基于 Opencv 实现眼睛控制鼠标
作者 | 小白
来源 | 小白学视觉
如何用眼睛来控制鼠标?一种基于单一前向视角的机器学习眼睛姿态估计方法。在此项目中,每次单击鼠标时,我们都会编写代码来裁剪你们的眼睛图像。使用这些数据,我们可以反向训练模型,从你们您的眼睛预测鼠标的位置。在开始项目之前,我们需要引入第三方库。
# For monitoring web camera and performing image minipulations
import cv2
# For performing array operations
import numpy as np
# For creating and removing directories
import os
import shutil
# For recognizing and performing actions on mouse presses
from pynput.mouse import Listener
首先让我们了解一下Pynput的Listener工作原理。pynput.mouse.Listener创建一个后台线程,该线程记录鼠标的移动和鼠标的点击。这是一个简化代码,当你们按下鼠标时,它会打印鼠标的坐标:
from pynput.mouse import Listener
def on_click(x, y, button, pressed):
"""Args:x: the x-coordinate of the mousey: the y-coordinate of the mousebutton: 1 or 0, depending on right-click or left-clickpressed: 1 or 0, whether the mouse was pressed or released"""
if pressed:
print (x, y)
with Listener(on_click = on_click) as listener:listener.join()
现在,为了实现我们的目的,让我们扩展这个框架。但是,我们首先需要编写裁剪眼睛边界框的代码。我们稍后将在on_click函数内部调用此函数。我们使用Haar级联对象检测来确定用户眼睛的边界框。你们可以在此处下载检测器文件,让我们做一个简单的演示来展示它是如何工作的:
import cv2
# Load the cascade classifier detection object
cascade = cv2.CascadeClassifier("haarcascade_eye.xml")
# Turn on the web camera
video_capture = cv2.VideoCapture(0)
# Read data from the web camera (get the frame)
_, frame = video_capture.read()
# Convert the image to grayscale
gray = cv2.cvtColor(frame, cv2.COLOR_BGR2GRAY)
# Predict the bounding box of the eyes
boxes = cascade.detectMultiScale(gray, 1.3, 10)
# Filter out images taken from a bad angle with errors
# We want to make sure both eyes were detected, and nothing else
if len(boxes) == 2:
eyes = []
for box in boxes:# Get the rectangle parameters for the detected eye
x, y, w, h = box# Crop the bounding box from the frame
eye = frame[y:y + h, x:x + w]# Resize the crop to 32x32
eye = cv2.resize(eye, (32, 32))# Normalize
