吴恩达老师深度学习视频课笔记:单隐含层神经网络公式推导及C++实现(二分类)
关于逻辑回归的公式推导和实现可以参考: http://blog.csdn.net/fengbingchun/article/details/79346691
下面是在逻辑回归的基础上,对单隐含层的神经网络进行公式推导:
选择激活函数时的一些经验:不同层的激活函数可以不一样。如果输出层值是0或1,在做二元分类,可以选择sigmoid作为输出层的激活函数;其它层可以选择默认(不确定情况下)使用ReLU作为激活函数。使用ReLU作为激活函数一般比使用sigmoid或tanh在使用梯度下降法时学习速度会快很多。一般在深度学习中都需要使用非线性激活函数。唯一能用线性激活函数的地方通常也就只有输出层。
深度学习中的权值w不能初始化为0,偏置b可以初始化为0.
反向传播中的求导需要使用微积分的链式法则。
以下code是完全按照上面的推导公式进行实现的,对数字0和1进行二分类。训练数据集为从MNIST中train中随机选取的0、1各10个图像;测试数据集为从MNIST中test中随机选取的0、1各10个图像,如下图,其中第一排前10个0用于训练,后10个0用于测试;第二排前10个1用于训练,后10个1用于测试:
关于数据集MNIST的介绍可以参考: http://blog.csdn.net/fengbingchun/article/details/49611549
single_hidden_layer.hpp:
#ifndef FBC_SRC_NN_SINGLE_HIDDEN_LAYER_HPP_
#define FBC_SRC_NN_SINGLE_HIDDEN_LAYER_HPP_#include <string>
#include <vector>namespace ANN {template<typename T>
class SingleHiddenLayer { // two categories
public:typedef enum ActivationFunctionType {Sigmoid = 0,TanH = 1,ReLU = 2,Leaky_ReLU = 3} ActivationFunctionType;SingleHiddenLayer() = default;int init(const T* data, const T* labels, int train_num, int feature_length,int hidden_layer_node_num = 20, T learning_rate = 0.00001, int iterations = 10000, int hidden_layer_activation_type = 2, int output_layer_activation_type = 0);int train(const std::string& model);int load_model(const std::string& model);T predict(const T* data, int feature_length) const;private:T calculate_activation_function(T value, ActivationFunctionType type) const;T calcuate_activation_function_derivative(T value, ActivationFunctionType type) const;int store_model(const std::string& model) const;void init_train_variable();void init_w_and_b();ActivationFunctionType hidden_layer_activation_type = ReLU;ActivationFunctionType output_layer_activation_type = Sigmoid;std::vector<std::vector<T>> x; // training setstd::vector<T> y; // ground truth labelsint iterations = 10000;int m = 0; // train samples numint feature_length = 0;T alpha = (T)0.00001; // learning ratestd::vector<std::vector<T>> w1, w2; // weightsstd::vector<T> b1, b2; // thresholdint hidden_layer_node_num = 10;int output_layer_node_num = 1;T J = (T)0.;std::vector<std::vector<T>> dw1, dw2;std::vector<T> db1, db2;std::vector<std::vector<T>> z1, a1, z2, a2, da2, dz2, da1, dz1;
}; // class SingleHiddenLayer} // namespace ANN#endif // FBC_SRC_NN_SINGLE_HIDDEN_LAYER_HPP_
single_hidden_layer.cpp:#include "single_hidden_layer.hpp"
#include <algorithm>
#include <cmath>
#include <random>
#include <memory>
#include "common.hpp"namespace ANN {template<typename T>
int SingleHiddenLayer<T>::init(const T* data, const T* labels, int train_num, int feature_length,int hidden_layer_node_num, T learning_rate, int iterations, int hidden_layer_activation_type, int output_layer_activation_type)
