeff Dean都看不下去了
作者 | 夕颜、Just出品 | AI科技大本营(ID:rgznai100)【导读】近日,推特上一篇揭露 YouTube 网红老师 Siraj Raval 新发表论文涉抄袭其他学者的帖子引起了讨论。揭露者是曼彻斯特大学计算机科学系研究员 Andrew M. Webb,他在 Twit…
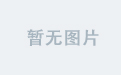
数位dp(求1-n中数字1出现的个数)
题意:求1-n的n个数字中1出现的个数。 解法:数位dp,dp[pre][now][equa] 记录着第pre位为now,equa表示前边是否有降数字(即后边可不能够任意取,true为没降,true为已降);常规的记忆化搜…
TensorRT Samples: MNIST API
关于TensorRT的介绍可以参考: http://blog.csdn.net/fengbingchun/article/details/78469551 以下是参考TensorRT 2.1.2中的sampleMNISTAPI.cpp文件改写的实现对手写数字0-9识别的测试代码,各个文件内容如下:common.hpp:#ifndef FBC_TENSORR…
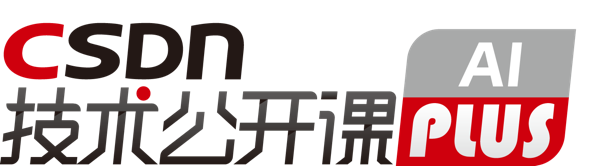
免费学习AI公开课:打卡、冲击排行榜,还有福利领取
CSDN 技术公开课 Plus--AI公开课再度升级内容全新策划:贴近开发者,更多样、更落地形式多样升级:线上线下、打卡学习,资料福利,共同交流成长,扫描下方小助手二维码,回复:公开课&#…
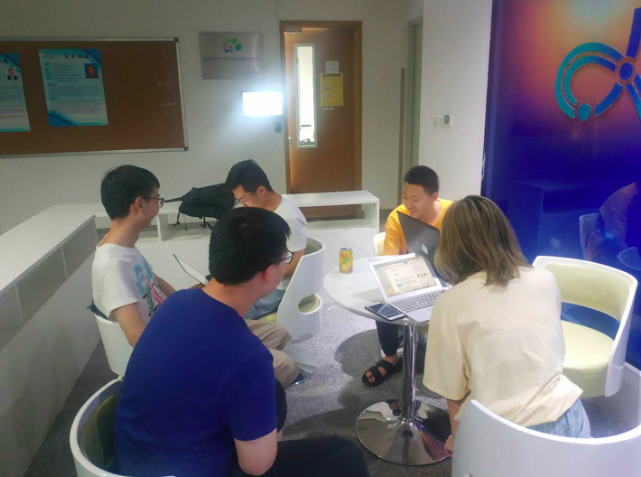
Gamma阶段第一次scrum meeting
每日任务内容 队员昨日完成任务明日要完成的任务张圆宁#91 用户体验与优化:发现用户体验细节问题https://github.com/rRetr0Git/rateMyCourse/issues/91#91 用户体验与优化:发现并优化用户体验,修复问题https://github.com/rRetr0Git/rateMyC…
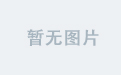
windows 切换 默认 jdk 版本
set JAVA_HOMEC:\jdk1.6.0u24 set PATH%JAVA_HOME%\bin;%PATH%转载于:https://www.cnblogs.com/dmdj/p/3756887.html
TensorRT Samples: GoogleNet