eye = (eye - eye.min()) / (eye.max() - eye.min())# Further crop to just around the eyeball
eye = eye[10:-10, 5:-5]# Scale between [0, 255] and convert to int datatype
eye = (eye * 255).astype(np.uint8)# Add the current eye to the list of 2 eyes
eyes.append(eye)# Concatenate the two eye images into one
eyes = np.hstack(eyes)
现在,让我们使用此知识来编写用于裁剪眼睛图像的函数。首先,我们需要一个辅助函数来进行标准化:
def normalize(x):minn, maxx = x.min(), x.max()
return (x - minn) / (maxx - minn)
这是我们的眼睛裁剪功能。如果发现眼睛,它将返回图像。否则,它返回None:
def scan(image_size=(32, 32)):
_, frame = video_capture.read()
gray = cv2.cvtColor(frame, cv2.COLOR_BGR2GRAY)
boxes = cascade.detectMultiScale(gray, 1.3, 10)
if len(boxes) == 2:
eyes = []
for box in boxes:
x, y, w, h = box
eye = frame[y:y + h, x:x + w]
eye = cv2.resize(eye, image_size)
eye = normalize(eye)
eye = eye[10:-10, 5:-5]
eyes.append(eye)
return (np.hstack(eyes) * 255).astype(np.uint8)
else:
return None
现在,让我们来编写我们的自动化,该自动化将在每次按下鼠标按钮时运行。(假设我们之前已经root在代码中将变量定义为我们要存储图像的目录):
def on_click(x, y, button, pressed):
# If the action was a mouse PRESS (not a RELEASE)
if pressed:
# Crop the eyeseyes = scan()
# If the function returned None, something went wrong
if not eyes is None:
# Save the imagefilename = root + "{} {} {}.jpeg".format(x, y, button)cv2.imwrite(filename, eyes)
现在,我们可以回忆起pynput的实现Listener,并进行完整的代码实现:
import cv2
import numpy as np
import os
import shutil
from pynput.mouse import Listenerroot = input("Enter the directory to store the images: ")
if os.path.isdir(root):resp = ""
while not resp in ["Y", "N"]:resp = input("This directory already exists. If you continue, the contents of the existing directory will be deleted. If you would still like to proceed, enter [Y]. Otherwise, enter [N]: ")
if resp == "Y": shutil.rmtree(root)
else:exit()
os.mkdir(root)# Normalization helper function
def normalize(x):minn, maxx = x.min(), x.max()