{CHECK(train_num > 2 && feature_length > 0 && hidden_layer_node_num > 0 && learning_rate > 0 && iterations > 0);CHECK(hidden_layer_activation_type >= 0 && hidden_layer_activation_type < 4);CHECK(output_layer_activation_type >= 0 && output_layer_activation_type < 4);this->hidden_layer_node_num = hidden_layer_node_num;this->alpha = learning_rate;this->iterations = iterations;this->hidden_layer_activation_type = static_cast<ActivationFunctionType>(hidden_layer_activation_type);this->output_layer_activation_type = static_cast<ActivationFunctionType>(output_layer_activation_type);this->m = train_num;this->feature_length = feature_length;this->x.resize(train_num);this->y.resize(train_num);for (int i = 0; i < train_num; ++i) {const T* p = data + i * feature_length;this->x[i].resize(feature_length);for (int j = 0; j < feature_length; ++j) {this->x[i][j] = p[j];}this->y[i] = labels[i];}return 0;
}template<typename T>
void SingleHiddenLayer<T>::init_train_variable()
{J = (T)0.;dw1.resize(this->hidden_layer_node_num);db1.resize(this->hidden_layer_node_num);for (int i = 0; i < this->hidden_layer_node_num; ++i) {dw1[i].resize(this->feature_length);for (int j = 0; j < this->feature_length; ++j) {dw1[i][j] = (T)0.;}db1[i] = (T)0.;}dw2.resize(this->output_layer_node_num);db2.resize(this->output_layer_node_num);for (int i = 0; i < this->output_layer_node_num; ++i) {dw2[i].resize(this->hidden_layer_node_num);for (int j = 0; j < this->hidden_layer_node_num; ++j) {dw2[i][j] = (T)0.;}db2[i] = (T)0.;}z1.resize(this->m); a1.resize(this->m); da1.resize(this->m); dz1.resize(this->m);for (int i = 0; i < this->m; ++i) {z1[i].resize(this->hidden_layer_node_num);a1[i].resize(this->hidden_layer_node_num);dz1[i].resize(this->hidden_layer_node_num);da1[i].resize(this->hidden_layer_node_num);for (int j = 0; j < this->hidden_layer_node_num; ++j) {z1[i][j] = (T)0.;a1[i][j] = (T)0.;dz1[i][j] = (T)0.;da1[i][j] = (T)0.;}}z2.resize(this->m); a2.resize(this->m); da2.resize(this->m); dz2.resize(this->m);for (int i = 0; i < this->m; ++i) {z2[i].resize(this->output_layer_node_num);a2[i].resize(this->output_layer_node_num);dz2[i].resize(this->output_layer_node_num);da2[i].resize(this->output_layer_node_num);for (int j = 0; j < this->output_layer_node_num; ++j) {z2[i][j] = (T)0.;a2[i][j] = (T)0.;dz2[i][j] = (T)0.;da2[i][j] = (T)0.;}}
}template<typename T>
void SingleHiddenLayer<T>::init_w_and_b()
{w1.resize(this->hidden_layer_node_num); // (hidden_layer_node_num, feature_length)b1.resize(this->hidden_layer_node_num); // (hidden_layer_node_num, 1)w2.resize(this->output_layer_node_num); // (output_layer_node_num, hidden_layer_node_num)b2.resize(this->output_layer_node_num); // (output_layer_node_num, 1)std::random_device rd;std::mt19937 generator(rd());std::uniform_real_distribution<T> distribution(-0.01, 0.01);for (int i = 0; i < this->hidden_layer_node_num; ++i) {w1[i].resize(this->feature_length);for (int j = 0; j < this->feature_length; ++j) {w1[i][j] = distribution(generator);}b1[i] = distribution(generator);}for (int i = 0; i < this->output_layer_node_num; ++i) {w2[i].resize(this->hidden_layer_node_num);for (int j = 0; j < this->hidden_layer_node_num; ++j) {w2[i][j] = distribution(generator);}b2[i] = distribution(generator);}
