将AP-10K数据集Json格式转成Yolov8-Pose姿态的txt格式
AP-10K数据集下载地址:GitHub - AlexTheBad/AP-10K: NeurIPS 2021 Datasets and Benchmarks Track
具体代码如下:
utils.py
import glob
import os
import shutil
from pathlib import Path
import numpy as np
from PIL import ExifTags
from tqdm import tqdm
# Parameters
img_formats = ['bmp', 'jpg', 'jpeg', 'png', 'tif', 'tiff', 'dng'] # acceptable image suffixes
vid_formats = ['mov', 'avi', 'mp4', 'mpg', 'mpeg', 'm4v', 'wmv', 'mkv'] # acceptable video suffixes
# Get orientation exif tag
for orientation in ExifTags.TAGS.keys():
if ExifTags.TAGS[orientation] == 'Orientation':
break
def exif_size(img):
# Returns exif-corrected PIL size
s = img.size # (width, height)
try:
rotation = dict(img._getexif().items())[orientation]
if rotation in [6, 8]: # rotation 270
s = (s[1], s[0])
except:
pass
return s
def split_rows_simple(file='../data/sm4/out.txt'): # from utils import *; split_rows_simple()
# splits one textfile into 3 smaller ones based upon train, test, val ratios
with open(file) as f:
lines = f.readlines()
s = Path(file).suffix
lines = sorted(list(filter(lambda x: len(x) > 0, lines)))
i, j, k = split_indices(lines, train=0.9, test=0.1, validate=0.0)
for k, v in {'train': i, 'test': j, 'val': k}.items(): # key, value pairs
if v.any():
new_file = file.replace(s, f'_{k}{s}')
with open(new_file, 'w') as f:
f.writelines([lines[i] for i in v])
def split_files(out_path, file_name, prefix_path=''): # split training data
file_name = list(filter(lambda x: len(x) > 0, file_name))
file_name = sorted(file_name)
i, j, k = split_indices(file_name, train=0.9, test=0.1, validate=0.0)
datasets = {'train': i, 'test': j, 'val': k}
for key, item in datasets.items():
if item.any():
with open(f'{out_path}_{key}.txt', 'a') as file:
for i in item:
file.write('%s%s\n' % (prefix_path, file_name[i]))
def split_indices(x, train=0.9, test=0.1, validate=0.0, shuffle=True): # split training data
n = len(x)
v = np.arange(n)
if shuffle:
np.random.shuffle(v)
i = round(n * train) # train
j = round(n * test) + i # test
k = round(n * validate) + j # validate
return v[:i], v[i:j], v[j:k] # return indices
def make_dirs(dir='new_dir/'):
# Create folders
dir = Path(dir)
if dir.exists():
shutil.rmtree(dir) # delete dir
for p in dir, dir / 'labels', dir / 'images':
p.mkdir(parents=True, exist_ok=True) # make dir
return dir
def write_data_data(fname='data.data', nc=80):
# write darknet *.data file
lines = ['classes = %g\n' % nc,
'train =../out/data_train.txt\n',
'valid =../out/data_test.txt\n',
'names =../out/data.names\n',
'backup = backup/\n',
'eval = coco\n']
with open(fname, 'a') as f:
f.writelines(lines)
def image_folder2file(folder='images/'): # from utils import *; image_folder2file()
# write a txt file listing all imaged in folder
s = glob.glob(f'{folder}*.*')
with open(f'{folder[:-1]}.txt', 'w') as file:
for l in s:
file.write(l + '\n') # write image list
def add_coco_background(path='../data/sm4/', n=1000): # from utils import *; add_coco_background()
# add coco background to sm4 in outb.txt
p = f'{path}background'
if os.path.exists(p):
shutil.rmtree(p) # delete output folder
os.makedirs(p) # make new output folder
# copy images
for image in glob.glob('../coco/images/train2014/*.*')[:n]:
os.system(f'cp {image} {p}')
# add to outb.txt and make train, test.txt files
f = f'{path}out.txt'
fb = f'{path}outb.txt'
os.system(f'cp {f} {fb}')
with open(fb, 'a') as file:
file.writelines(i + '\n' for i in glob.glob(f'{p}/*.*'))
split_rows_simple(file=fb)
def create_single_class_dataset(path='../data/sm3'): # from utils import *; create_single_class_dataset('../data/sm3/')
# creates a single-class version of an existing dataset
os.system(f'mkdir {path}_1cls')
def flatten_recursive_folders(path='../../Downloads/data/sm4/'): # from utils import *; flatten_recursive_folders()
# flattens nested folders in path/images and path/JSON into single folders
idir, jdir = f'{path}images/', f'{path}json/'
nidir, njdir = Path(f'{path}images_flat/'), Path(f'{path}json_flat/')
n = 0
# Create output folders
for p in [nidir, njdir]:
if os.path.exists(p):
shutil.rmtree(p) # delete output folder
os.makedirs(p) # make new output folder
for parent, dirs, files in os.walk(idir):
for f in tqdm(files, desc=parent):
f = Path(f)
stem, suffix = f.stem, f.suffix
if suffix.lower()[1:] in img_formats:
n += 1
stem_new = '%g_' % n + stem
image_new = nidir / (stem_new + suffix) # converts all formats to *.jpg
json_new = njdir / f'{stem_new}.json'
image = parent / f
json = Path(parent.replace('images', 'json')) / str(f).replace(suffix, '.json')
os.system("cp '%s' '%s'" % (json, json_new))
os.system("cp '%s' '%s'" % (image, image_new))
# cv2.imwrite(str(image_new), cv2.imread(str(image)))
print('Flattening complete: %g jsons and images' % n)
def coco91_to_coco80_class(): # converts 80-index (val2014) to 91-index (paper)
# https://tech.amikelive.com/node-718/what-object-categories-labels-are-in-coco-dataset/
x = [0, 1, 2, 3, 4, 5, 6, 7, 8, 9, 10, None, 11, 12, 13, 14, 15, 16, 17, 18, 19, 20, 21, 22, 23, None, 24, 25, None,
None, 26, 27, 28, 29, 30, 31, 32, 33, 34, 35, 36, 37, 38, 39, None, 40, 41, 42, 43, 44, 45, 46, 47, 48, 49, 50,
51, 52, 53, 54, 55, 56, 57, 58, 59, None, 60, None, None, 61, None, 62, 63, 64, 65, 66, 67, 68, 69, 70, 71, 72,
None, 73, 74, 75, 76, 77, 78, 79, None]
return x
AP10Kjson2v8txt.py
import json
from collections import defaultdict
from utils import *
def convert_coco_json(cocojsonpath, savepath,use_keypoints=False):
"""Converts COCO dataset annotations to a format suitable for training YOLOv5 models.
Args:
labels_dir (str, optional): Path to directory containing COCO dataset annotation files.
use_segments (bool, optional): Whether to include segmentation masks in the output.
use_keypoints (bool, optional): Whether to include keypoint annotations in the output.
cls91to80 (bool, optional): Whether to map 91 COCO class IDs to the corresponding 80 COCO class IDs.