return (x - minn) / (maxx - minn)# Eye cropping function
def scan(image_size=(32, 32)):_, frame = video_capture.read()gray = cv2.cvtColor(frame, cv2.COLOR_BGR2GRAY)boxes = cascade.detectMultiScale(gray, 1.3, 10)
if len(boxes) == 2:eyes = []
for box in boxes:x, y, w, h = boxeye = frame[y:y + h, x:x + w]eye = cv2.resize(eye, image_size)eye = normalize(eye)eye = eye[10:-10, 5:-5]eyes.append(eye)
return (np.hstack(eyes) * 255).astype(np.uint8)
else:
return Nonedef on_click(x, y, button, pressed):
# If the action was a mouse PRESS (not a RELEASE)
if pressed:
# Crop the eyeseyes = scan()
# If the function returned None, something went wrong
if not eyes is None:
# Save the imagefilename = root + "{} {} {}.jpeg".format(x, y, button)cv2.imwrite(filename, eyes)cascade = cv2.CascadeClassifier("haarcascade_eye.xml")
video_capture = cv2.VideoCapture(0)with Listener(on_click = on_click) as listener:listener.join()
运行此命令时,每次单击鼠标(如果两只眼睛都在视线中),它将自动裁剪网络摄像头并将图像保存到适当的目录中。图像的文件名将包含鼠标坐标信息,以及它是右击还是左击。
这是一个示例图像。在此图像中,我在分辨率为2560x1440的监视器上在坐标(385,686)上单击鼠标左键:
级联分类器非常准确,到目前为止,我尚未在自己的数据目录中看到任何错误。现在,让我们编写用于训练神经网络的代码,以给定你们的眼睛图像来预测鼠标的位置。
import numpy as np
import os
import cv2
import pyautogui
from tensorflow.keras.models import *
from tensorflow.keras.layers import *
from tensorflow.keras.optimizers import *
现在,让我们添加级联分类器:
cascade = cv2.CascadeClassifier("haarcascade_eye.xml")
video_capture = cv2.VideoCapture(0)
正常化:
def normalize(x):minn, maxx = x.min(), x.max()
return (x - minn) / (maxx - minn)
捕捉眼睛:
def scan(image_size=(32, 32)):
_, frame = video_capture.read()
gray = cv2.cvtColor(frame, cv2.COLOR_BGR2GRAY)
boxes = cascade.detectMultiScale(gray, 1.3, 10)
if len(boxes) == 2:
eyes = []
for box in boxes:
x, y, w, h = box
eye = frame[y:y + h, x:x + w]
eye = cv2.resize(eye, image_size)
eye = normalize(eye)
eye = eye[10:-10, 5:-5]
