Numpy入门教程:05. 逻辑函数
背景
什么是 NumPy 呢?
NumPy 这个词来源于两个单词 – Numerical
和Python
。其是一个功能强大的 Python 库,可以帮助程序员轻松地进行数值计算,通常应用于以下场景:
- 执行各种数学任务,如:数值积分、微分、内插、外推等。因此,当涉及到数学任务时,它形成了一种基于 Python 的 MATLAB 的快速替代。
- 计算机中的图像表示为多维数字数组。NumPy 提供了一些优秀的库函数来快速处理图像。例如,镜像图像、按特定角度旋转图像等。
- 在编写机器学习算法时,需要对矩阵进行各种数值计算。如:矩阵乘法、求逆、换位、加法等。NumPy 数组用于存储训练数据和机器学习模型的参数。
逻辑函数
真值测试
numpy.all(a, axis=None, out=None, keepdims=np._NoValue)
Test whether all array elements along a given axis evaluate to True.numpy.any(a, axis=None, out=None, keepdims=np._NoValue)
Test whether any array element along a given axis evaluates to True.
import numpy as npa = np.array([0, 4, 5])
b = np.copy(a)
print(np.all(a == b)) # True
print(np.any(a == b)) # True
b[0] = 1
print(np.all(a == b)) # False
print(np.any(a == b)) # Trueprint(np.all([1.0, np.nan])) # True
print(np.any([1.0, np.nan])) # Truea = np.eye(3)
print(np.all(a, axis=0)) # [False False False]
print(np.any(a, axis=0)) # [ True True True]
逻辑运算
numpy.logical_not(x, *args, **kwargs)
Compute the truth value of NOT x element-wise.numpy.logical_and(x1, x2, *args, **kwargs)
Compute the truth value of x1 AND x2 element-wise.numpy.logical_or(x1, x2, *args, **kwargs)
Compute the truth value of x1 OR x2 element-wise.numpy.logical_xor(x1, x2, *args, **kwargs)
Compute the truth value of x1 XOR x2, element-wise.
【例】
import numpy as npx = np.arange(5)
print(np.logical_not(3))
# False
print(np.logical_not([True, False, 0, 1]))
# [False True True False]
print(np.logical_not(x < 3))
# [False False False True True]print(np.logical_and(True, False))
# False
print(np.logical_and([True, False], [True, False]))
# [ True False]
print(np.logical_and(x > 1, x < 4))
# [False False True True False]print(np.logical_or(True, False))
# True
print(np.logical_or([True, False], [False, False]))
# [ True False]
print(np.logical_or(x < 1, x > 3))
# [ True False False False True]print(np.logical_xor(True, False))
# True
print(np.logical_xor([True, True, False, False], [True, False, True, False]))
# [False True True False]
print(np.logical_xor(x < 1, x > 3))
# [ True False False False True]
print(np.logical_xor(0, np.eye(2)))
# [[ True False]
# [False True]]
对照
numpy.greater(x1, x2, *args, **kwargs)
Return the truth value of (x1 > x2) element-wise.numpy.greater_equal(x1, x2, *args, **kwargs)
Return the truth value of (x1 >= x2) element-wise.numpy.equal(x1, x2, *args, **kwargs)
Return (x1 == x2) element-wise.numpy.not_equal(x1, x2, *args, **kwargs)
Return (x1 != x2) element-wise.numpy.less(x1, x2, *args, **kwargs)
Return the truth value of (x1 < x2) element-wise.numpy.less_equal(x1, x2, *args, **kwargs)
Return the truth value of (x1 =< x2) element-wise.
【例】numpy对以上对照函数进行了运算符的重载。
import numpy as npx = np.array([1, 2, 3, 4, 5, 6, 7, 8])y = x > 2
print(y)
print(np.greater(x, 2))
# [False False True True True True True True]y = x >= 2
print(y)
print(np.greater_equal(x, 2))
# [False True True True True True True True]y = x == 2
print(y)
print(np.equal(x, 2))
# [False True False False False False False False]y = x != 2
print(y)
print(np.not_equal(x, 2))
# [ True False True True True True True True]y = x < 2
print(y)
print(np.less(x, 2))
# [ True False False False False False False False]y = x <= 2
print(y)
print(np.less_equal(x, 2))
# [ True True False False False False False False]
【例】
import numpy as npx = np.array([[11, 12, 13, 14, 15],[16, 17, 18, 19, 20],[21, 22, 23, 24, 25],[26, 27, 28, 29, 30],[31, 32, 33, 34, 35]])
y = x > 20
print(y)
print(np.greater(x, 20))
# [[False False False False False]
# [False False False False False]
# [ True True True True True]
# [ True True True True True]
# [ True True True True True]]y = x >= 20
print(y)
print(np.greater_equal(x, 20))
# [[False False False False False]
# [False False False False True]
# [ True True True True True]
# [ True True True True True]
# [ True True True True True]]y = x == 20
print(y)
print(np.equal(x, 20))
# [[False False False False False]
# [False False False False True]
# [False False False False False]
# [False False False False False]
# [False False False False False]]y = x != 20
print(y)
print(np.not_equal(x, 20))
# [[ True True True True True]
# [ True True True True False]
# [ True True True True True]
# [ True True True True True]
# [ True True True True True]]y = x < 20
print(y)
print(np.less(x, 20))
# [[ True True True True True]
# [ True True True True False]
# [False False False False False]
# [False False False False False]
# [False False False False False]]y = x <= 20
print(y)
print(np.less_equal(x, 20))
# [[ True True True True True]
# [ True True True True True]
# [False False False False False]
# [False False False False False]
# [False False False False False]]
【例】
import numpy as npnp.random.seed(20200611)