}template<typename T>
int SingleHiddenLayer<T>::train(const std::string& model)
{CHECK(x.size() == y.size());CHECK(output_layer_node_num == 1);init_w_and_b();for (int iter = 0; iter < this->iterations; ++iter) {init_train_variable();for (int i = 0; i < this->m; ++i) {for (int p = 0; p < this->hidden_layer_node_num; ++p) {for (int q = 0; q < this->feature_length; ++q) {z1[i][p] += w1[p][q] * x[i][q];}z1[i][p] += b1[p]; // z[1](i)=w[1]*x(i)+b[1]a1[i][p] = calculate_activation_function(z1[i][p], this->hidden_layer_activation_type); // a[1](i)=g[1](z[1](i))}for (int p = 0; p < this->output_layer_node_num; ++p) {for (int q = 0; q < this->hidden_layer_node_num; ++q) {z2[i][p] += w2[p][q] * a1[i][q];}z2[i][p] += b2[p]; // z[2](i)=w[2]*a[1](i)+b[2]a2[i][p] = calculate_activation_function(z2[i][p], this->output_layer_activation_type); // a[2](i)=g[2](z[2](i))}for (int p = 0; p < this->output_layer_node_num; ++p) {J += -(y[i] * std::log(a2[i][p]) + (1 - y[i] * std::log(1 - a2[i][p]))); // J+=-[y(i)*loga[2](i)+(1-y(i))*log(1-a[2](i))]}for (int p = 0; p < this->output_layer_node_num; ++p) {da2[i][p] = -(y[i] / a2[i][p]) + ((1. - y[i]) / (1. - a2[i][p])); // da[2](i)=-(y(i)/a[2](i))+((1-y(i))/(1.-a[2](i)))dz2[i][p] = da2[i][p] * calcuate_activation_function_derivative(z2[i][p], this->output_layer_activation_type); // dz[2](i)=da[2](i)*g[2]'(z[2](i))}for (int p = 0; p < this->output_layer_node_num; ++p) {for (int q = 0; q < this->hidden_layer_node_num; ++q) {dw2[p][q] += dz2[i][p] * a1[i][q]; // dw[2]+=dz[2](i)*(a[1](i)^T)}db2[p] += dz2[i][p]; // db[2]+=dz[2](i)}for (int p = 0; p < this->hidden_layer_node_num; ++p) {for (int q = 0; q < this->output_layer_node_num; ++q) {da1[i][p] = w2[q][p] * dz2[i][q]; // (da[1](i)=w[2](i)^T)*dz[2](i)dz1[i][p] = da1[i][p] * calcuate_activation_function_derivative(z1[i][p], this->hidden_layer_activation_type); // dz[1](i)=da[1](i)*(g[1]'(z[1](i)))}}for (int p = 0; p < this->hidden_layer_node_num; ++p) {for (int q = 0; q < this->feature_length; ++q) {dw1[p][q] += dz1[i][p] * x[i][q]; // dw[1]+=dz[1](i)*(x(i)^T)}db1[p] += dz1[i][p]; // db[1]+=dz[1](i)}}J /= m;for (int p = 0; p < this->output_layer_node_num; ++p) {for (int q = 0; q < this->hidden_layer_node_num; ++q) {dw2[p][q] = dw2[p][q] / m; // dw[2] /=m}db2[p] = db2[p] / m; // db[2] /=m}for (int p = 0; p < this->hidden_layer_node_num; ++p) {for (int q = 0; q < this->feature_length; ++q) {dw1[p][q] = dw1[p][q] / m; // dw[1] /= m}db1[p] = db1[p] / m; // db[1] /= m}for (int p = 0; p < this->output_layer_node_num; ++p) {for (int q = 0; q < this->hidden_layer_node_num; ++q) {w2[p][q] = w2[p][q] - this->alpha * dw2[p][q]; // w[2]=w[2]-alpha*dw[2]}b2[p] = b2[p] - this->alpha * db2[p]; // b[2]=b[2]-alpha*db[2]}for (int p = 0; p < this->hidden_layer_node_num; ++p) {for (int q = 0; q < this->feature_length; ++q) {w1[p][q] = w1[p][q] - this->alpha * dw1[p][q]; // w[1]=w[1]-alpha*dw[1]}b1[p] = b1[p] - this->alpha * db1[p]; // b[1]=b[1]-alpha*db[1]}}CHECK(store_model(model) == 0);