eyes.append(eye)
return (np.hstack(eyes) * 255).astype(np.uint8)
else:
return None
让我们定义显示器的尺寸。你们必须根据自己的计算机屏幕的分辨率更改以下参数:
# Note that there are actually 2560x1440 pixels on my screen
# I am simply recording one less, so that when we divide by these
# numbers, we will normalize between 0 and 1. Note that mouse
# coordinates are reported starting at (0, 0), not (1, 1)
width, height = 2559, 1439
现在,让我们加载数据(同样,假设你们已经定义了root)。我们并不在乎是单击鼠标右键还是单击鼠标左键,因为我们的目标只是预测鼠标的位置:
filepaths = os.listdir(root)
X, Y = [], []
for filepath in filepaths:
x, y, _ = filepath.split(' ')
x = float(x) / width
y = float(y) / height
X.append(cv2.imread(root + filepath))
Y.append([x, y])
X = np.array(X) / 255.0
Y = np.array(Y)
print (X.shape, Y.shape)
让我们定义我们的模型架构:
model = Sequential()
model.add(Conv2D(32, 3, 2, activation = 'relu', input_shape = (12, 44, 3)))
model.add(Conv2D(64, 2, 2, activation = 'relu'))
model.add(Flatten())
model.add(Dense(32, activation = 'relu'))
model.add(Dense(2, activation = 'sigmoid'))
model.compile(optimizer = "adam", loss = "mean_squared_error")
model.summary()
这是我们的摘要:
接下来的任务是训练模型。我们将在图像数据中添加一些噪点:
epochs = 200
for epoch in range(epochs):model.fit(X, Y, batch_size = 32)
现在让我们使用我们的模型来实时移动鼠标。请注意,这需要大量数据才能正常工作。但是,作为概念证明,你们会注意到,实际上只有200张图像,它确实将鼠标移到了你们要查看的常规区域。当然,除非你们拥有更多的数据,否则这是不可控的。
while True:eyes = scan()
if not eyes is None:eyes = np.expand_dims(eyes / 255.0, axis = 0)x, y = model.predict(eyes)[0]pyautogui.moveTo(x * width, y * height)
这是一个概念证明的例子。请注意,在进行此屏幕录像之前,我们只训练了很少的数据。这是我们的鼠标根据眼睛自动移动到终端应用程序窗口的视频。就像我说的那样,这很容易,因为数据很少。有了更多的数据,它有望稳定到足以以更高的特异性进行控制。仅用几百张图像,你们就只能将其移动到注视的整个区域内。另外,如果在整个数据收集过程中,你们在屏幕的特定区域(例如边缘)都没有拍摄任何图像,则该模型不太可能在该区域内进行预测。
往
期
回
顾
分享
7款可替代top命令的工具!
资讯
再一次输给AI,弯道急速超车
技术
真香,邮件分类准确识别垃圾邮件
技术
程序员元宵节的正确打开方式
分享
点收藏
点点赞
点在看
相关文章:
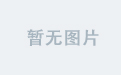
linux 安装安装rz/sz 和 ssh