关于TensorRT的介绍可以参考: http://blog.csdn.net/fengbingchun/article/details/78469551 以下是参考TensorRT 2.1.2中的sampleGoogleNet.cpp文件改写的测试代码,文件(googlenet.cpp)内容如下:#include <iostream> #include <t…
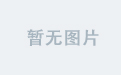
Visual Studio Code Go 插件文档翻译
此插件为 Go 语言在 VS Code 中开发提供了多种语言支持。 阅读版本变更日志了解此插件过去几个版本的更改内容。 1. 语言功能 (Language Features) 1.1 智能感知 (IntelliSense) 编码时符号自动补全(使用 gocode )编码时函数签名帮助提示(使用…
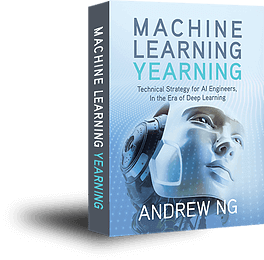
资源 | 吴恩达《机器学习训练秘籍》中文版58章节完整开源
整理 | Jane出品 | AI科技大本营(ID:rgznai100)一年前,吴恩达老师的《Machine Learning Yearning》(机器学习训练秘籍)中文版正式发布,经过一年多的陆续更新,近日,这本书的中文版 58…

js字符串加密的几种方法
在做web前端的时候免不了要用javascript来处理一些简单操作,其实如果要用好JQuery, Prototype,Dojo 等其中一两个javascript框架并不简单,它提高你的web交互和用户体验,从而能使你的web前端有非一样的感觉,如海阔凭鱼跃。当然&…
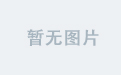
Vue开发入门看这篇文章就够了
摘要: 很多值得了解的细节。 原文:Vue开发看这篇文章就够了作者:RandomFundebug经授权转载,版权归原作者所有。 介绍 Vue 中文网Vue githubVue.js 是一套构建用户界面(UI)的渐进式JavaScript框架库和框架的区别 我们所说的前端框架…
TensorRT Samples: CharRNN
关于TensorRT的介绍可以参考: http://blog.csdn.net/fengbingchun/article/details/78469551 以下是参考TensorRT 2.1.2中的sampleCharRNN.cpp文件改写的测试代码,文件(charrnn.cpp)内容如下:#include <assert.h> #include <str…
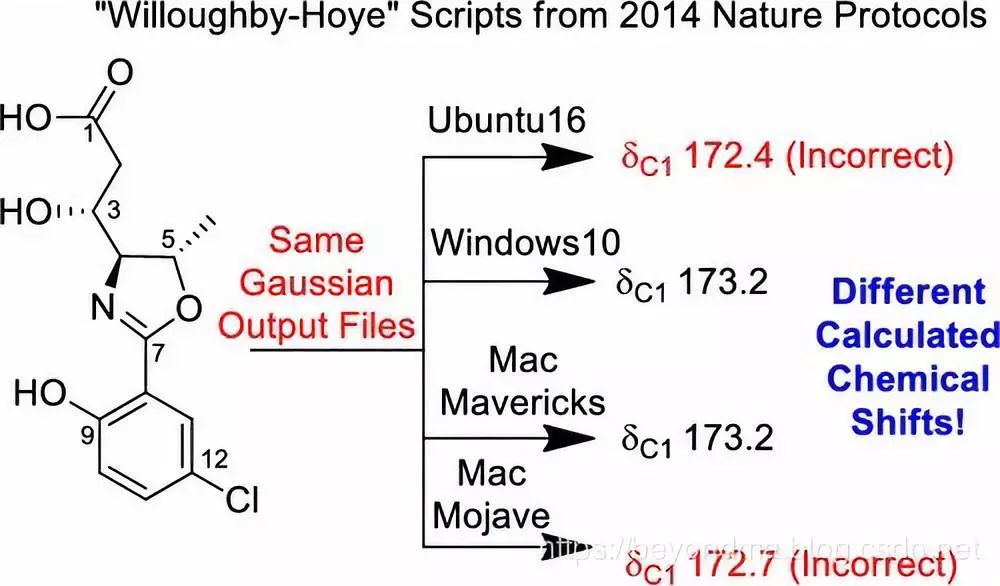
Python脚本BUG引发学界震动,影响有多大?