}template<typename T>
int SingleHiddenLayer<T>::load_model(const std::string& model)
{std::ifstream file;file.open(model.c_str(), std::ios::binary);if (!file.is_open()) {fprintf(stderr, "open file fail: %s\n", model.c_str());return -1;}file.read((char*)&this->hidden_layer_node_num, sizeof(int));file.read((char*)&this->output_layer_node_num, sizeof(int));int type{ -1 };file.read((char*)&type, sizeof(int));this->hidden_layer_activation_type = static_cast<ActivationFunctionType>(type);file.read((char*)&type, sizeof(int));this->output_layer_activation_type = static_cast<ActivationFunctionType>(type);file.read((char*)&this->feature_length, sizeof(int));this->w1.resize(this->hidden_layer_node_num);for (int i = 0; i < this->hidden_layer_node_num; ++i) {this->w1[i].resize(this->feature_length);}this->b1.resize(this->hidden_layer_node_num);this->w2.resize(this->output_layer_node_num);for (int i = 0; i < this->output_layer_node_num; ++i) {this->w2[i].resize(this->hidden_layer_node_num);}this->b2.resize(this->output_layer_node_num);int length = w1.size() * w1[0].size();std::unique_ptr<T[]> data1(new T[length]);T* p = data1.get();file.read((char*)p, sizeof(T)* length);file.read((char*)this->b1.data(), sizeof(T)* b1.size());int count{ 0 };for (int i = 0; i < this->w1.size(); ++i) {for (int j = 0; j < this->w1[0].size(); ++j) {w1[i][j] = p[count++];}}length = w2.size() * w2[0].size();std::unique_ptr<T[]> data2(new T[length]);p = data2.get();file.read((char*)p, sizeof(T)* length);file.read((char*)this->b2.data(), sizeof(T)* b2.size());count = 0;for (int i = 0; i < this->w2.size(); ++i) {for (int j = 0; j < this->w2[0].size(); ++j) {w2[i][j] = p[count++];}}file.close();return 0;
}template<typename T>
T SingleHiddenLayer<T>::predict(const T* data, int feature_length) const
{CHECK(feature_length == this->feature_length);CHECK(this->output_layer_node_num == 1);CHECK(this->hidden_layer_activation_type >= 0 && this->hidden_layer_activation_type < 4);CHECK(this->output_layer_activation_type >= 0 && this->output_layer_activation_type < 4);std::vector<T> z1(this->hidden_layer_node_num, (T)0.), a1(this->hidden_layer_node_num, (T)0.),z2(this->output_layer_node_num, (T)0.), a2(this->output_layer_node_num, (T)0.);for (int p = 0; p < this->hidden_layer_node_num; ++p) {for (int q = 0; q < this->feature_length; ++q) {z1[p] += w1[p][q] * data[q];}z1[p] += b1[p];a1[p] = calculate_activation_function(z1[p], this->hidden_layer_activation_type);}for (int p = 0; p < this->output_layer_node_num; ++p) {for (int q = 0; q < this->hidden_layer_node_num; ++q) {z2[p] += w2[p][q] * a1[q];}z2[p] += b2[p];a2[p] = calculate_activation_function(z2[p], this->output_layer_activation_type);}return a2[0];