x = np.array([[11, 12, 13, 14, 15],[16, 17, 18, 19, 20],[21, 22, 23, 24, 25],[26, 27, 28, 29, 30],[31, 32, 33, 34, 35]])y = np.random.randint(10, 40, [5, 5])
print(y)
# [[32 28 31 33 37]
# [23 37 37 30 29]
# [32 24 10 33 15]
# [27 17 10 36 16]
# [25 32 23 39 34]]z = x > y
print(z)
print(np.greater(x, y))
# [[False False False False False]
# [False False False False False]
# [False False True False True]
# [False True True False True]
# [ True False True False True]]z = x >= y
print(z)
print(np.greater_equal(x, y))
# [[False False False False False]
# [False False False False False]
# [False False True False True]
# [False True True False True]
# [ True True True False True]]z = x == y
print(z)
print(np.equal(x, y))
# [[False False False False False]
# [False False False False False]
# [False False False False False]
# [False False False False False]
# [False True False False False]]z = x != y
print(z)
print(np.not_equal(x, y))
# [[ True True True True True]
# [ True True True True True]
# [ True True True True True]
# [ True True True True True]
# [ True False True True True]]z = x < y
print(z)
print(np.less(x, y))
# [[ True True True True True]
# [ True True True True True]
# [ True True False True False]
# [ True False False True False]
# [False False False True False]]z = x <= y
print(z)
print(np.less_equal(x, y))
# [[ True True True True True]
# [ True True True True True]
# [ True True False True False]
# [ True False False True False]
# [False True False True False]]
【例】
import numpy as npx = np.array([[11, 12, 13, 14, 15],[16, 17, 18, 19, 20],[21, 22, 23, 24, 25],[26, 27, 28, 29, 30],[31, 32, 33, 34, 35]])np.random.seed(20200611)
y = np.random.randint(10, 50, 5)print(y)
# [32 37 30 24 10]z = x > y
print(z)
print(np.greater(x, y))
# [[False False False False True]
# [False False False False True]
# [False False False False True]
# [False False False True True]
# [False False True True True]]z = x >= y
print(z)
print(np.greater_equal(x, y))
# [[False False False False True]
# [False False False False True]
# [False False False True True]
# [False False False True True]
# [False False True True True]]z = x == y
print(z)
print(np.equal(x, y))
# [[False False False False False]
# [False False False False False]
# [False False False True False]
# [False False False False False]
# [False False False False False]]z = x != y
print(z)
print(np.not_equal(x, y))
# [[ True True True True True]
# [ True True True True True]
# [ True True True False True]
# [ True True True True True]
# [ True True True True True]]z = x < y
print(z)
print(np.less(x, y))
# [[ True True True True False]
# [ True True True True False]
# [ True True True False False]
# [ True True True False False]
# [ True True False False False]]z = x <= y
print(z)
print(np.less_equal(x, y))
# [[ True True True True False]
# [ True True True True False]
# [ True True True True False]
# [ True True True False False]
# [ True True False False False]]
numpy.isclose(a, b, rtol=1.e-5, atol=1.e-8, equal_nan=False)
Returns a boolean array where two arrays are element-wise equal within a tolerance.numpy.allclose(a, b, rtol=1.e-5, atol=1.e-8, equal_nan=False)
Returns True if two arrays are element-wise equal within a tolerance.numpy.all(isclose(a, b, rtol=rtol, atol=atol, equal_nan=equal_nan))
The tolerance values are positive, typically very small numbers. The relative difference (rtol
* abs(b
)) and the absolute difference atol
are added together to compare against the absolute difference between a
and b
.
判断是否为True的计算依据:
np.absolute(a - b) <= (atol + rtol * absolute(b))- atol:float,绝对公差。
- rtol:float,相对公差。
NaNs are treated as equal if they are in the same place and if equal_nan=True
. Infs are treated as equal if they are in the same place and of the same sign in both arrays.
【例】比较两个数组是否可以认为相等。
import numpy as npx = np.isclose([1e10, 1e-7], [1.00001e10, 1e-8])
print(x) # [ True False]x = np.allclose([1e10, 1e-7], [1.00001e10, 1e-8])
print(x) # Falsex = np.isclose([1e10, 1e-8], [1.00001e10, 1e-9])
print(x) # [ True True]x = np.allclose([1e10, 1e-8], [1.00001e10, 1e-9])
print(x) # Truex = np.isclose([1e10, 1e-8], [1.0001e10, 1e-9])
print(x) # [False True]x = np.allclose([1e10, 1e-8], [1.0001e10, 1e-9])
print(x) # Falsex = np.isclose([1.0, np.nan], [1.0, np.nan])
print(x) # [ True False]x = np.allclose([1.0, np.nan], [1.0, np.nan])
print(x) # Falsex = np.isclose([1.0, np.nan], [1.0, np.nan], equal_nan=True)
print(x) # [ True True]x = np.allclose([1.0, np.nan], [1.0, np.nan], equal_nan=True)
print(x) # True
当前活动
我是 终身学习者“老马”,一个长期践行“结伴式学习”理念的 中年大叔。
我崇尚分享,渴望成长,于2010年创立了“LSGO软件技术团队”,并加入了国内著名的开源组织“Datawhale”,也是“Dre@mtech”、“智能机器人研究中心”和“大数据与哲学社会科学实验室”的一员。
愿我们一起学习,一起进步,相互陪伴,共同成长。
后台回复「搜搜搜」,随机获取电子资源!