Raises:
FileNotFoundError: If the labels_dir path does not exist.
Example Usage:
convert_coco(labels_dir='../coco/annotations/', use_segments=True, use_keypoints=True, cls91to80=True)
Output:
Generates output files in the specified output directory.
"""
# save_dir = make_dirs('yolo_labels') # output directory
save_dir = make_dirs(savepath) # output directory
# Import json
for json_file in sorted(Path(cocojsonpath).resolve().glob('*.json')):
fn = Path(save_dir) / 'labels' / json_file.stem.replace('instances_', '') # folder name
fn.mkdir(parents=True, exist_ok=True)
with open(json_file) as f:
data = json.load(f)
# Create image dict
images = {f'{x["id"]:d}': x for x in data['images']}
# Create image-annotations dict
imgToAnns = defaultdict(list)
for ann in data['annotations']:
imgToAnns[ann['image_id']].append(ann)
# Write labels file
for img_id, anns in tqdm(imgToAnns.items(), desc=f'Annotations {json_file}'):
img = images[f'{img_id:d}']
h, w, f = img['height'], img['width'], img['file_name']
bboxes = []
segments = []
keypoints = []
for ann in anns:
if ann['iscrowd']:
continue
# The COCO box format is [top left x, top left y, width, height]
box = np.array(ann['bbox'], dtype=np.float64)
box[:2] += box[2:] / 2 # xy top-left corner to center
box[[0, 2]] /= w # normalize x
box[[1, 3]] /= h # normalize y
if box[2] <= 0 or box[3] <= 0: # if w <= 0 and h <= 0
continue
cls = ann['category_id'] - 1 # class
print('cls---',cls)
box = [cls] + box.tolist()
if box not in bboxes:
bboxes.append(box)
if use_keypoints and ann.get('keypoints') is not None:
k = (np.array(ann['keypoints']).reshape(-1, 3) / np.array([w, h, 1])).reshape(-1).tolist()
k = box + k
keypoints.append(k)
# Write
with open((fn / f).with_suffix('.txt'), 'a') as file:
for i in range(len(bboxes)):
if use_keypoints:
line = *(keypoints[i]), # cls, box, keypoints
file.write(('%g ' * len(line)).rstrip() % line + '\n')
if __name__ == '__main__':
source = 'COCO'
jsonpath = r'H:\XRW_Project\ap-10k\annotations'
savepath = r'H:\XRW_Project\ap10kposedata'
if source == 'COCO':
convert_coco_json(jsonpath, # directory with *.json
savepath,