安装rz,sz yum install lrzsz; 安装ssh yum install openssh-server 查看已安装包 rpm -qa | grep ssh 更新yum源 1、备份 mv /etc/yum.repos.d/CentOS-Base.repo /etc/yum.repos.d/CentOS-Base.repo.backup 2、下载新的CentOS-Base.repo 到/etc/yum.repos.d/ CentO…
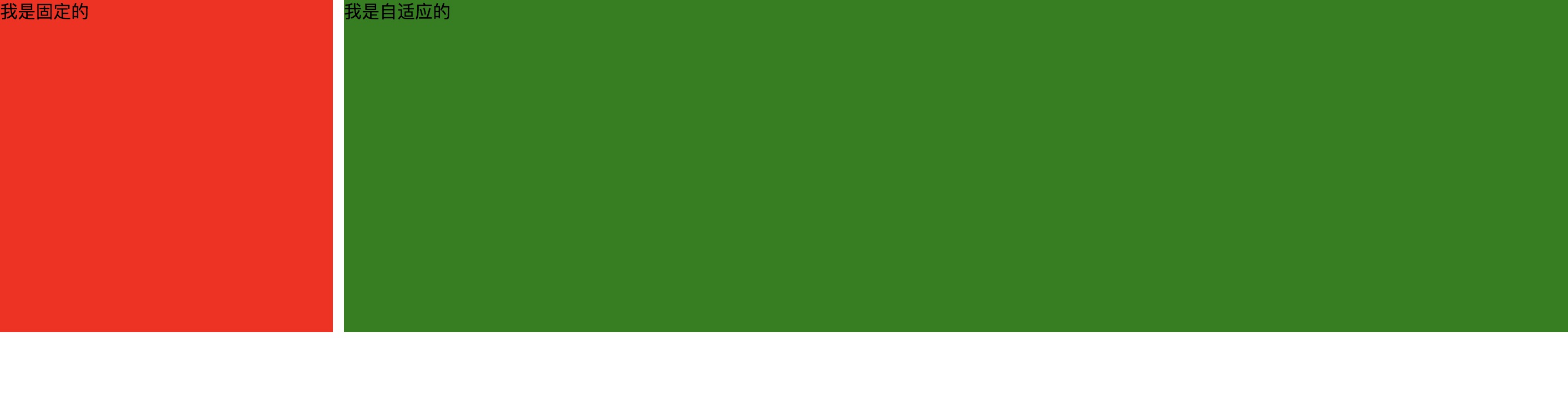
css左固定右自适应常用方法
下面是几种方法的公用部分(右自适应也是一样的,换一下方向) html: <div class"demo"> <div class"sidebar">我是固定的</div> <div class"content">我是自适应的</div> </di…

nginx或httpd实现负载均衡tomcat(三)
接博客nginx或httpd实现反向代理tomcat并实现会话保持(二)实例四:使用httpd负载均衡后端tomcat服务第一步:准备两个tomcat服务器节172.16.240.203修改tomcat的server.xml配置文件,添加一个host。<Host name"to…
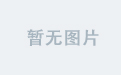
为 PHP 应用提速、提速、再提速
原文地址: http://www.ibm.com/developerworks/cn/opensource/os-php-fastapps1/ http://www.ibm.com/developerworks/cn/opensource/os-php-fastapps2/index.html为 PHP 应用提速、提速、再提速!PHP 是一种脚本语言,常用于创建 Web 应用程序…

冬奥会夺金的背后杀手锏,竟是位 AI 虚拟教练
整理 | 禾木木 出品 | AI科技大本营(ID:rgznai100) 近日,一则消息登上了热搜: 2月14日晚,在北京冬奥会自由式滑雪女子空中技巧决赛中,徐梦桃为中国代表团再添一金。她选择了难度系数4.293的动作,…
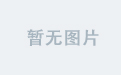
Socket-实例
import socket,os,time server socket.socket() server.bind(("localhost",9999)) server.listen()while True:conn,addrserver.accept()print("new conn",addr)while True:print("等待新指令")data conn.recv(1024)if not data:print("客…
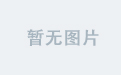
kcachegrind安装
http://kcachegrind.sourceforge.net/cgi-bin/show.cgi/KcacheGrindDownload http://hi.baidu.com/wangxinhui419/blog/item/4a7409c78c22b4c8d100608a.html http://wxiner.blog.sohu.com/156841393.html说明:linux下如果安装不上,直接下载windows版的吧…
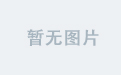
Java【小考】
课上, 老师出了一个题: 考察:1、类的定义 2、类的属性 3、类的方法、重载、构造方法、代码块 题目是这样的: 设计 一个 类:Tree 要求: 1、包含main方法 2、属性:静态: String name ; double hei…
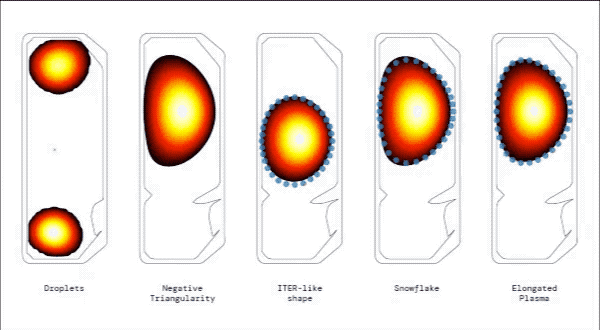
首个深度强化学习AI,能控制核聚变,成功登上《Nature》
编译 | 禾木木 出品 | AI科技大本营(ID:rgznai100) 最近,DeepMind 开发出了世界上第一个深度强化学习 AI ,可以在模拟环境和真正的核聚变装置中实现对等离子体的自主控制。 这项研究成果登上了《Nature》杂志。 托卡马克是一种用于…