欢迎关注,请扫描二维码:
相关文章:
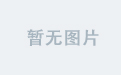
git获取指定release版本代码
首先手里必须有release的版本的备份出来的/.repo/manifests/default.xml文件,该文件记录了每个git库的在该版本下的具体的版本情况,整个代码的sync都是依据他来的; 1、repo sync 将本地代码更新至最新; 2、将手里的manifests.xml&…

【内网福音】如何离线部署Rancher
2019独角兽企业重金招聘Python工程师标准>>> 对于在公司内网环境中、无法访问互联网的用户而言,离线安装部署Rancher是解决问题的关键。本文是Rancher离线部署教程,专为内网用户排坑解难。 版本说明 OS:Centos7.3 Docker version:…
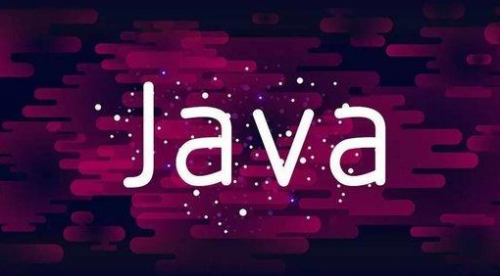
JAVA工资高吗
JAVA工资高吗?很多人都是非常关注这个问题的,近几年,java技术在互联网行业有了自己的一席之地,越来越多的人都投身到java技术行业,下面我们来看看详细的介绍。 JAVA工资高吗? 近年来,在美国、加拿大、澳大利亚、新加坡等发达国家…
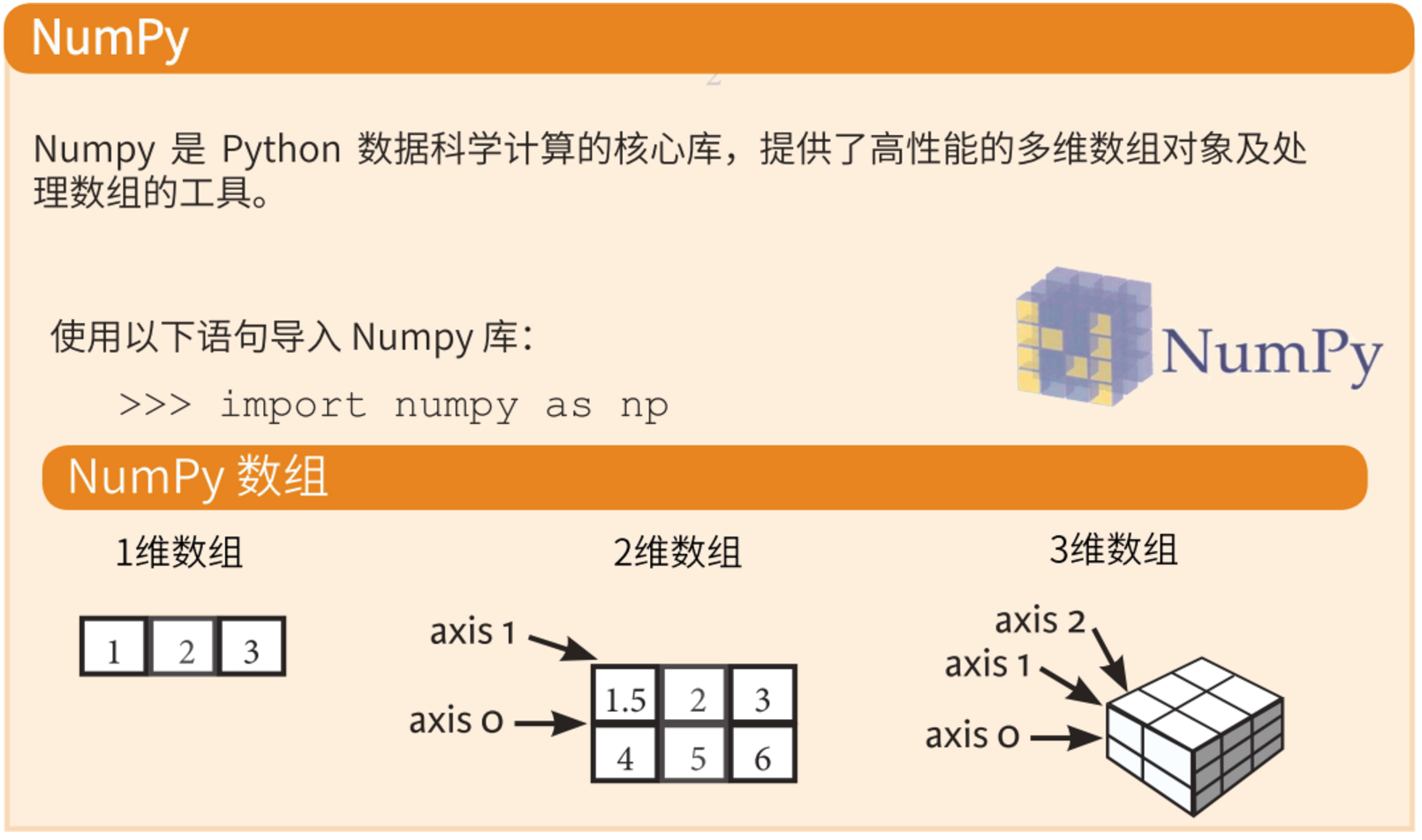
Numpy入门教程:06. 排序,搜索和计数
背景 什么是 NumPy 呢? NumPy 这个词来源于两个单词 – Numerical和Python。其是一个功能强大的 Python 库,可以帮助程序员轻松地进行数值计算,通常应用于以下场景: 执行各种数学任务,如:数值积分、微分、…

活动目录在构建核心过程中的八个关键点(下)
活动目录是一个面向Windows Server级别的目录服务。在之前的博客文章中介绍了活动目录设计中需要遵循的七个原则,今天在这里讲解有关《活动目录构建核心关键点》。 全文请见专题:http://os.51cto.com/art/201104/254054.htm 5. LDAP协议简介 LDAP的英文全…
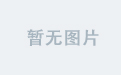