use_keypoints=True)
- cocojsonpath:CoCo数据集json文件存放路径
- savepath:生成的txt存放路径
运行Ap10Kjson2v8txt.py
运行完成后得到:
txt内容:
<class-index> <x> <y> <width> <height> <px1> <py1> <p1-visibility> <px2> <py2> <p2-visibility> <pxn> <pyn> <p2-visibility>
<class-index>是对象的类的索引,<x> <y> <width> <height>是边界框的坐标,<px1> <py1> <px2> <py2> ... <pxn> <pyn>是关键点的像素坐标。坐标由空格分隔。
检查生成的txt是否准确
将txt的信息可视化在图片上进行验证
AP0KPoseVisual.py
import cv2
imgpath = r'H:\XRW_Project\ap-10k\data\000000018871.jpg'
txtpath = r'H:\XRW_Project\posedata\labels\ap10k-train-split1\000000018871.txt'
f = open(txtpath,'r')
lines = f.readlines()
img = cv2.imread(imgpath)
h, w, c = img.shape
colors = [[255, 128, 0], [255, 153, 51], [255, 178, 102], [230, 230, 0], [255, 153, 255],
[153, 204, 255], [255, 102, 255], [255, 51, 255], [102, 178, 255], [51, 153, 255],
[255, 153, 153], [255, 102, 102], [255, 51, 51], [153, 255, 153], [102, 255, 102],
[51, 255, 51], [0, 255, 0], [0, 0, 255], [255, 0, 0], [255, 255, 255]]
for line in lines:
print(line)
l = line.split(' ')
print(len(l))
cx = float(l[1]) * w
cy = float(l[2]) * h
weight = float(l[3]) * w
height = float(l[4]) * h
xmin = cx - weight/2
ymin = cy - height/2
xmax = cx + weight/2
ymax = cy + height/2
print((xmin,ymin),(xmax,ymax))
cv2.rectangle(img,(int(xmin),int(ymin)),(int(xmax),int(ymax)),(0,255,0),2)
kpts = []
for i in range(17):
x = float(l[5:][3*i]) * w
y = float(l[5:][3*i+1]) * h
s = int(l[5:][3*i+2])
print(x,y,s)
if s != 0:
cv2.circle(img,(int(x),int(y)),1,colors[i],2)
kpts.append([int(x),int(y),int(s)])
print(kpts)
kpt_line = [[1, 2], [1, 3], [2, 3], [3, 4], [4, 5],
[4, 6], [6, 7], [7, 8], [4, 9], [9, 10],
[10, 11], [5, 12], [12, 13], [13, 14],
[5, 15], [15, 16], [16, 17]]
for j in range(len(kpt_line)):
m,n = kpt_line[j][0],kpt_line[j][1]
if kpts[m-1][2] !=0 and kpts[n-1][2] !=0:
cv2.line(img,(kpts[m-1][0],kpts[m-1][1]),(kpts[n-1][0],kpts[n-1][1]),colors[j],2)
img = cv2.resize(img, None, fx=0.8, fy=0.8)
cv2.imshow('1',img)
cv2.waitKey(0)
这样就将AP-10K数据集Json格式转成Yolov8-Pose的txt格式了。
相关文章:
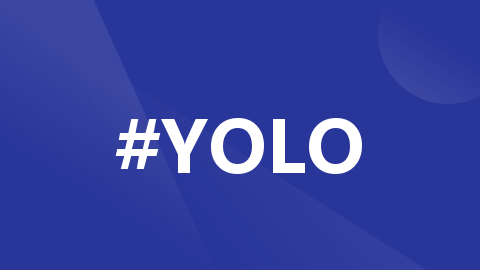
Yolov11-detect训练自己的数据集
至此,整个YOLOv11的训练预测阶段完成,与YOLOv8差不多。欢迎各位批评指正。