}template<typename T>
T SingleHiddenLayer<T>::calculate_activation_function(T value, ActivationFunctionType type) const
{T result{ 0 };switch (type) {case Sigmoid:result = (T)1. / ((T)1. + std::exp(-value));break;case TanH:result = (T)(std::exp(value) - std::exp(-value)) / (std::exp(value) + std::exp(-value));break;case ReLU:result = std::max((T)0., value);break;case Leaky_ReLU:result = std::max((T)0.01*value, value);break;default:CHECK(0);break;}return result;
}template<typename T>
T SingleHiddenLayer<T>::calcuate_activation_function_derivative(T value, ActivationFunctionType type) const
{T result{ 0 };switch (type) {case Sigmoid: {T tmp = calculate_activation_function(value, Sigmoid);result = tmp * (1. - tmp);}break;case TanH: {T tmp = calculate_activation_function(value, TanH);result = 1 - tmp * tmp;}break;case ReLU:result = value < 0. ? 0. : 1.;break;case Leaky_ReLU:result = value < 0. ? 0.01 : 1.;break;default:CHECK(0);break;}return result;
}template<typename T>
int SingleHiddenLayer<T>::store_model(const std::string& model) const
{std::ofstream file;file.open(model.c_str(), std::ios::binary);if (!file.is_open()) {fprintf(stderr, "open file fail: %s\n", model.c_str());return -1;}file.write((char*)&this->hidden_layer_node_num, sizeof(int));file.write((char*)&this->output_layer_node_num, sizeof(int));int type = this->hidden_layer_activation_type;file.write((char*)&type, sizeof(int));type = this->output_layer_activation_type;file.write((char*)&type, sizeof(int));file.write((char*)&this->feature_length, sizeof(int));int length = w1.size() * w1[0].size();std::unique_ptr<T[]> data1(new T[length]);T* p = data1.get();for (int i = 0; i < w1.size(); ++i) {for (int j = 0; j < w1[0].size(); ++j) {p[i * w1[0].size() + j] = w1[i][j];}}file.write((char*)p, sizeof(T)* length);file.write((char*)this->b1.data(), sizeof(T)* this->b1.size());length = w2.size() * w2[0].size();std::unique_ptr<T[]> data2(new T[length]);p = data2.get();for (int i = 0; i < w2.size(); ++i) {for (int j = 0; j < w2[0].size(); ++j) {p[i * w2[0].size() + j] = w2[i][j];}}file.write((char*)p, sizeof(T)* length);file.write((char*)this->b2.data(), sizeof(T)* this->b2.size());file.close();return 0;
}template class SingleHiddenLayer<float>;
template class SingleHiddenLayer<double>;} // namespace ANN
main.cpp:#include "funset.hpp"
#include <iostream>
#include "perceptron.hpp"
#include "BP.hpp""
#include "CNN.hpp"
#include "linear_regression.hpp"
#include "naive_bayes_classifier.hpp"
#include "logistic_regression.hpp"
#include "common.hpp"
#include "knn.hpp"
#include "decision_tree.hpp"
#include "pca.hpp"
#include <opencv2/opencv.hpp>
#include "logistic_regression2.hpp"
#include "single_hidden_layer.hpp"// ====================== single hidden layer(two categories) ===============
int test_single_hidden_layer_train()