smarty变量调节器--count_words[计算词数]
计算变量里的词数 。 Example 5-7. count_words <?php$smarty->assign(articleTitle, Dealers Will Hear Car Talk at Noon.);?>Where template is:{$articleTitle}{$articleTitle|count_words}This will output:Dealers Will Hear Car Talk at Noon.7 See also cou…
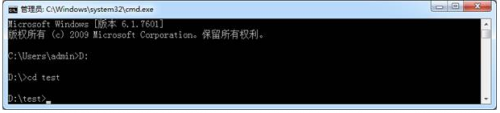
如何开发属于自己的第一个Java程序
学习java技术都是循序渐进的,搭建好了Java开发环境之后,下面就来学习一下如何开发Java程序。为了让初学者更好地完成第一个Java程序,接下来小编通过几个步骤进行逐一讲解。 1.编写Java源文件 在D盘根目录下新建一个test文件夹,并在…
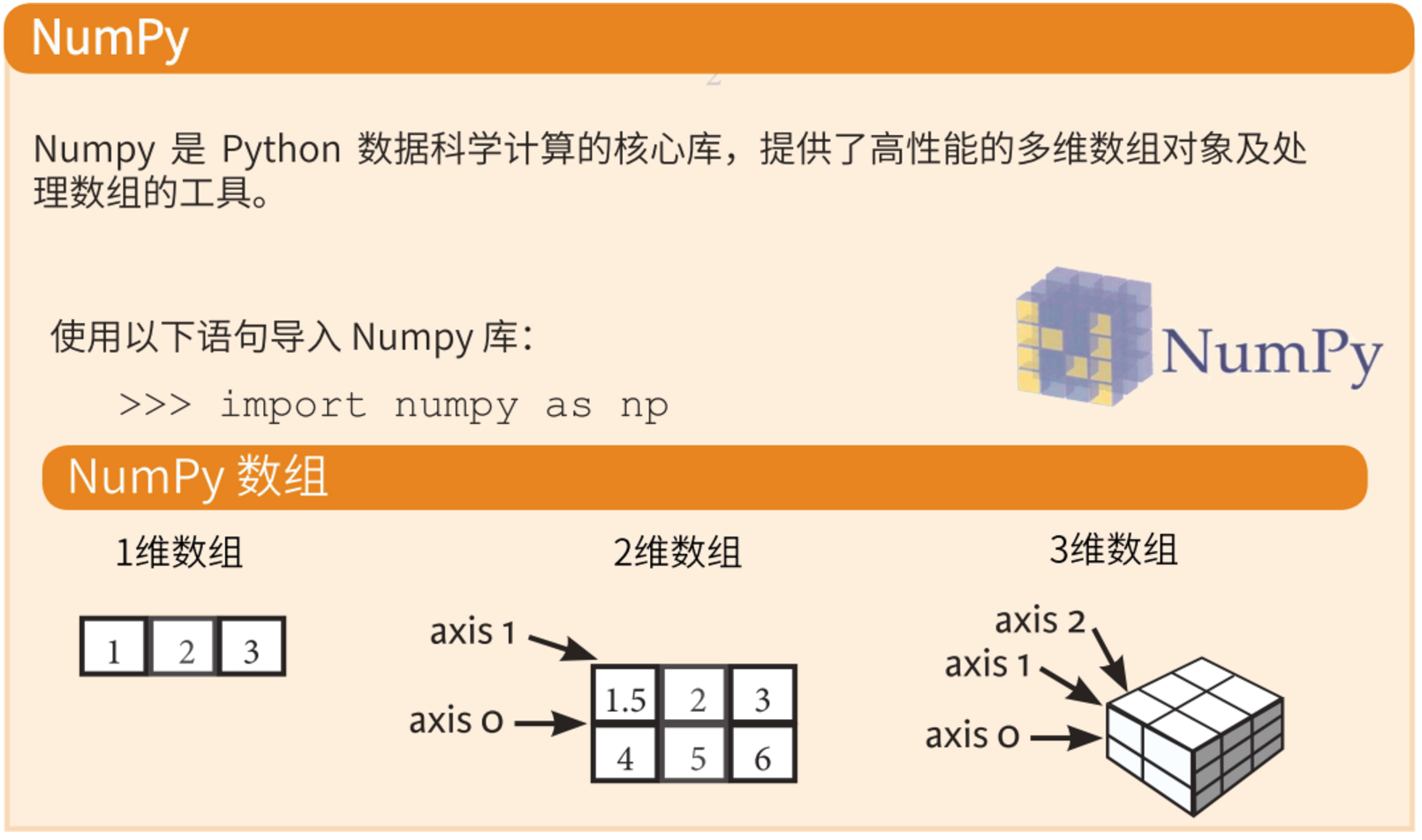
Numpy入门教程:07. 随机抽样
背景 什么是 NumPy 呢? NumPy 这个词来源于两个单词 – Numerical和Python。其是一个功能强大的 Python 库,可以帮助程序员轻松地进行数值计算,通常应用于以下场景: 执行各种数学任务,如:数值积分、微分、…
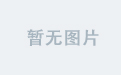
如何成为一个Android高手
很多Android开发者已经度过了初级、中级,如何成为一个Android高手呢? eoeAndroid就各个级别的程序员应该掌握哪些内容作为下面分类. 一、初级 1. 拥有娴熟的Java基础,理解设计模式,比如OOP语言的工厂模式要懂得. 2. 掌握Android U…

云终端处理器——Atom
由于上周展会的缘故,开始对云终端【I】处理器产生兴趣,接下来在“物理层”【II】来理解下X86-Atom,ARM,MIPS三种处理器,这是第一篇,主Atom Intel公司的官网简单介绍了一句 “英特尔 凌动【III】 处理器&am…