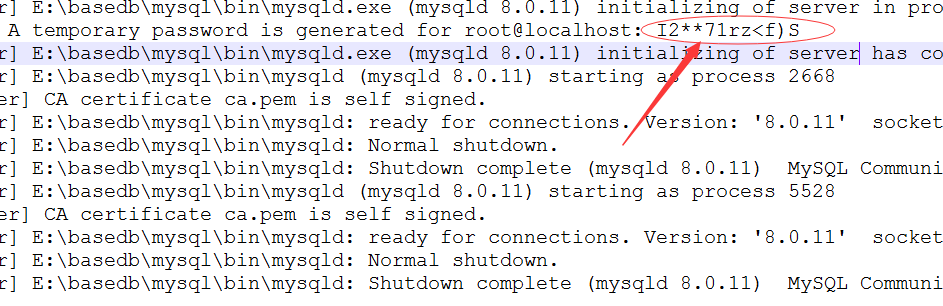
windows下安装mysql8.0压缩版
下面总结下安装过程: 首先解压下载好的压缩版本。将解压后mysql的bin文件目录配置系统环境path变量中使用cmd打开命令窗口,输入mysqld --initialize命令初始化mysql的data数据目录,记住初始化完毕后,会在解压目录下生成一个data文…
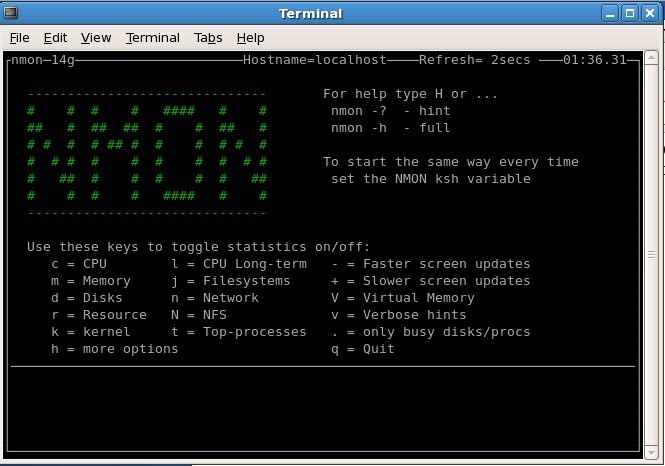
Linux实时监控工具Nmon使用
官网:http://nmon.sourceforge.net/pmwiki.php?nMain.HomePage 下载:http://sourceforge.net/projects/nmon/files/nmon_linux_14g.tar.gz 解压: #chmod ux nmon_x86_64_sles11 #chmod 777 nmon_x86_64_sles11 版本不同,对应文件…
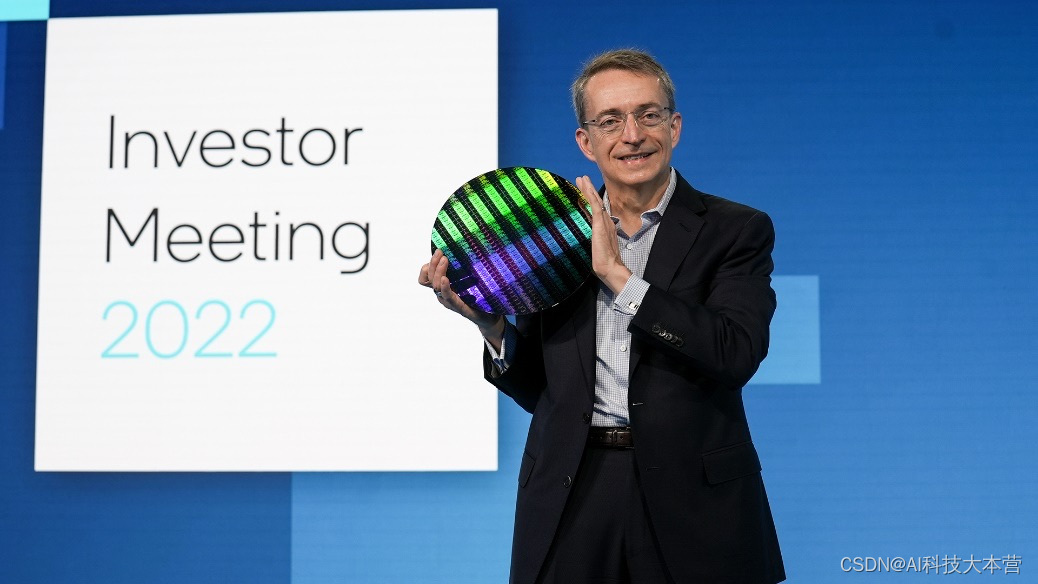
英特尔2022年投资者大会:公布技术路线图及重要节点
在英特尔2022年投资者大会上,英特尔CEO帕特基辛格和各业务部门负责人概述了公司发展战略及长期增长规划的主要内容。在半导体需求旺盛的时代,英特尔的多项长期规划将充分把握转型增长的机遇。在演讲中,英特尔公布了其主要业务部门的产品路线图…
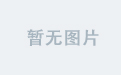
20151102adonet2
实现增删改查 ExecuteNonQuery:执行增删改语句,返回值为该命令所影响的行数. ExecuteReader:执行查询,并返回 一个DataReader对象. ExecuteScalar:执行查询,返回查询数.(删除修改时判断记录是否存在) string strSql string.Format("select count(*) from RNews where New…

day07 -文件的基本操作
1、什么是文件? 文件是操作系统为用户或应用程序提供的一个读写硬盘的虚拟单位文件的操作核心就:读、写即我们只需要对于进行读写操作,就是对操作系统发起请求,然后由操作系统将用户或者应用程序对文件的读写操作转换成具体的硬盘…
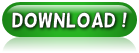
淘宝Tengine服务器开源
http://tengine.taobao.org/index_cn.htmlTengine是由 淘宝核心系统部基于Nginx开发的Web服务器,它在 Nginx的基础上,针对大访问量网站的需求,添加了很多功能和特性。Tengine的性能和稳定性已经在大型的网站如 淘宝网, 淘宝商城等…

22个案例详解Pandas数据分析/预处理时的实用技巧,超简单
作者 | 俊欣来源 | 关于数据分析与可视化今天小编打算来讲一讲数据分析方面的内容,整理和总结一下Pandas在数据预处理和数据分析方面的硬核干货,我们大致会说Pandas计算交叉列表Pandas将字符串与数值转化成时间类型Pandas将字符串转化成数值类型Pandas当…
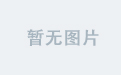
PL/SQL 中Returning Into的用法
ORACLE的DML语句中可以指定RETURNING INTO语句。RETURNING INTO语句的使用在很多情况下可以简化PL/SQL编程,少一次select into语句。DELETE操作:RETURNING返回的是DELETE之前的结果; INSERT操作:RETURNING返回的是INSERT之后的结果…

Java常用多线程辅助工具---countdownLatch
为什么80%的码农都做不了架构师?>>> 前言 上一篇博文说到semaphore,一个加强版的synchronized,该多线程辅助工具适用于控制对资源操作或者访问的场景。现在有一张场景是,需要等各个线程都都执行完了再进行下一步的操作…

听说,英特尔要对外开放 x86 授权?