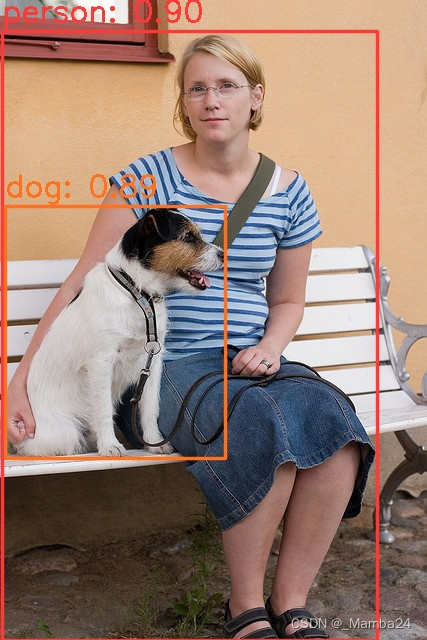
YOLOv10训练自己的数据集
至此,整个YOLOv10的训练预测阶段完成,与YOLOv8差不多。欢迎各位批评指正。
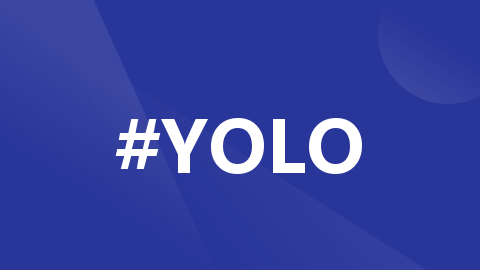
YOLOv10环境搭建、模型预测和ONNX推理
运行后会在文件yolov10s.pt存放路径下生成一个的yolov10s.onnxONNX模型文件。安装完成之后,我们简单执行下推理命令测试下效果,默认读取。终端,进入base环境,创建新环境。(1)onnx模型转换。
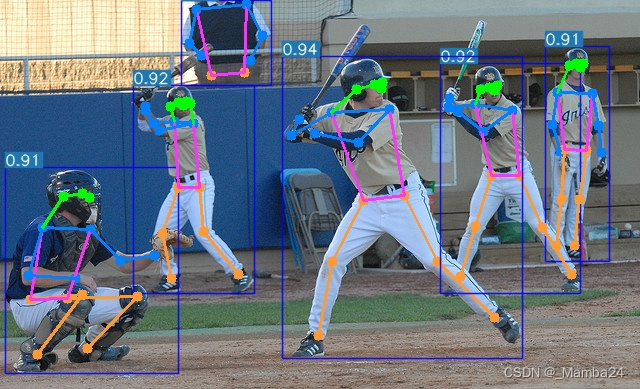
YOLOv7-Pose 姿态估计-环境搭建和推理
终端,进入base环境,创建新环境,我这里创建的是p38t17(python3.8,pytorch1.7)安装pytorch:(网络环境比较差时,耗时会比较长)下载好后打开yolov7-pose源码包。imgpath:需要预测的图片的存放路径。modelpath:模型的存放路径。Yolov7-pose权重下载。打开工程后,进入设置。
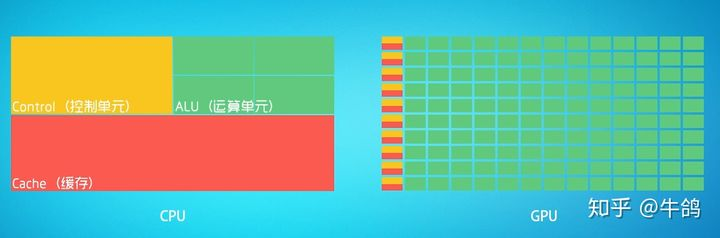
深度学习硬件基础:CPU与GPU
CPU:叫做中央处理器(central processing unit)作为计算机系统的运算和控制核心,是信息处理、程序运行的最终执行单元。[^3]可以形象的理解为有25%的ALU(运算单元)、有25%的Control(控制单元)、50%的Cache(缓存单元)GPU:叫做图形处理器。
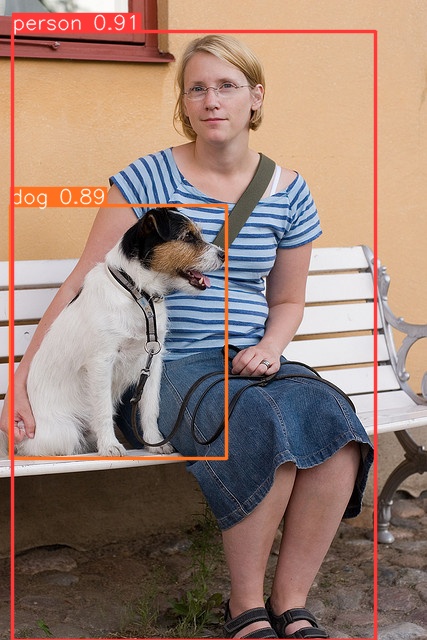
YOLOv8-Detect训练CoCo数据集+自己的数据集
至此,整个训练预测阶段完成。此过程同样可以在linux系统上进行,在数据准备过程中需要仔细,保证最后得到的数据准确,最好是用显卡进行训练。有问题评论区见!
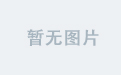
YOLOv5中Ghostbottleneck结构shortcut=True和shortcut=False有什么区别
GhostBotleneck结构中的shodcut=True和shorcut=False的区别在干是否使用残差连接。当shorcu=True时,使用残差连接,可以以加速模型的收敛速度和提高模型的准确率,当shorcu=False时,不使用残差连接,可以减少模型的参数数量和计算量。实际上不只是Ghostbottleneck具有残差连接,在C3、C2f等具有Bottleneck模块的结构均可根据此例举一反三。残差块是深度卷积神经网络中的一种基本模块,可以有效地解决梯度消失和梯度爆炸的问题。
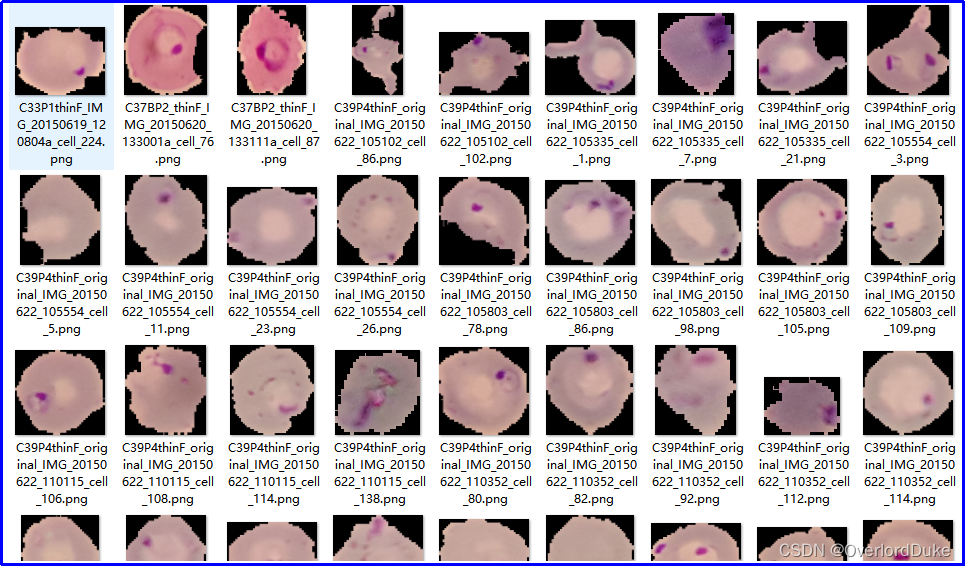
基于深度学习的细胞感染性识别与判定
通过引入深度学习技术,我们能够更精准地识别细胞是否受到感染,为医生提供更及时的信息,有助于制定更有效的治疗方案。基于深度学习的方法通过学习大量样本,能够自动提取特征并进行准确的感染性判定,为医学研究提供了更高效和可靠的手段。通过引入先进的深度学习技术,我们能够实现更快速、准确的感染性判定,为医学研究和临床实践提供更为可靠的工具。其准确性和效率将为医学研究带来新的突破,为疾病的早期诊断和治疗提供更可靠的支持。通过大规模的训练,模型能够学到细胞感染的特征,并在未知数据上做出准确的预测。
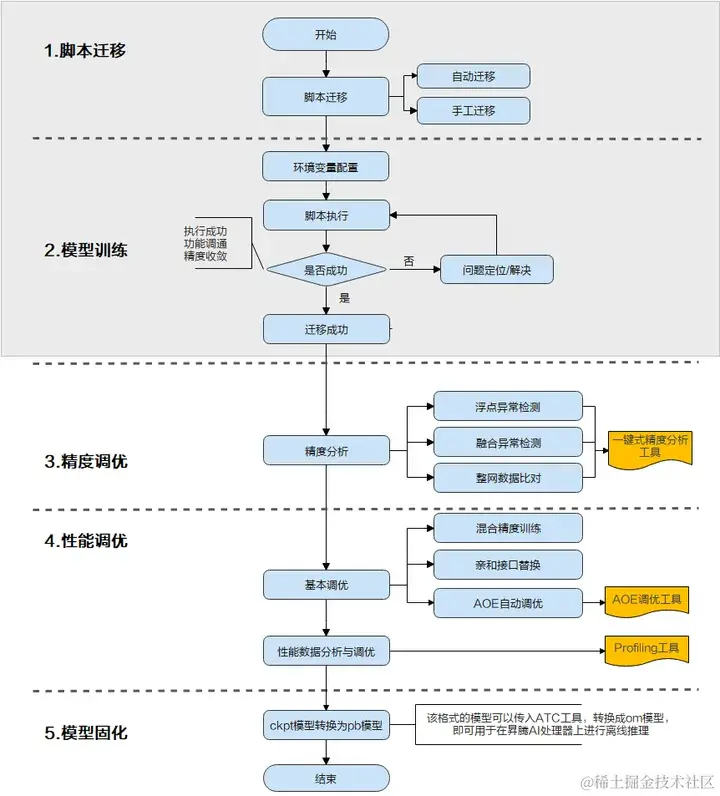
一文详解TensorFlow模型迁移及模型训练实操步骤
当前业界很多训练脚本是基于TensorFlow的Python API进行开发的,默认运行在CPU/GPU/TPU上,为了使这些脚本能够利用昇腾AI处理器的强大算力执行训练,需要对TensorFlow的训练脚本进行迁移。
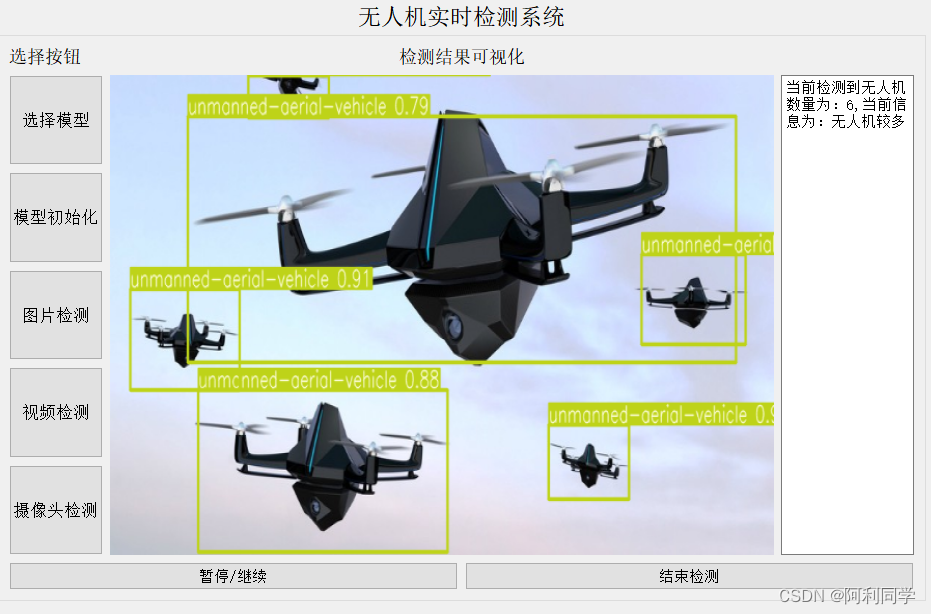
改进的yolov5目标检测-yolov5替换骨干网络-yolo剪枝(TensorRT及NCNN部署)
改进的yolov5目标检测-yolov5替换骨干网络-yolo剪枝(TensorRT及NCNN部署)2021.10.30 复现TPH-YOLOv52021.10.31 完成替换backbone为Ghostnet2021.11.02 完成替换backbone为Shufflenetv22021.11.05 完成替换backbone为Mobilenetv3Small2021.11.10 完成EagleEye对YOLOv5系列剪枝支持2021.11.14 完成MQBench对YOLOv5系列量
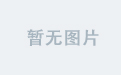
PyTorch中nn.Module的继承类中方法foward是自动执行的么?