作者 | beyondma编辑 | Jane来源 | CSDN博客近日一篇“A guide to small-molecule structure assignment through computation of (1H and 13C) NMR chemical shifts”文章火爆网络,据作者看到的资料上看这篇论文自身的结果没有什么问题,但是,…
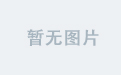
C++中public、protect和private用法区别
Calsspig : public animal,意思是外部代码可以随意访问 Classpig : protect animal ,意思是外部代码无法通过该子类访问基类中的public Classpig : private animal ,意思是告诉编译器从基类继承的每一个成员都当成private,即只有这个子类可以访问 转载于:https://blog.51cto.…
TensorRT Samples: MNIST(Plugin, add a custom layer)
关于TensorRT的介绍可以参考:http://blog.csdn.net/fengbingchun/article/details/78469551 以下是参考TensorRT 2.1.2中的samplePlugin.cpp文件改写的通过IPlugin添加一个全连接层实现对手写数字0-9识别的测试代码,plugin.cpp文件内容如下:…
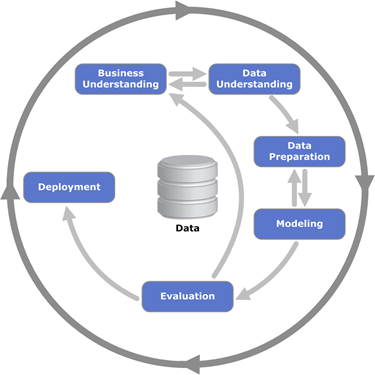
AutoML很火,过度吹捧的结果?
作者 | Denis Vorotyntsev译者 | Shawnice编辑 | Jane出品 | AI科技大本营(ID:rgznai100)【导语】现在,很多企业都很关注AutoML领域,很多开发者也开始接触和从事AutoML相关的研究与应用工作,作者也是&#…
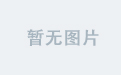
tomcat6 配置web管理端访问权限
配置tomcat 管理端登陆 /apache-tomcat-6.0.35/conf/tomcat-users.xml 配置文件,使用时需要把注释去掉<!-- <!-- <role rolename"tomcat"/> <role rolename"role1"/> <user username"tomcat" password"…
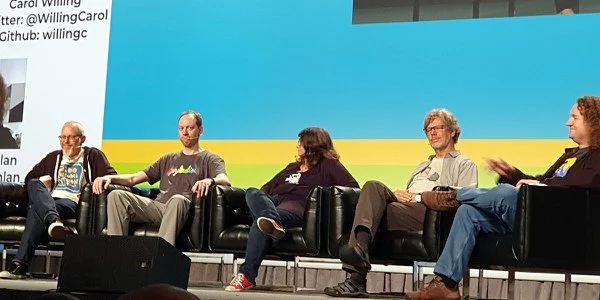
@程序员:Python 3.8正式发布,重要新功能都在这里
整理 | Jane、夕颜出品 | AI科技大本营(ID:rgznai100)【导读】最新版本的Python发布了!今年夏天,Python 3.8发布beta版本,但在2019年10月14日,第一个正式版本已准备就绪。现在,我们都…
TensorRT Samples: MNIST(serialize TensorRT model)
关于TensorRT的介绍可以参考: http://blog.csdn.net/fengbingchun/article/details/78469551 这里实现在构建阶段将TensorRT model序列化存到本地文件,然后在部署阶段直接load TensorRT model序列化的文件进行推理,mnist_infer.cpp文件内容…