Java培训的学费标准是多少
很多想要进入到互联网行业的小伙伴都会选择java这门编程语言,java编程语言技术在互联网公司是起着非常重要的作用的,那么如今市面上的java培训机构有很多,选择报Java培训的学费标准是多少呢?来看看下面的详细介绍。 Java培训的学费…
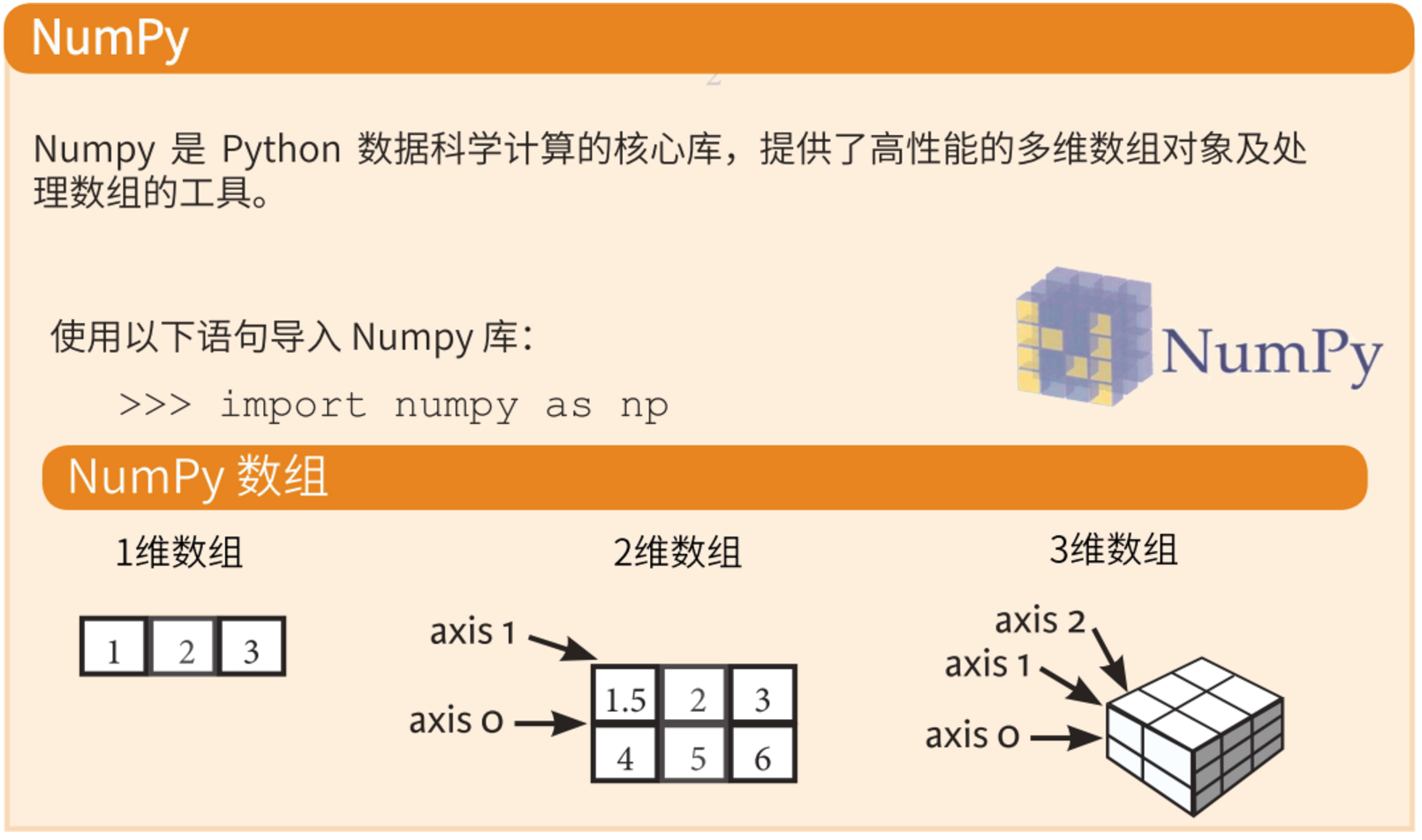
Numpy入门教程:08. 集合操作
背景 什么是 NumPy 呢? NumPy 这个词来源于两个单词 – Numerical和Python。其是一个功能强大的 Python 库,可以帮助程序员轻松地进行数值计算,通常应用于以下场景: 执行各种数学任务,如:数值积分、微分、…
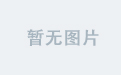
iPhone开发技巧之工具篇(4)--- 使用afconvert转换WAV文件
转载自:http://www.yifeiyang.net/iphone-development-skills-of-tool-papers-4-wav-file-conversion-using-afconvert/ 程序中经常使用 .WAV 的音效文件,虽然可以直接使用它,但是最好转换为 apple 推荐的 .CAF 格式。 这个时候我们就可以使用…
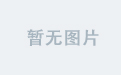
SQLite与pandas
以下链接对SQLite使用方法总结的很棒: http://www.cnblogs.com/yuxc/archive/2011/08/18/2143606.html 有关利用pandas读写QSLite的内容,可参考以下链接: http://pandas.pydata.org/pandas-docs/stable/generated/pandas.read_sql.html http:…
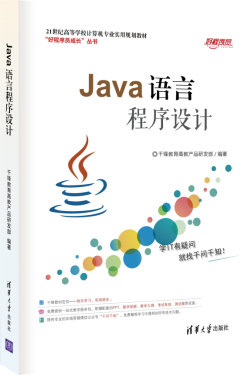
零基础学习java,这些书一定要看!
学习java技术除了看视频,看书也是非常重要的,尤其是零基础同学,本文包含学习Java各个阶段的书籍推荐,史上最全,学习Java,没有书籍怎么行,就好比出征没带兵器一个道理,这些书籍整理出…