在 PyTorch的 nn.Module中,forward方法并不是自动执行的,但它是在模型进行前向传播时必须调用的一个方法。当你实例化一个继承自torch.nn.Module的自定义类并传入输入数据时,需要通过调用该实例来实现前向传播计算,这实际上会隐式地调用forward方法。
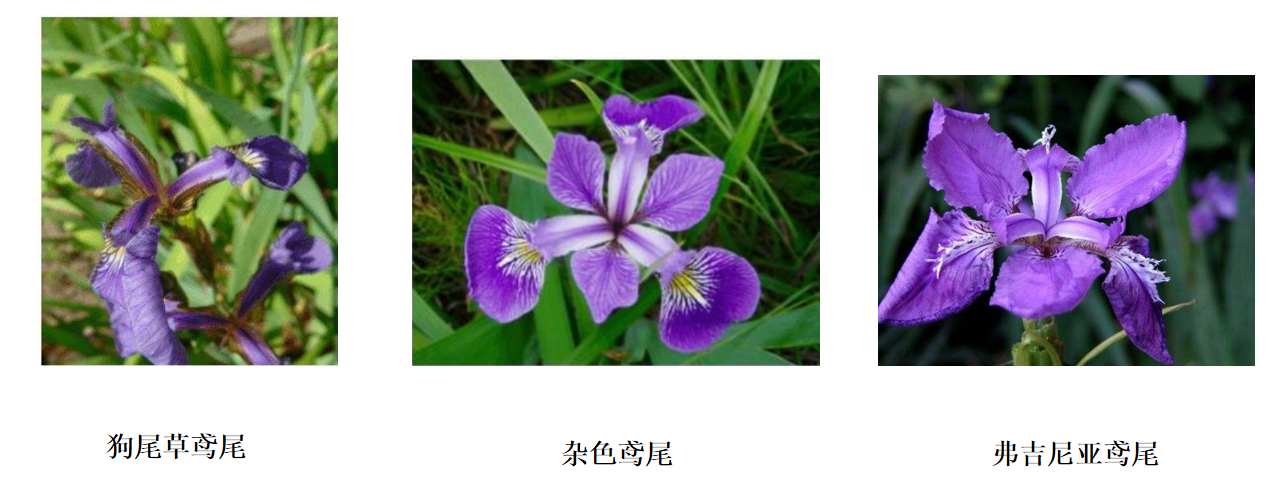
基于神经网络——鸢尾花识别(Iris)
鸢尾花识别是学习AI入门的案例,这里和大家分享下使用Tensorflow2框架,编写程序,获取鸢尾花数据,搭建神经网络,最后训练和识别鸢尾花。
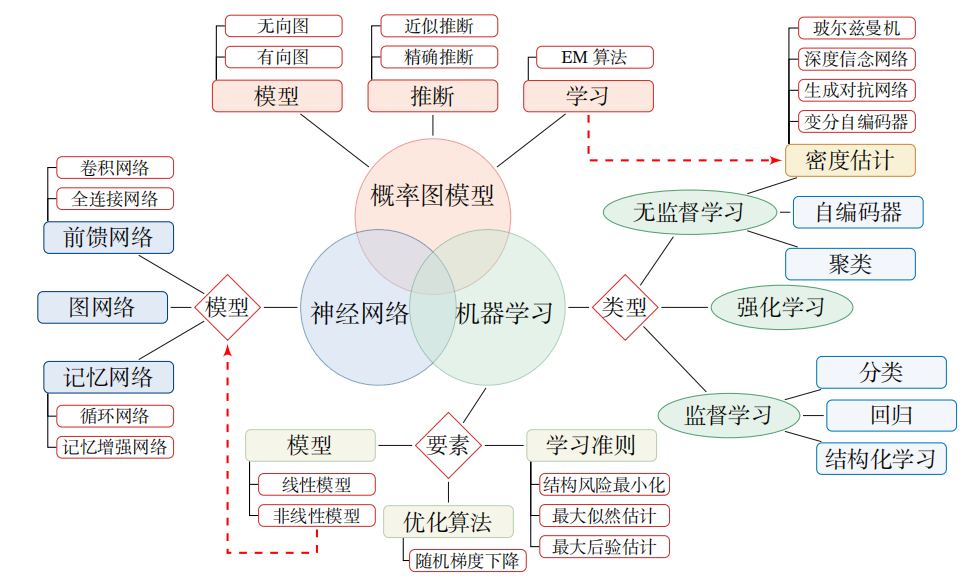
深度学习知识点全面总结
深度学习定义:一般是指通过训练多层网络结构对未知数据进行分类或回归深度学习分类:有监督学习方法——深度前馈网络、卷积神经网络、循环神经网络等; 无监督学习方法——深度信念网、深度玻尔兹曼机,深度自编码器等。深度神经网络的基本思想是通过构建多层网络,对目标进行多层表示,以期通过多层的高层次特征来表示数据的抽象语义信息,获得更好的特征鲁棒性。神经网络的计算主要有两种:前向传播(foward propagation, FP)作用于每一层的输入,通过逐层计算得到输出结果;
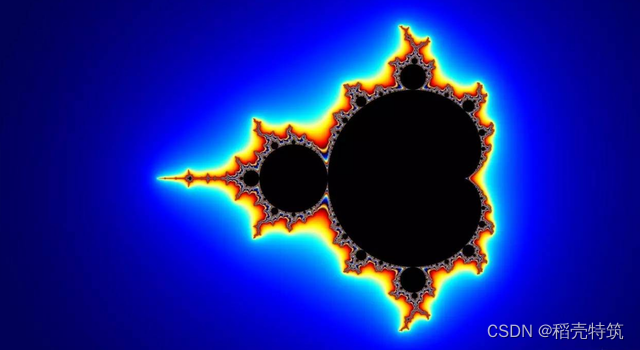
为什么深度学习神经网络可以学习任何东西
尽管如此,神经网络在处理一些对计算机而言极具挑战性的任务上表现出色,特别是在需要直觉和模糊逻辑的领域,如计算机视觉和自然语言处理,神经网络已经彻底改变了这些领域的面貌。在探讨神经网络如何学习的过程中,我们首先遇到了一个基本问题:如果我们不完全知道一个函数的形式,只知道它的部分输入和输出值,我们能否对这个函数进行逆向工程?重要的是,只要知道了这个函数,就可以针对任意输入x计算出对应的输出y。一种简单而有力的思考世界的方式,通过结合简单的计算,我们可以让计算机构造任何我们想要的功能,神经网络,从本质上讲,
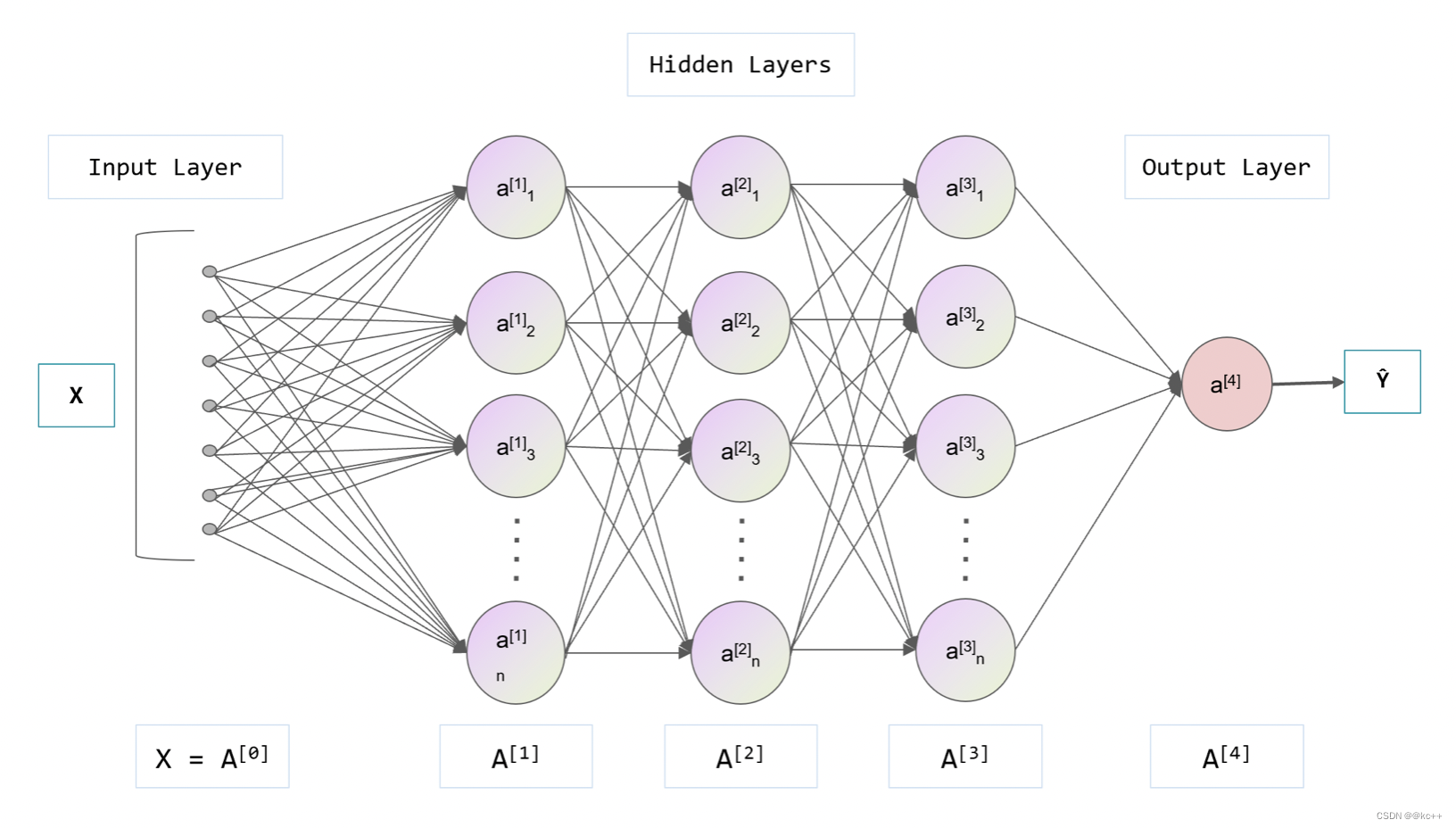
深度学习与神经网络