{const std::string image_path{ "E:/GitCode/NN_Test/data/images/digit/handwriting_0_and_1/" };cv::Mat data, labels;for (int i = 1; i < 11; ++i) {const std::vector<std::string> label{ "0_", "1_" };for (const auto& value : label) {std::string name = std::to_string(i);name = image_path + value + name + ".jpg";cv::Mat image = cv::imread(name, 0);if (image.empty()) {fprintf(stderr, "read image fail: %s\n", name.c_str());return -1;}data.push_back(image.reshape(0, 1));}}data.convertTo(data, CV_32F);std::unique_ptr<float[]> tmp(new float[20]);for (int i = 0; i < 20; ++i) {if (i % 2 == 0) tmp[i] = 0.f;else tmp[i] = 1.f;}labels = cv::Mat(20, 1, CV_32FC1, tmp.get());ANN::SingleHiddenLayer<float> shl;const float learning_rate{ 0.00001f };const int iterations{ 10000 };const int hidden_layer_node_num{ static_cast<int>(std::log2(data.cols)) };const int hidden_layer_activation_type{ ANN::SingleHiddenLayer<float>::ReLU };const int output_layer_activation_type{ ANN::SingleHiddenLayer<float>::Sigmoid };int ret = shl.init((float*)data.data, (float*)labels.data, data.rows, data.cols,hidden_layer_node_num, learning_rate, iterations, hidden_layer_activation_type, output_layer_activation_type);if (ret != 0) {fprintf(stderr, "single_hidden_layer(two categories) init fail: %d\n", ret);return -1;}const std::string model{ "E:/GitCode/NN_Test/data/single_hidden_layer.model" };ret = shl.train(model);if (ret != 0) {fprintf(stderr, "single_hidden_layer(two categories) train fail: %d\n", ret);return -1;}return 0;
}int test_single_hidden_layer_predict()
{const std::string image_path{ "E:/GitCode/NN_Test/data/images/digit/handwriting_0_and_1/" };cv::Mat data, labels, result;for (int i = 11; i < 21; ++i) {const std::vector<std::string> label{ "0_", "1_" };for (const auto& value : label) {std::string name = std::to_string(i);name = image_path + value + name + ".jpg";cv::Mat image = cv::imread(name, 0);if (image.empty()) {fprintf(stderr, "read image fail: %s\n", name.c_str());return -1;}data.push_back(image.reshape(0, 1));}}data.convertTo(data, CV_32F);std::unique_ptr<int[]> tmp(new int[20]);for (int i = 0; i < 20; ++i) {if (i % 2 == 0) tmp[i] = 0;else tmp[i] = 1;}labels = cv::Mat(20, 1, CV_32SC1, tmp.get());CHECK(data.rows == labels.rows);const std::string model{ "E:/GitCode/NN_Test/data/single_hidden_layer.model" };ANN::SingleHiddenLayer<float> shl;int ret = shl.load_model(model);if (ret != 0) {fprintf(stderr, "load single_hidden_layer(two categories) model fail: %d\n", ret);return -1;}for (int i = 0; i < data.rows; ++i) {float probability = shl.predict((float*)(data.row(i).data), data.cols);fprintf(stdout, "probability: %.6f, ", probability);if (probability > 0.5) fprintf(stdout, "predict result: 1, ");else fprintf(stdout, "predict result: 0, ");fprintf(stdout, "actual result: %d\n", ((int*)(labels.row(i).data))[0]);}return 0;
}
执行结果如下:由执行结果可知,测试图像全部分类正确。 GitHub: https://github.com/fengbingchun/NN_Test
相关文章:
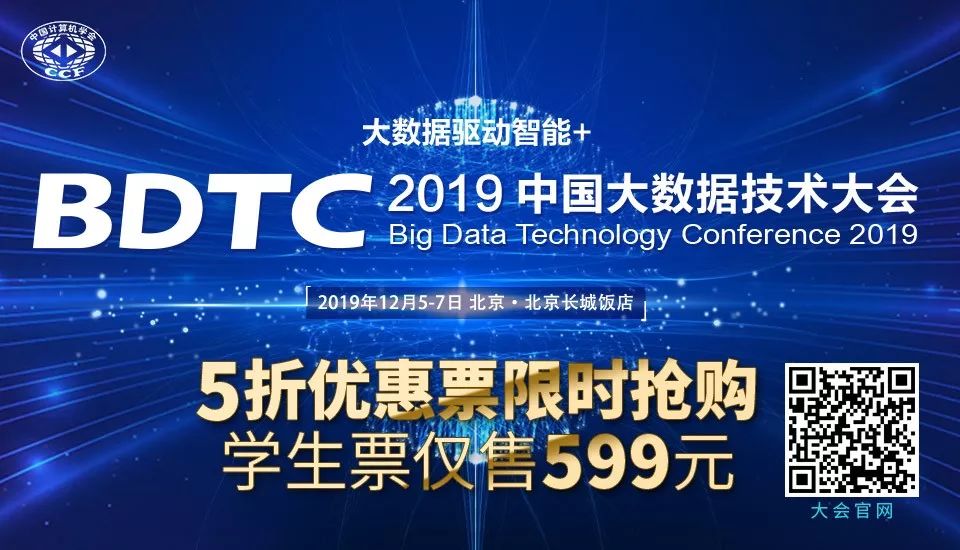
「2019中国大数据技术大会」超值学生票来啦!