整理 | 郑丽媛出品 | CSDN(ID:CSDNnews)同为主流芯片架构,相较于可申请授权的 ARM 和开源的 RISC-V,x86 一直以来都保持着“高高在上”的立场——唯有英特尔和 AMD 拥有 x86 授权。但目前看来,x86 的“高冷…
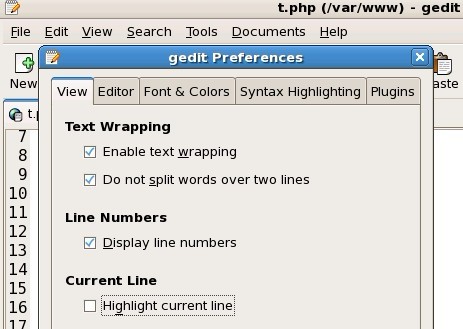
Linux下gedit显示行号
Gedit 编辑->视图
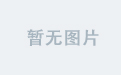
关于String数组的用法
android ContextWrapper.getResources(ContextWrapper.java:89) getResources()报错原因是在OnCreate方法外 无法完成Context的初始化 正确做法: String[] names;names new String[6];names[0] getResources().getString(R.string.a);names[1] getResources().g…
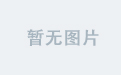
使用RabbitMQ做数据接收和处理时,自动关闭
场景:N个客户端向MQ里发送数据;服务器上有另一个控制台程序(假设叫ServerClient)来处理这里数据(往数据库保存)。方向为Client * n→MQSERVER→ServerClient 问题:ServerClient自动关闭,没有错误日志&#…

在 Python 中妙用短路机制
作者 | 费弗里来源 | Python大数据分析本期我们即将学习的是:Python中短路机制的妙用。不同于物理学中的「短路」(Short circuit)那般危险,Python中的短路机制非常有用,跟很多其他编程语言中的短路机制作用类似&#x…
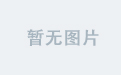
《Advanced PHP Programming》读书笔记
此书无中文版,但是写的极好!本来想翻译的,可是时间不允许了。 http://www.amazon.com/Advanced-PHP-Programming-George-Schlossnagle/dp/0672325616/refpd_rhf_dp_p_t_1约定:加粗字体表示章节,由于时间关系解释性的说…