神经网络是一种模拟人脑神经元行为的计算模型,神经网络由大量的神经元(在计算领域中常被称为“节点”或“单元”)组成,并且这些神经元被分为不同的层,分别为输入层、隐藏层和输出层。每一个神经元都与前一层的所有神经元相连接,连接的强度(或权重)代表了该连接的重要性。神经元接收前一层神经元的信息(这些信息经过权重加权),然后通过激活函数(如Sigmoid、ReLU等)处理,将结果传递到下一层。输入层接收原始数据,隐藏层负责处理这些数据,而输出层则将处理后的结果输出。
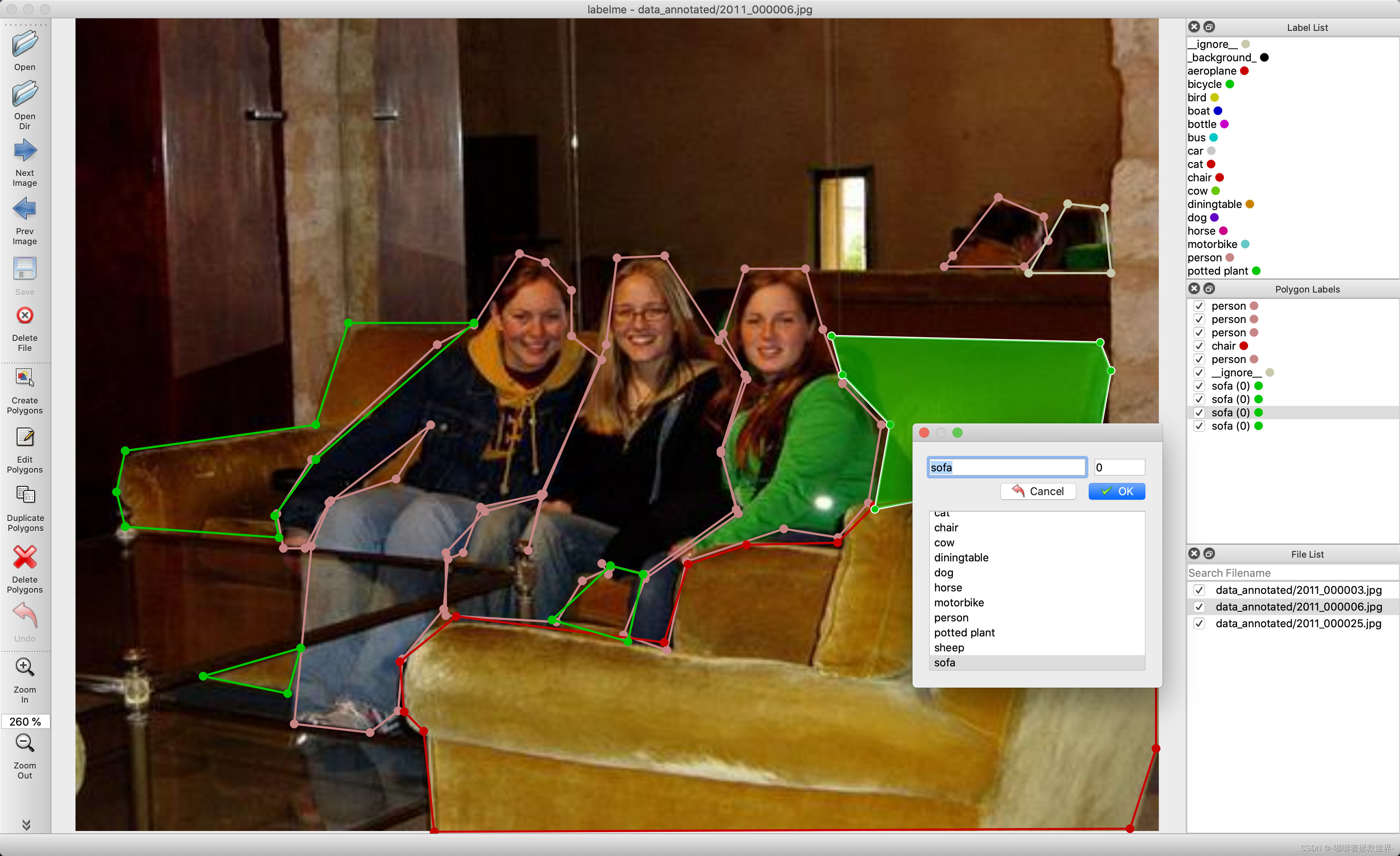
labelme安装与使用教程(内附一键运行包和转格式代码)
Labelme是一个开源的图像标注工具,由麻省理工学院的计算机科学和人工智能实验室(CSAIL)开发。它主要用于创建计算机视觉和机器学习应用所需的标记数据集。LabelMe让用户可以在图片上标注对象和区域,为机器学习模型提供训练数据。它支持多种标注类型,如矩形框、多边形和线条等。它是用 Python 编写的,并使用 Qt 作为其图形界面。
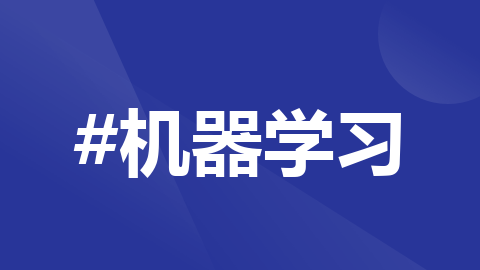
讲解光流估计 liteflownet3
LiteFlowNet3 是光流估计模型 LiteFlowNet 的最新版本。它采用了轻量级的网络结构,具有较小的模型参数和计算复杂度,同时具备较高的计算效率和准确性。LiteFlowNet3 的设计目标是在保持较小模型尺寸的同时,提供与传统光流估计算法相当甚至更好的性能。
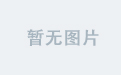
讲解异常: cv::Exception,位于内存位置 0x00000059E67CE590 处
在使用OpenCV进行图像处理和计算机视觉任务时,异常是一种常见的异常情况,通常由于内存分配失败引起。在解决该异常时,我们应该考虑增加系统可用内存、优化算法和数据集,以及检查代码中的内存管理问题。通过这些方法,我们可以更好地处理异常,提高系统的稳定性和性能。希望本文能够帮助您理解和解决异常,并顺利进行OpenCV图像处理和计算机视觉任务。
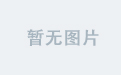
讲解Unsupported gpu architecture ‘compute_*‘2017解决方法
在使用2017年以前的NVIDIA GPU进行深度学习训练时,经常会遇到"Unsupported GPU Architecture 'compute_*'"的错误。本篇文章将介绍该错误的原因并提供解决方法。