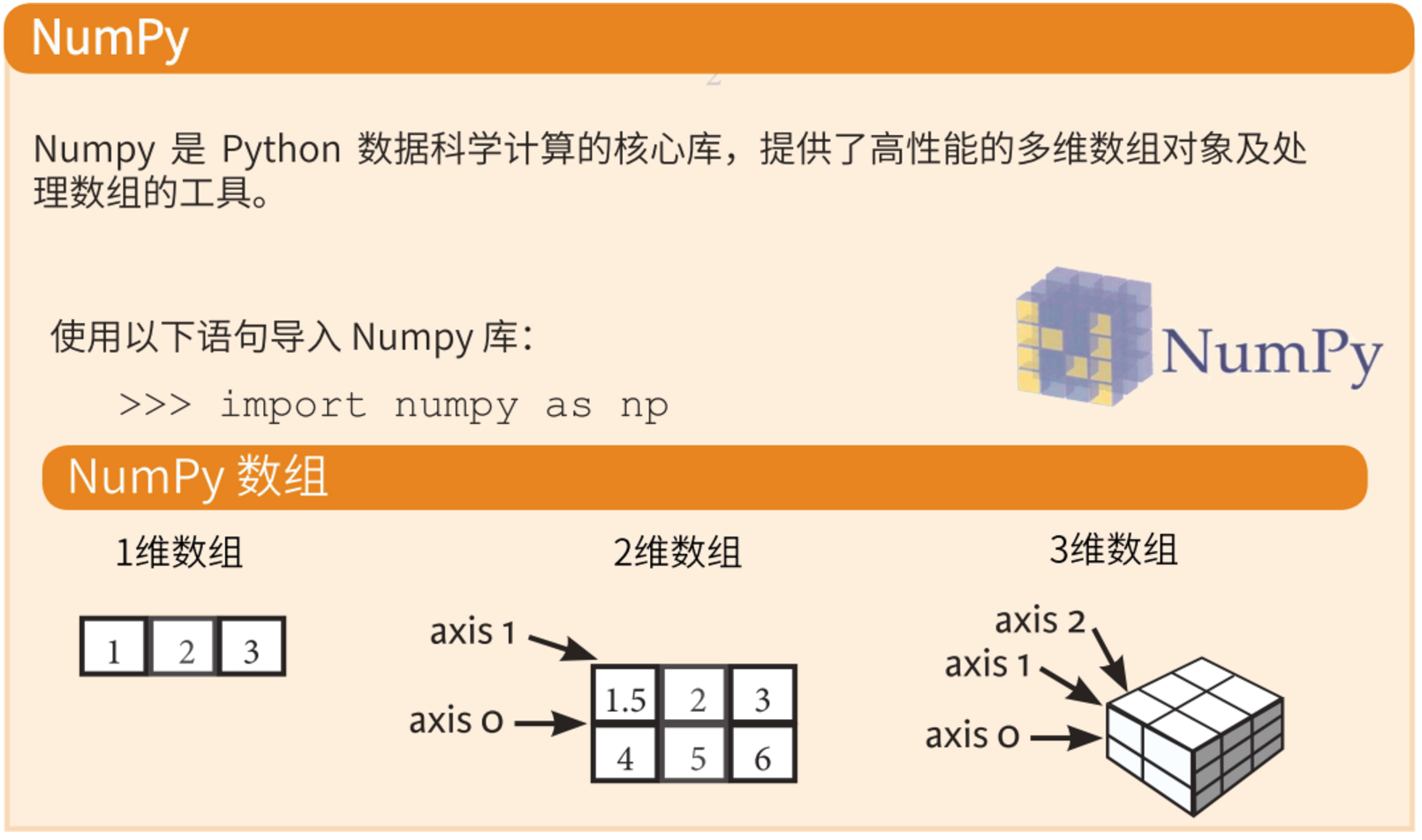
Numpy入门教程:练习作业01
序言 什么是 NumPy 呢? NumPy 这个词来源于两个单词 – Numerical和Python。其是一个功能强大的 Python 库,可以帮助程序员轻松地进行数值计算,通常应用于以下场景: 执行各种数学任务,如:数值积分、微分、…

转乱码UTF8和UTF-8网页编码
http://www.lovelucy.info/utf8-vs-utf-8.html#more-794 一、遇到的问题 曾经被字符集间复杂的转换搞怕了,正好新项目要求国际化,需要能够显示多种语言,于是一开始就规定统统使用 UTF-8 编码。 所有代码文件使用 UTF-8 编码存盘MySQL数据库所…
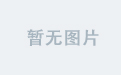
linux管道的执行顺序
最近有个疑问,netstat -antup|head -500 类似这条命令中,是netstat 执行完然后截取前500条记录还是,netstat 与head 并行执行,netstat 执行完500条就不再继续? 最终答案由酷学园darkdanger大大提供: 唔…
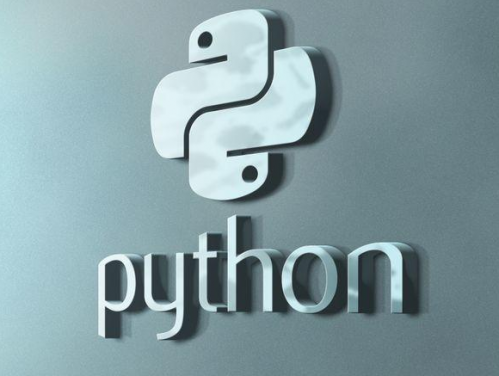
为什么学习Python数据分析
为什么学习Python数据分析?这是很多人都比较关注的一个问题,Python编程语言近几年在互联网行业是非常火爆的,尤其是在人工智能这一领域,它会大大的提高我们的工作效率等等,具体来看看下面的详细介绍就知道了。 为什么学习Python数…
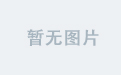
Python自动化开发学习6
引子 假设我们要在我们的程序里表示狗,狗有如下属性:名字、品种、颜色。那么可以先定义一个模板,然后调用这个模板生成各种狗。 def dog(name,d_type,color):data {name:name,d_type:d_type,color:color}return data d1 dog(小七,拉布拉多,…