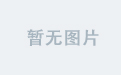
cookie记录用户的浏览商品的路径
在电子商务的网站中,经常要记录用户的浏览路径,以判断用户到底对哪些商品感兴趣,或者哪些商品之间存在关联。 下面将使用cookie记录用户的浏览过的历史页面。该网站将每个页面的标题保存在该页面的$TITLE 变量中,用户每访问一次&a…
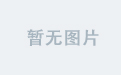
如何快速写一个违背双亲委托机制的classloader
很多情况下,不得以必须写个classloader来满足需求。例如你一个工程里你想用相同的数据库的多个版本,自己制定了一个jar包目录,没有classloader管理等等。如果是一个遵循java已经规定好的机制的classloader(双亲委托以及加载依赖类…
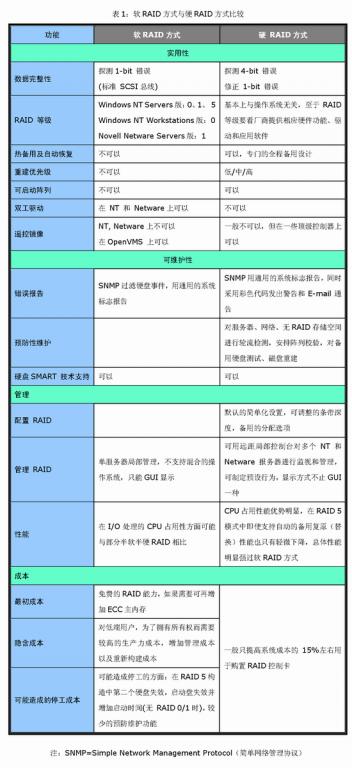
让我们谈谈RAID
转自:http://soft.zdnet.com.cn/techupdate/2004/0330/397707.shtml 更新时间: 2004-03-30 17:14:00 作者: 赵效民 感觉写的很好就转来了。 RAID的种类 RAID的英文全称为Redundant Array of Inexpensive(或Independent) Disks&…
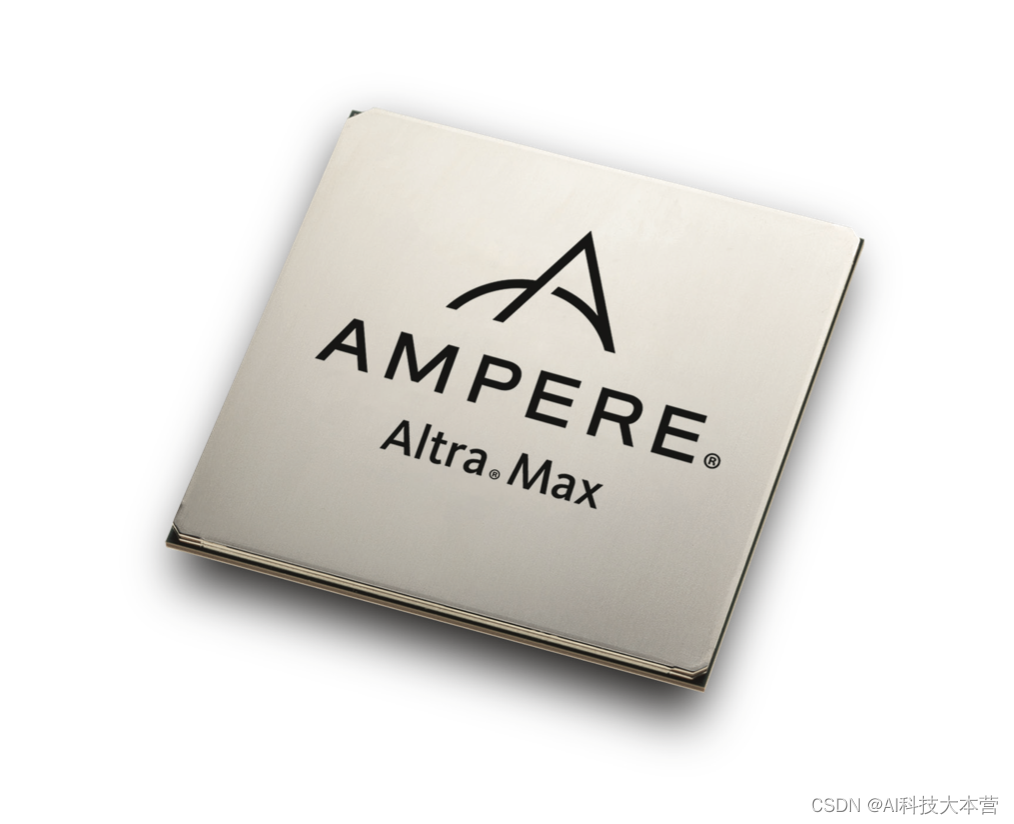
Ampere 携手 Rigetti 开发混合量子经典计算机
该合作旨在为价值 160 亿美元的机器学习市场提供服务,赋能机器学习应用的发展双方将把 Ampere Altra Max 处理器和 Rigetti 量子处理单元进行优化结合,为机器学习提供整合的云平台 2022 年 2 月 21 日,安晟培半导体科技有限公司(A…

[C++] NULL VS nullptr
NULL VS nullptr 转载于:https://www.cnblogs.com/tianhangzhang/p/4945623.html
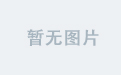
swift 的defer使得资源的分配和释放代码可以放到一起
只是一种语法和逻辑上的优化