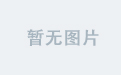
【mysql错误】用as别名 做where条件,报未知的列 1054 - Unknown column 'name111' in 'field list'...
需求:SELECT a AS b WHRER b1; //这样使用会报错,说b不存在。 因为mysql底层跑SQL语句时:where 后的筛选条件在先, as B的别名在后。所以机器看到where 后的别名是不认的,所以会报说B不存在。 这个b只是字段a查询结…
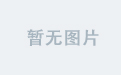
C++2年经验
网络 sql 基础算法 最多到图和树 常用的几种设计模式,5以内即可转载于:https://www.cnblogs.com/liujin2012/p/3766106.html
在Caffe中调用TensorRT提供的MNIST model
在TensorRT 2.1.2中提供了MNIST的model,这里拿来用Caffe的代码调用实现,原始的mnist_mean.binaryproto文件调整为了纯二进制文件mnist_tensorrt_mean.binary,测试结果与使用TensorRT调用(http://blog.csdn.net/fengbingchun/article/details/…
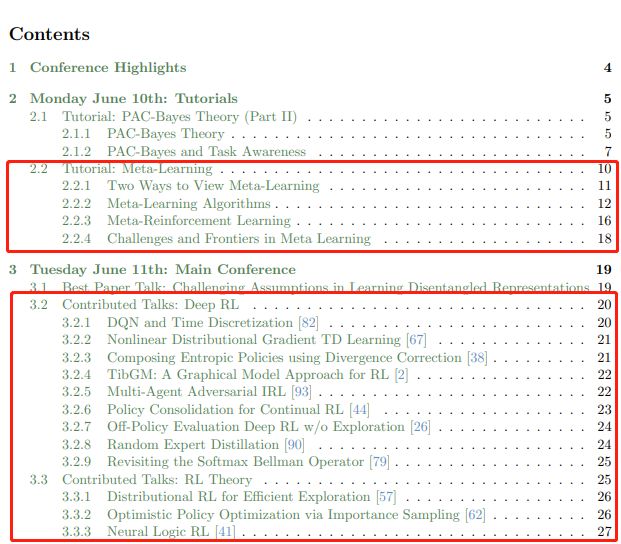
142页ICML会议强化学习笔记整理,值得细读
作者 | David Abel编辑 | DeepRL来源 | 深度强化学习实验室(ID: Deep-RL)ICML 是 International Conference on Machine Learning的缩写,即国际机器学习大会。ICML如今已发展为由国际机器学习学会(IMLS)主办的年度机器…

CF1148F - Foo Fighters
CF1148F - Foo Fighters 题意:你有n个物品,每个都有val和mask。 你要选择一个数s,如果一个物品的mask & s含有奇数个1,就把val变成-val。 求一个s使得val总和变号。 解:分步来做。发现那个奇数个1可以变成&#x…
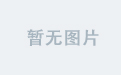
html传參中?和amp;
<a href"MealServlet?typefindbyid&mid<%m1.getMealId()%> 在这句传參中?之后的代表要传递的參数当中有两个參数第一个为type第二个为mid假设是一个參数就不用加&假设是多个參数须要加上&来传递
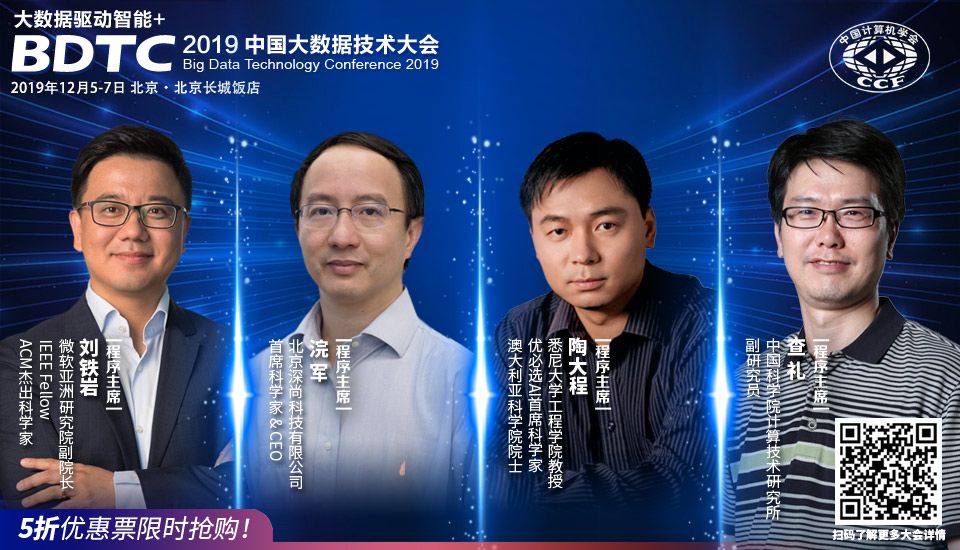
实战:手把手教你实现用语音智能控制电脑 | 附完整代码
作者 | 叶圣出品 | AI科技大本营(ID:rgznai100)导语:本篇文章将基于百度API实现对电脑的语音智能控制,不需要任何硬件上的支持,仅仅依靠一台电脑即可以实现。作者经过测试,效果不错,同时可以依据…
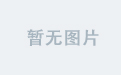
C++/C++11中左值、左值引用、右值、右值引用的使用
C的表达式要不然是右值(rvalue),要不然就是左值(lvalue)。这两个名词是从C语言继承过来的,原本是为了帮助记忆:左值可以位于赋值语句的左侧,右值则不能。 在C语言中,二者的区别就没那么简单了。一个左值表达式的求值结…