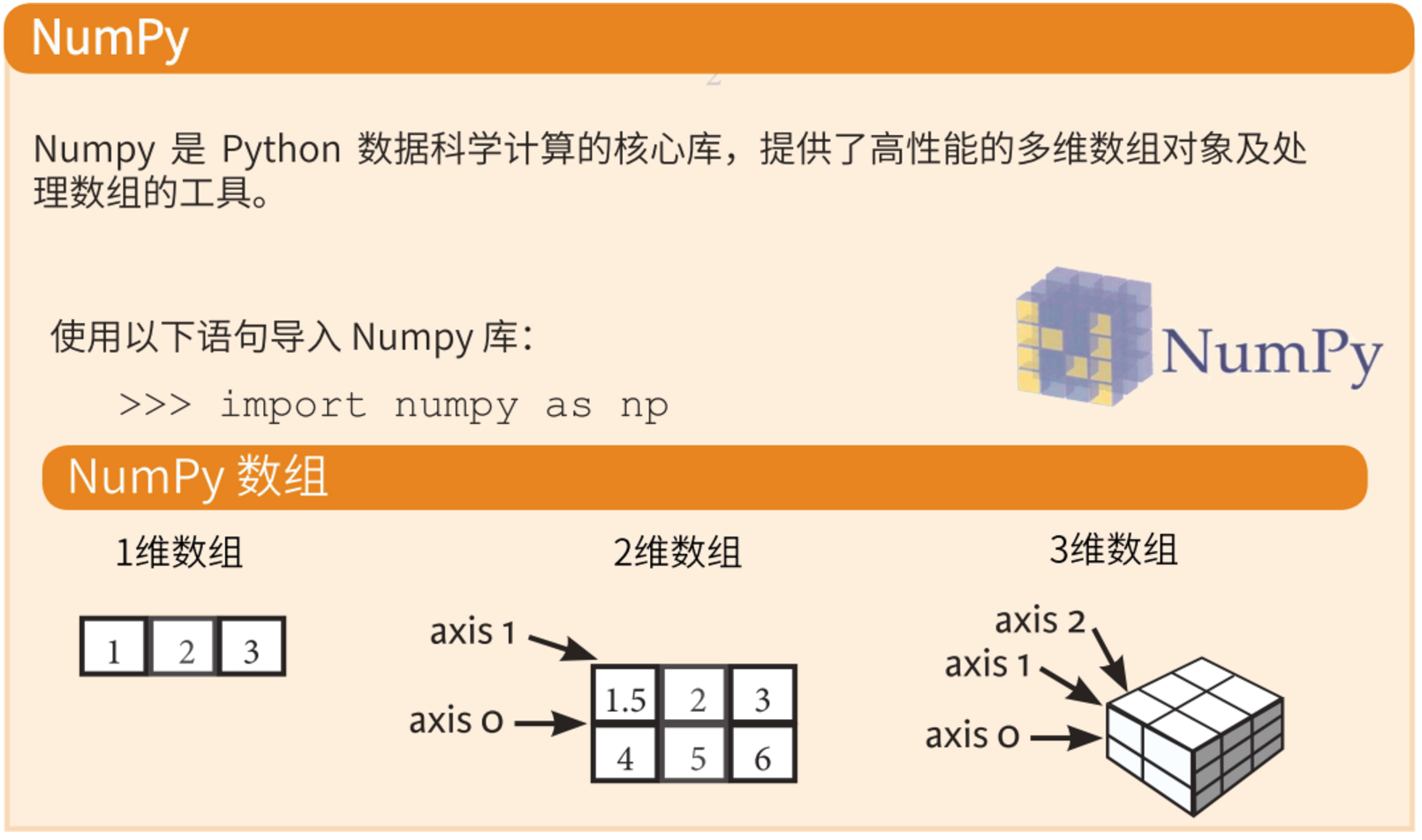
Numpy入门教程:09. 输入和输出
背景 什么是 NumPy 呢? NumPy 这个词来源于两个单词 – Numerical和Python。其是一个功能强大的 Python 库,可以帮助程序员轻松地进行数值计算,通常应用于以下场景: 执行各种数学任务,如:数值积分、微分、…
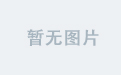
第二语言综合征
前些天在看一本书,温伯格的《理解专业程序员》,其中提到有的程序员得了第二语言综合征——在学习第三、第四门语言的时候很容易,但是学习第二门简直能要了他们的命。我当时就确定我患了这个毛病,因为我一直想了解Java语言…
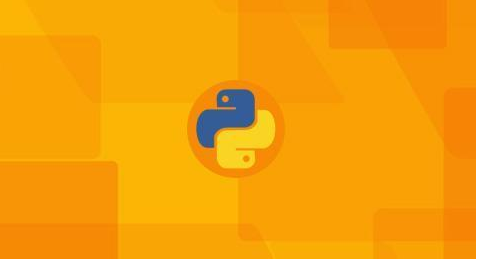
Python文件操作:finally子句的使用
finally子句与try-except语句连用时,无论try-except是否捕获到异常,finally子句后的代码都要执行,其语法格式如下: try: 可能出错的语句 ......except: 出错后的执行语句finally: 无论是否出错都会执行的语句 Python在处理文件时&…
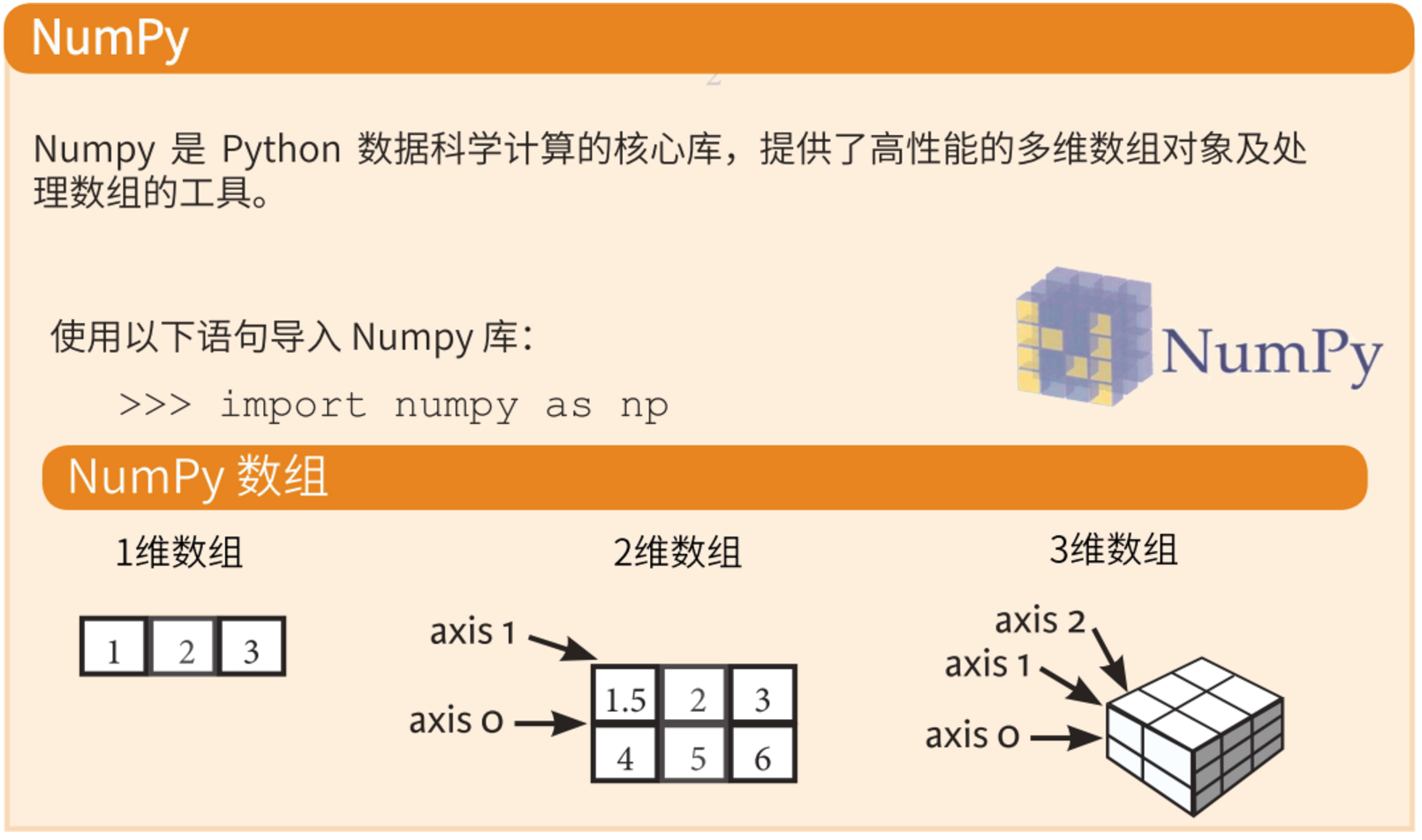
Numpy入门教程:练习作业02
背景 什么是 NumPy 呢? NumPy 这个词来源于两个单词 – Numerical和Python。其是一个功能强大的 Python 库,可以帮助程序员轻松地进行数值计算,通常应用于以下场景: 执行各种数学任务,如:数值积分、微分、…
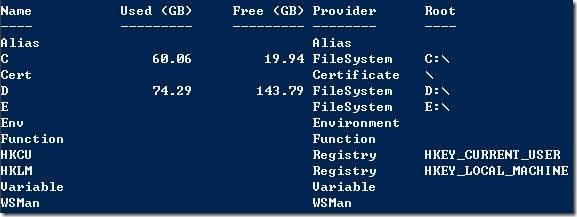
PowerShell 导入 SQL Server 的 PS 模块
接触过UNIX或者Linux 的朋友都知道此类系统有着功能强大、无所不能的壳程序,称之为Shell。微软公司于2006年第四季度正式发布PowerShell,它的出现标志着, 微软公司向服务器领域迈出了重要的一步, 不仅提供简便的图形化操作界面,同时提供类似于Unix, Linu…