大会官网:https://t.csdnimg.cn/U1wA经过11年的沉淀与发展,中国大数据技术大会见证了大数据技术生态在中国的建立、发展和成熟,已经成为国内大数据行业极具影响力的盛会,也是大数据人非常期待的年度深度分享盛会。在新的时代背景下…
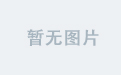
校验正确获取对象或者数组的属性方法(babel-plugin-idx/_.get)
背景: 开发中经常遇到取值属性的时候,需要校验数值的有效性。 例如: 获取props对象里面的friends属性 props.user && props.user.friends && props.user.friends[0] && props.user.friends[0].friends 对于深层的对…

Ring Tone Manager on Windows Mobile
2019独角兽企业重金招聘Python工程师标准>>> 手机铃声经常能够体现一个人的个性,有些哥们儿在自习室不把手机设置成震动,一来电就#$^%^&^%#$&$*,声音还很大,唯恐别人听不到。 Windows Mobile设备上如何来设置手…
OpenCV3.3中K-Means聚类接口简介及使用
OpenCV3.3中给出了K-均值聚类(K-Means)的实现,即接口cv::kmeans,接口的声明在include/opencv2/core.hpp文件中,实现在modules/core/src/kmeans.cpp文件中,其中:下面对此接口中的参数作个简单说明:(1)、data:…
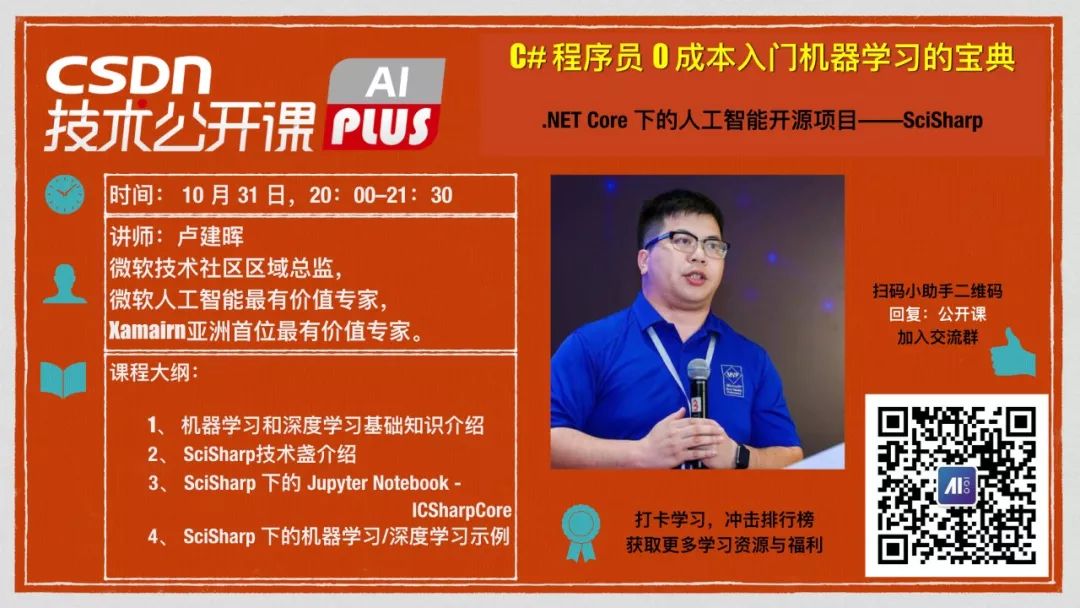
一文读懂对抗机器学习Universal adversarial perturbations | CSDN博文精选
作者 | Icoding_F2014来源 | CSDN博客本文提出一种 universal 对抗扰动,universal 是指同一个扰动加入到不同的图片中,能够使图片被分类模型误分类,而不管图片到底是什么。示意图:形式化的定义:对于d维数据分布 μ&…
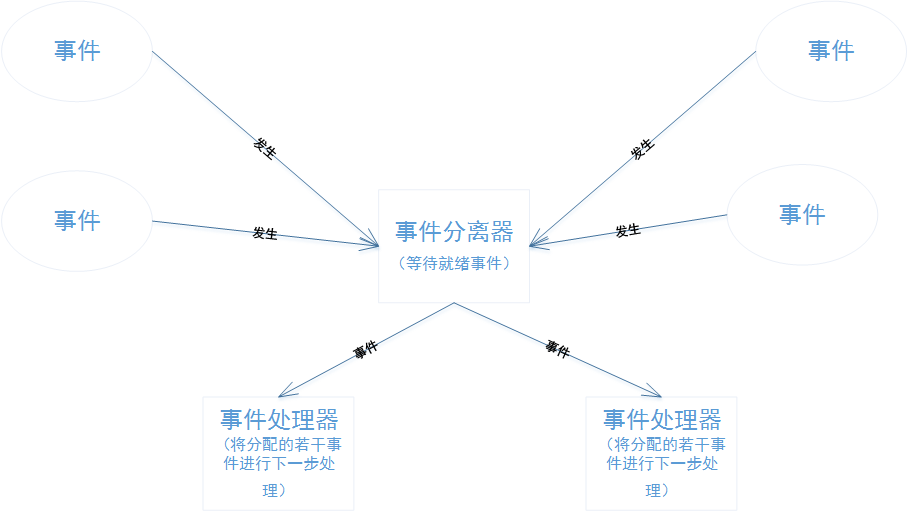
Reactor模式与Proactor模式
博主一脚刚踏进分布式的大门(看《分布式Java应用》,如果大家有啥推荐的书欢迎留言~),发现书中对NIO采用的Reactor模式、AIO采用的Proactor模式一笔带过,好奇心趋势我找了一下文章,发现两篇挺不错的文章&…
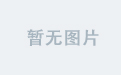
linux下使profile和.bash_profile立即生效的方法
使profile生效的方法1.source /etc/profile使用.bash_profile生效的方法1 . .bash_profile2 source .bash_profile3 exec bash --login转载于:https://blog.51cto.com/shine20/1436473
吴恩达老师深度学习视频课笔记:多隐含层神经网络公式推导(二分类)
多隐含层神经网络的推导步骤非常类似于单隐含层神经网络的步骤,只不过是多重复几遍。关于单隐含层神经网络公式的推导可以参考: http://blog.csdn.net/fengbingchun/article/details/79370310 逻辑回归是一个浅层模型(shadow model),或称单层…
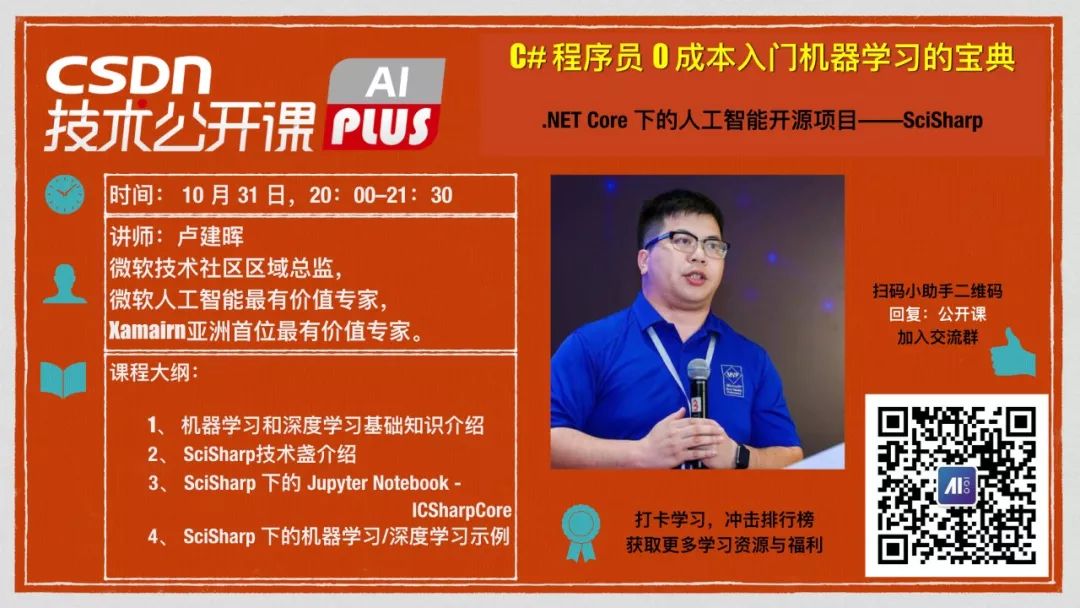
Python中的元编程:一个关于修饰器和元类的简单教程
作者 | Saurabh Kukade译者 | 刘畅出品 | AI科技大本营(ID:rgznai100)最近,作者遇到一个非常有趣的概念,它就是用 Python 进行元编程。我想在本文中分享我对该主题的见解。作者希望它可以帮助解决这个问题,因为很多人说…
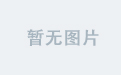
获取用户电脑的上网IP地址
在项目中经常要获取用户的上网的IP地址,如何获取用户的IP地址,方法很多,现在介绍以下2种。 /// <summary> /// 获取本机在局域网的IP地址 /// </summary> /// <returns></returns> …
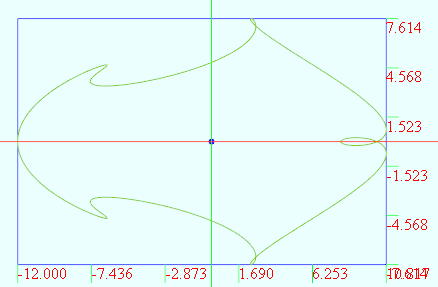
数学图形(1.40)T_parameter
不记得在哪搞了个数学公式生成的图形. vertices 1000t from 0 to (2*PI) r 2.0 x r*(5*cos(t) - cos(6*t)) y r*(3*sin(t) - sin(4*t)) 给线加上一维变量的变化,使之变成面: vertices D1:360 D2:21u from 0 to (2*PI) D1 v from 0 to 20 D2x (v2)*cos(u) - cos((v3)*u…
K-均值聚类(K-Means) C++代码实现
K-均值聚类(K-Means)简介可以参考: http://blog.csdn.net/fengbingchun/article/details/79276668 以下是K-Means的C实现,code参考OpenCV 3.3中的cv::kmeans函数,均值点初始化的方法仅支持KMEANS_RANDOM_CENTERS。以下是从数据集MNIST中提取…
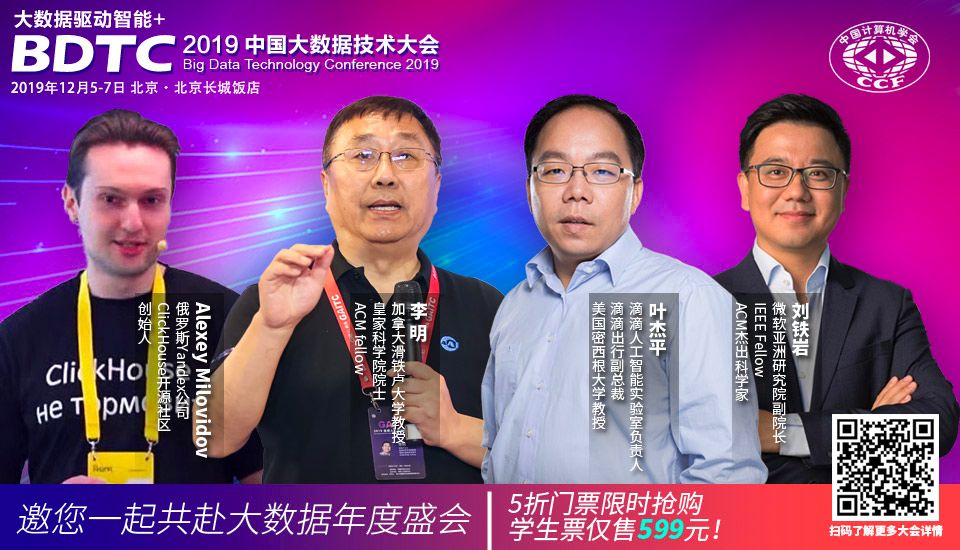
让学生网络相互学习,为什么深度相互学习优于传统蒸馏模型?| 论文精读
作者 | Ying Zhang,Tao Xiang等译者 | 李杰出品 | AI科技大本营(ID:rgznai100)蒸馏模型是一种将知识从教师网络(teacher)传递到学生网络(student)的有效且广泛使用的技术。通常来说,…

mac apache 配置
mac系统自带apache这无疑给广大的开发朋友提供了便利,接下来是针对其中的一些说明 一、自带apache相关命令 1. sudo apachectl start 启动服务,需要权限,就是你计算机的password 2. sudo apachectl stop 终止服务 ####3. sudo apachectl rest…
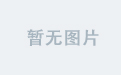
jQuery学习---------认识事件处理
3种事件模型:原始事件模型DOM事件模型IE事件模型原始事件模型(0级事件模型)1、事件处理程序被定义为函数实例,然后绑定到DOM元素事件对象上,实现事件的注册。例子:var btn document.getElementsByTagName(…
C++中的虚函数表介绍