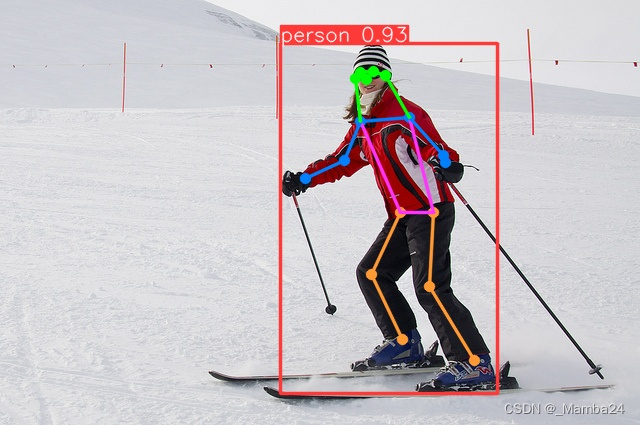
YOLOv8-Pose训练自己的数据集
至此,整个YOLOv8-Pose模型训练预测阶段完成。此过程同样可以在linux系统上进行,在数据准备过程中需要仔细,保证最后得到的数据准确,最好是用显卡进行训练。有问题评论区见!
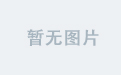
解决方案:avcodec_receive_packet AVERROR(EAGAIN)
FFmpeg是一个开源的跨平台音视频处理工具集,它由一个主命令行工具和一组库组成,提供了音视频编解码、格式转换、流媒体处理、音视频过滤、音视频录制和播放等功能。错误,我们将继续循环,直到获取到一个有效的数据包或遇到其他错误。同时,根据实际情况,调整解码器的缓冲区大小也可能有助于提高解码性能和减少错误发生的频率。然后,我们获取音频解码器并创建解码器上下文,并进行解码器的初始化。错误,并在实际应用场景中对解码后的音频数据包进行处理和分析。你可以根据自己的需求,进一步扩展和定制代码。,如果是的话,我们继续循环。
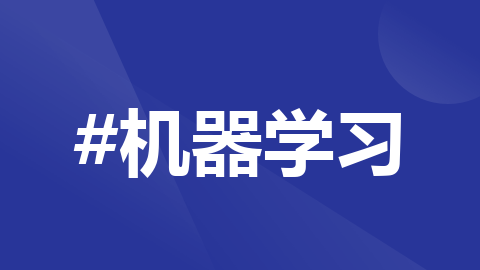
讲解from .pycaffe import Net, SGDSolver, NesterovSolver, AdaGradSolver, RMSPropSolver, AdaDeltaSolver,
相比于AdaGrad,AdaDelta算法进一步减少了学习率震荡的问题,并提供了更平稳的优化过程。以上六个模块在Caffe中发挥着重要的作用,为深度学习模型的训练和优化提供了基础支持。通过合理选择和配置这些模块,我们可以根据具体任务和模型需求进行高效的训练和推理。它是一种自适应学习率方法,通过使用梯度平方的滑动平均值来调整每个参数的学习率。如果你对Caffe框架、深度学习模型训练有进一步的兴趣,建议你阅读Caffe的官方文档和资源,深入学习和探索。模块,我们可以创建和操控神经网络,从而进行模型训练和推理。
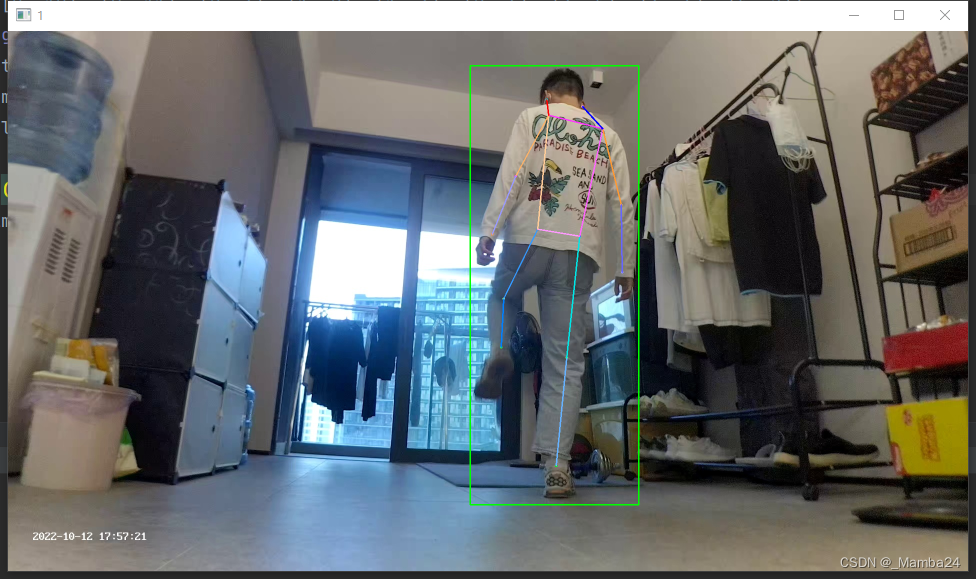
将labelme标注的人体姿态Json文件转成训练Yolov8-Pose的txt格式
最近在训练Yolov8-Pose时遇到一个问题,就是如何将自己使用labelme标注的Json文件转化成可用于Yolov8-Pose训练的txt文件。