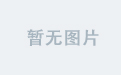
ARM嵌入式操作系统启动
任何一个操作系统的启动都至少关注两个方面:1,程序运行栈的初始化。2,处理器外设的初始化。 在ARMv6以及以前的体系结构中,定义了七种模式分别为&…
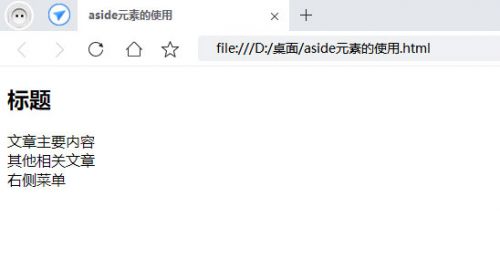
Html5 aside标签的用法和作用
aside元素用来定义当前页面或者文章的附属信息部分,它可以包含与当前页面或主要内容相关的引用、侧边栏、广告、导航条等其他类似的有别于主要内容的部分。 aside元素的用法主要分为两种。 ● 被包含在article元素内作为主要内容的附属信息。 ● 在article元素之外使…
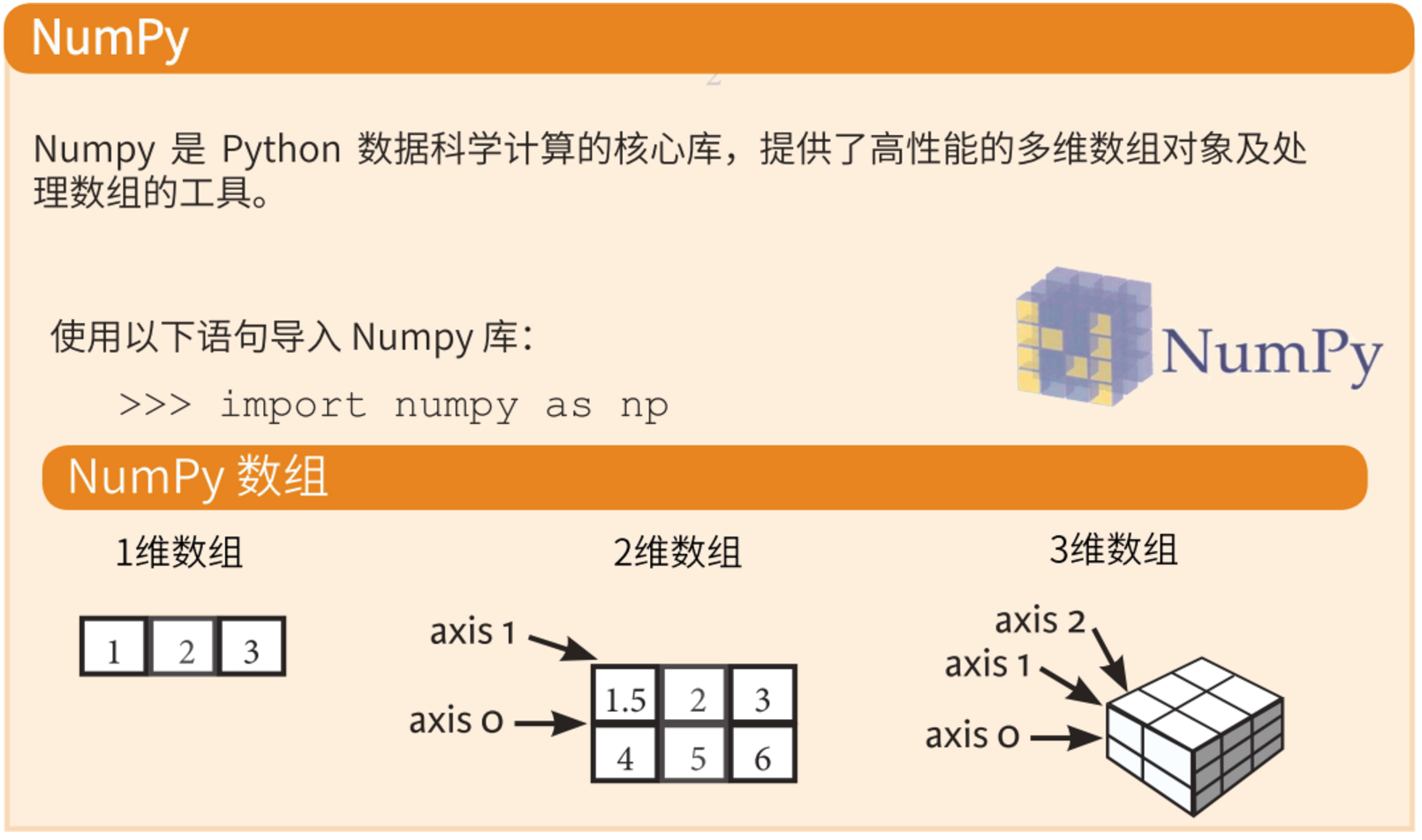
Numpy入门教程:10. 统计相关
背景 什么是 NumPy 呢? NumPy 这个词来源于两个单词 – Numerical和Python。其是一个功能强大的 Python 库,可以帮助程序员轻松地进行数值计算,通常应用于以下场景: 执行各种数学任务,如:数值积分、微分、…
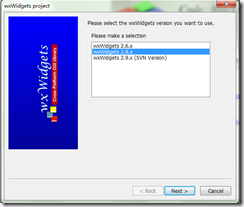
Windows 7+Code::Blocks+wxWidgets实录(一)
环境配置篇 玩过Linux的人应该对Code::Blocks和wxWidgets并不陌生。 Code::Blocks是一款非常有名的代码编辑器,在linux下用不惯vim的话,这是个不错的选择。但千万不要把它和编译器混淆,CB本身并没有独立编译程序的功能,需要调用系…
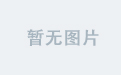
技巧:两部解决U盘安装windows 7
第一步:准备一个4G的U盘并使用disk part 工具制作成引导盘1、在运行中输入cmd 回车2、在黑色的命令提示符界面中输入Diskpart3、插入U盘 并输入List Disk查看4、输入Select Disk 1 (选择你的U盘所在的标识)5、输入clean 清除旧的信息6、输入c…