在C语言中,当我们使用基类的引用或指针调用一个虚成员函数时会执行动态绑定。因为我们直到运行时才能知道到底调用了哪个版本的虚函数,所以所有虚函数都必须有定义。通常情况下,如果我们不使用某个函数,则无须为该函数提供定义。但是我们必须…
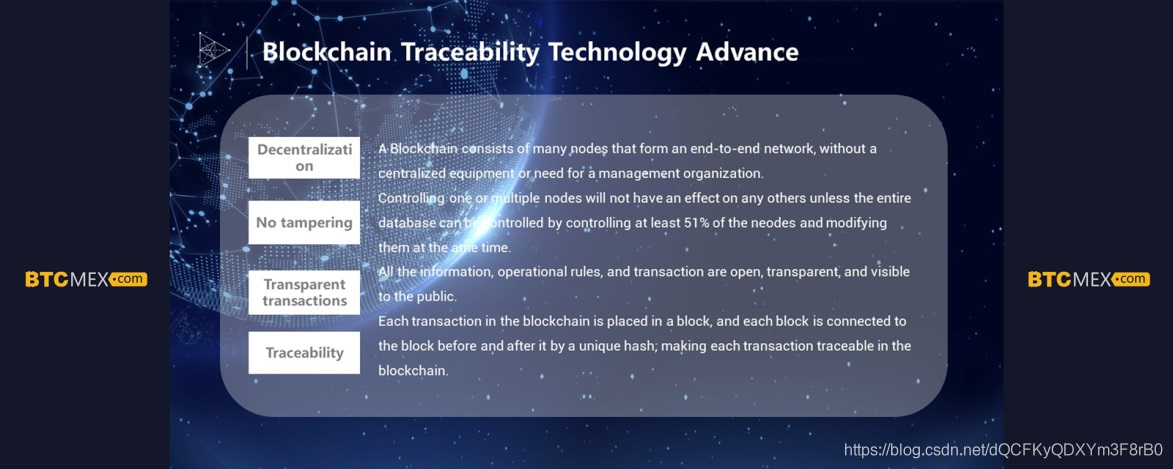
AI如何赋能金融行业?百度、图灵深视等同台分享技术实践
近日,由BTCMEX举办的金融技术创新研讨会在北京举办。BTCMEX投资人李笑来,AI技术公司TuringPass、百度、美国Apache基金会项目Pulsar、区块链安全公司SlowMist等相关专家参加了此次会议,共同探讨了金融技术在创新方面的现状。 图灵深视副总裁许…
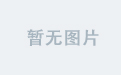
【Win32 API学习]打开可执行文件
在MFC中打开其他可执行文件常用到的方法有:WinExec、ShellExecute、CreatProcess。 1.WinExec WinExec 主要运行EXE文件,用法简单,只有两个参数,前一个指定命令路径,后一个指定窗口显示方式: UINT WinExec(…
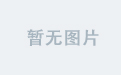
支付宝接口使用文档说明 支付宝异步通知
支付宝接口使用文档说明 支付宝异步通知(notify_url)与return_url. 现支付宝的通知有两类。 A服务器通知,对应的参数为notify_url,支付宝通知使用POST方式 B页面跳转通知,对应的参数为return_url,支付宝通知使用GET方式 ÿ…
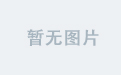
完全隐藏Master Page Site Actions菜单只有管理员才可以看见
1. 在Master Page Head 增加下面的Style <style type"text/css"> .ms-cui-tt{visibility:hidden;} </style> 2. 增加SPSecurityTrimmedControl <SharePoint:SPRibbonPeripheralContent runat"server" Location"TabRowLeft&qu…
深度学习中的随机梯度下降(SGD)简介
随机梯度下降(Stochastic Gradient Descent, SGD)是梯度下降算法的一个扩展。机器学习中反复出现的一个问题是好的泛化需要大的训练集,但大的训练集的计算代价也更大。机器学习算法中的代价函数通常可以分解成每个样本的代价函数的总和。随着训练集规模增长为数十亿…
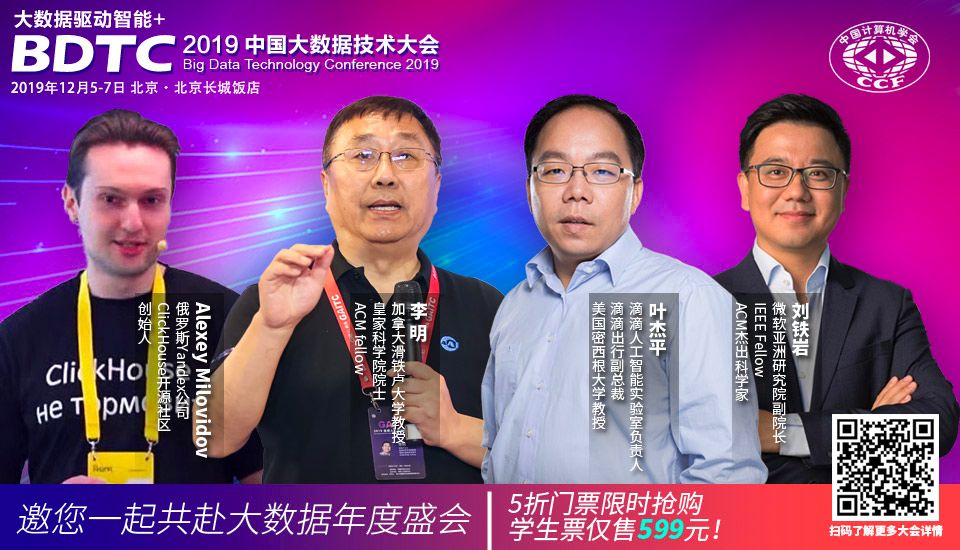
推荐系统中的前沿技术研究与落地:深度学习、AutoML与强化学习 | AI ProCon 2019...
整理 | 夕颜出品 | AI科技大本营(ID:rgznai100)个性化推荐算法滥觞于互联网的急速发展,随着国内外互联网公司,如 Netflix 在电影领域,亚马逊、淘宝、京东等在电商领域,今日头条在内容领域的采用和推动&…

运维日志管理系统
因公司数据安全和分析的需要,故调研了一下 GlusterFS lagstash elasticsearch kibana 3 redis 整合在一起的日志管理应用:安装,配置过程,使用情况等续一,glusterfs分布式文件系统部署:说明…
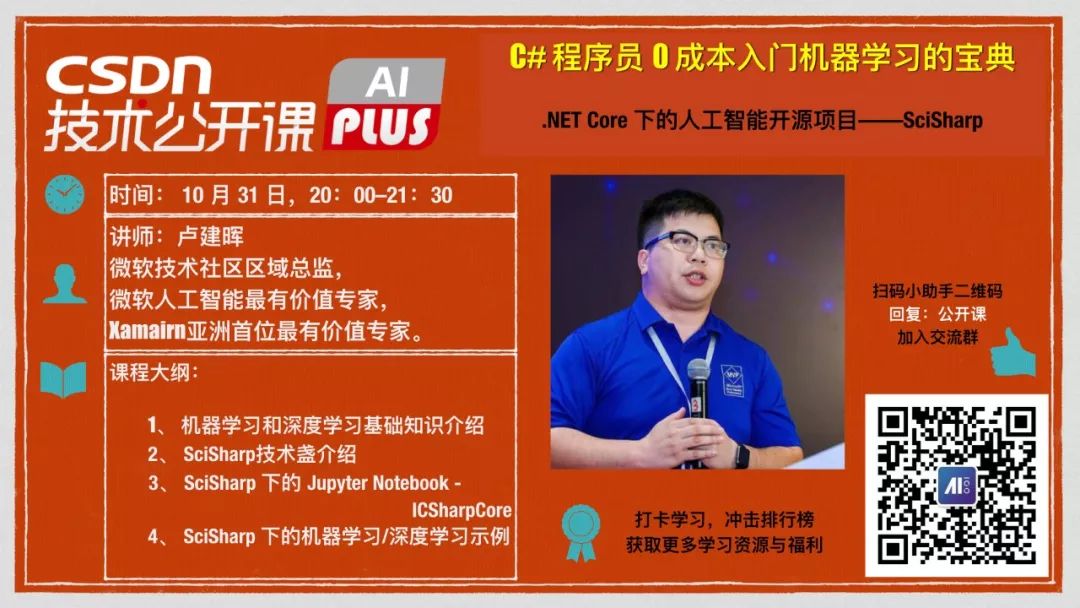
NLP学习思维导图,非常的全面和清晰
作者 | Tae Hwan Jung & Kyung Hee编译 | ronghuaiyang【导读】Github上有人整理了NLP的学习路线图(思维导图),非常的全面和清晰,分享给大家。先奉上GitHub地址:https://github.com/graykode/nlp-roadmapnlp-roadm…
Go在windows10 64位上安装过程
1. 从 https://golang.org/dl/ 下载最新的发布版本go1.10即go1.10.windows-amd64.msi; 2. 双击go1.10.windows-amd64.msi ,使用默认选项,默认会安装到C:\Go目录下; 3. 将C:\Go\bin目录添加到系统环境变量中(默认已自动添加),此目录下有go.exe…
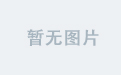
Windows SharePoint Services 3.0 应用程序模板
微软发布的一些WSS模板,看了一下,跟以前看到的模板好像不同模板分两类,一类是站点管理模板,一类是服务器管理模板站点管理模板:董事会、业务绩效报告、政府机构案例管理、课堂管理、临床试验启动和管理、竞争性分析站点…
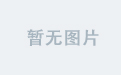
HAProxy+Keepalived高可用负载均衡配置
一、系统环境:系统版本:CentOS5.5 x86_64master_ip:172.20.27.40backup_ip:172.20.27.50 vip:172.20.27.200web_1: 172.20.27.90web_2:172.20.27.100二、haproxy安装:1.首先172.20.27.40安装上安装:1.1安装 tar zxvf haproxy-1.3.…
Go在Ubuntu 14.04 64位上的安装过程
1. 从 https://golang.org/dl/ 或 https://studygolang.com/dl 下载最新的发布版本go1.10即go1.10.linux-amd64.tar.gz; 2. 将下载的tar包解压缩到/usr/local目录下,执行以下命令,结果如下: $ sudo tar -C /usr/local -xzf go1.…
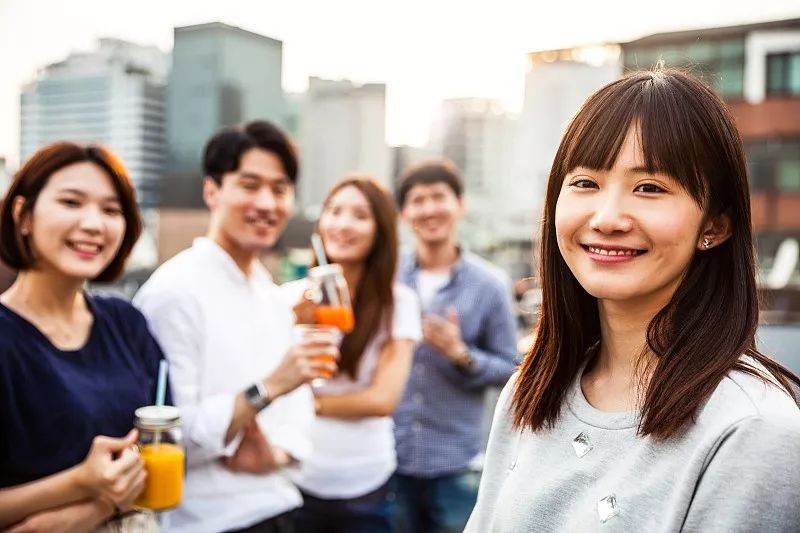
毕业就拿阿里offer,你和他比差在哪?
我在大学的时候,真的遇到一个神人,叫他小马吧。超前学习。1024,是程序员的节日,恰逢CSDN的20周年,我们准备为你做件大事!我们与AI博士唐宇迪、畅销书作家、北大硕士阿甘等4位老师,共同为大家带来…
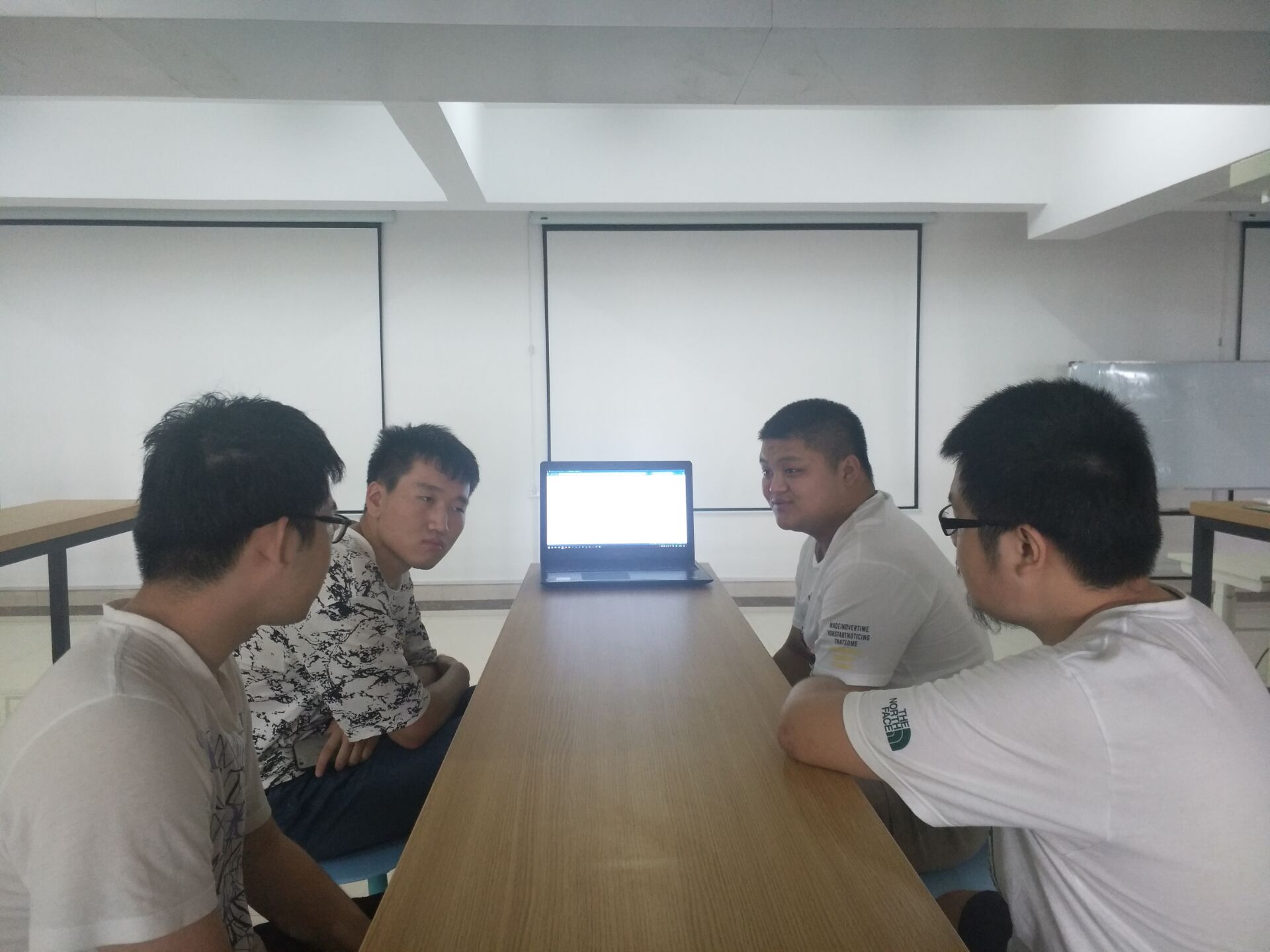
04号团队-团队任务5:项目总结会
1.团队信息 团队序号:04 开发项目:北软毕设管理系统 整理人:丛云聪 学号:2017035107185 在团队中的职务:项目经理兼产品经理 2.代码仓库地址 主仓库:https://gitee.com/The_Old_Cousin/StuInfoManage…