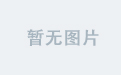
讲解Attempting to deserialize object on a CUDA device but torch.cuda.is_available() is False
"Attempting to deserialize object on a CUDA device but torch.cuda.is_available() is False" 错误提示表明您的代码尝试将一个在 CUDA 设备上训练好的模型加载到不支持 CUDA 的设备上,或者是将其加载到 CPU 上。在 PyTorch 中,当您试图将一个已经在 CUDA 设备上训练好的模型加载到 CPU 上时,或者当尝试将一个在 CUDA 设备上训练好的模型加载到不支持 CUDA 的设备上时,就会出现这个错误。
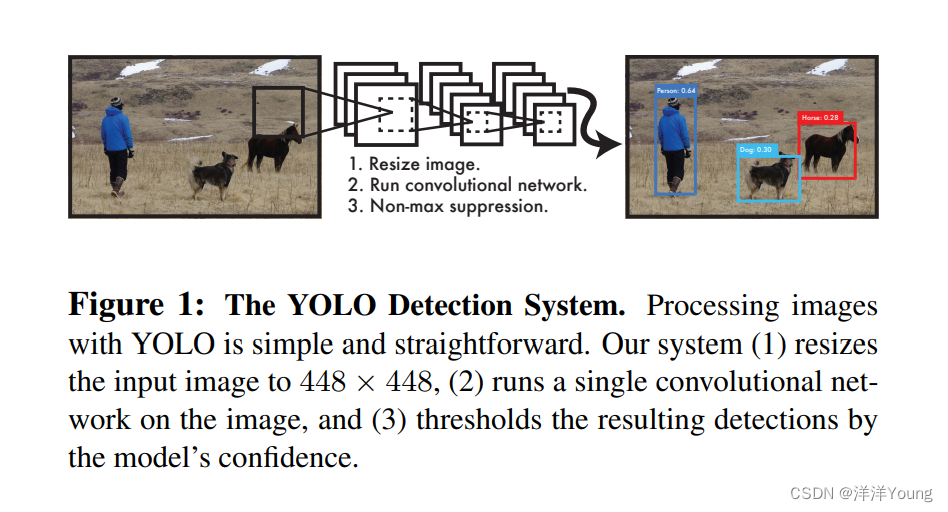
【卷积神经网络】YOLO 算法原理
文章浏览阅读128次。在计算机视觉领域中,目标检测(Object Detection)是一个具有挑战性且重要的新兴研究方向。目标检测不仅要预测图片中是否包含待检测的目标,还需要在图片中指出它们的位置。2015年,Joseph Redmon, Santosh Divvala 等人提出第一个 YOLO 模型,该模型具有实时性高、支持多物体检测的特点,已成为目标检测领域热门的研究算法。本文主要介绍 YOLO 算法及其基本原理。
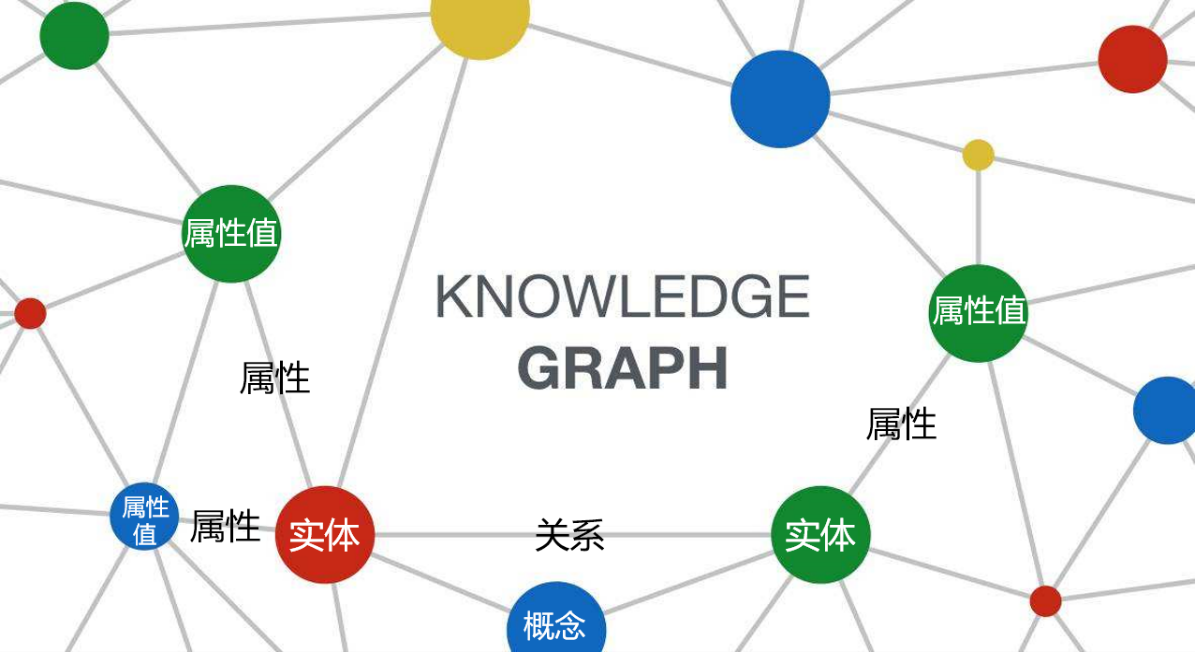
解码知识图谱:从核心概念到技术实战
本文深入探索了知识图谱的核心概念、发展历程、研究内容以及其在表示、存储、获取、构建和推理方面的技术细节。结合Python和PyTorch示例代码,文章旨在为读者提供一个全面、深入且实用的知识图谱概览,帮助广大技术爱好者和研究者深化对此领域的认识。
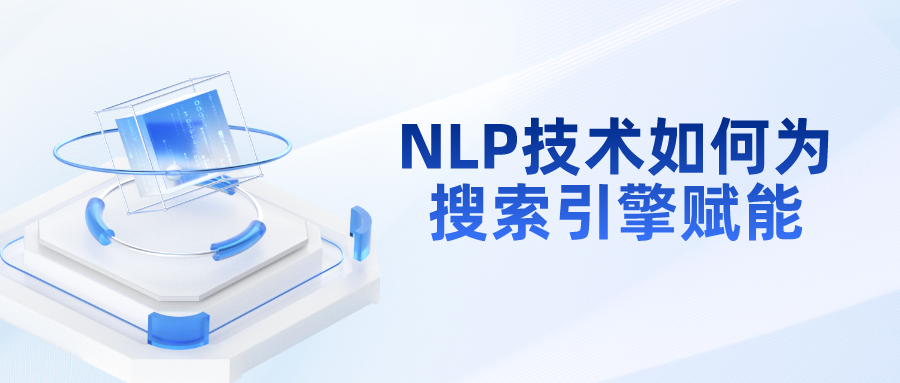
NLP技术如何为搜索引擎赋能
在全球化时代,搜索引擎不仅需要为用户提供准确的信息,还需理解多种语言和方言。本文详细探讨了搜索引擎如何通过NLP技术处理多语言和方言,确保为不同地区和文化的用户提供高质量的搜索结果,同时提供了基于PyTorch的实现示例,帮助您更深入地理解背后的技术细节。