经典网络LeNet-5介绍及代码测试(Caffe, MNIST, C++)
LeNet-5:包含7个层(layer),如下图所示:输入层没有计算在内,输入图像大小为32*32*1,是针对灰度图进行训练和预测的。论文名字为” Gradient-Based Learning Applied to Document Recognition”,可以直接从http://yann.lecun.com/exdb/publis/pdf/lecun-01a.pdf 下载原始论文。
第一层是卷积层,使用6个5*5的filter,stride为1,padding为0,输出结果为28*28*6,6个feature maps,训练参数(5*5*1)*6+6=156(weights + bias);
第二层进行平均池化操作,filter为2*2,stride为2,padding为0,输出结果为14*14*6,6个feature maps,训练参数1*6+6=12(coefficient + bias);
第三层是卷积层,使用16个5*5的filter,stride为1,padding为0,输出结果为10*10*16,16个feature maps, 训练参数(按照论文给的连接方式) (5*5*3+1)*6 + (5*5*4+1)*6+(5*5*4+1)*3+(5*5*6+1)*1 = 1516(weights + bias);
第四层又是平均池化层,filter为2*2,stride为2,padding为0,输出结果为5*5*16,16个feature maps,训练参数1*16+16=32(coefficient + bias);
第五层是卷积层,使用120个5*5的fiter,stride为1,输出结果为1*1*120,120个feature maps,训练参数(5*5*6)*120+120=48120(weights + bias);
第六层是一个全连接层,有84个神经元,训练参数120*84+84=10164(weights + bias);此layer使用的激活函数为tanh。
第七层得到最后的输出预测y’的值,y’有10个可能的值,对应识别0--9这10个数字,在现在的版本中,使用softmax函数输出10种分类结果,即第七层为softmax。
输入图像size为32*32比训练数据集图像size为28*28大的原因:期望诸如笔划终点(stroke end-points)或角点(corner)这些潜在的特征(potential distinctive feature)能够出现在最高层特征检测器(highest-level feature detectors)的感受野的中心。
没有把layer2中的每个feature map连接到layer3中的每个feature map原因:(1). 不完全的连接机制将连接的数量保持在合理的范围内;(2). 更重要的,它强制破坏了网络的对称性。因为不同的feature maps来自不同的输入,所以不同的feature maps被强制提取不同的features.(The main reason is to break the symmetry in the network and keeps the number of connections within reasonable bounds.)
LeNet-5中的5是指5个隐藏层即卷积层、池化层、卷积层、池化层、卷积层。
不同的filter可以提取不同的特征,如边沿、线性、角等特征。
关于卷积神经网络的基础介绍可参考之前的blog:
https://blog.csdn.net/fengbingchun/article/details/50529500
https://blog.csdn.net/fengbingchun/article/details/80262495
https://blog.csdn.net/fengbingchun/article/details/68065338
https://blog.csdn.net/fengbingchun/article/details/69001433
以下是参考Caffe中的测试代码对LeNet-5网络进行测试的代码,与论文中的不同处包括:
(1). 论文中要求输入层图像大小为32*32,这里为28*28;
(2). 论文中第一层卷积层输出是6个feature maps,这里是20个feature maps;
(3). 论文中池化层取均值,而这里取最大值;
(4). 论文中第三层卷积层输出是16个feature maps,这里是50个feature maps,而且这里第二层的feature map是连接到第三层的每个feature map的;
(5). 论文中第五层是卷积层,这里是全连接层并输出500个神经元,激活函数采用ReLU;
(6). 论文中第七层是RBF(Euclidean Radial Basic Function),这里采用Softmax。
以下是测试代码(lenet-5.cpp):
#include "funset.hpp"
#include "common.hpp"int lenet_5_mnist_train()
{
#ifdef CPU_ONLYcaffe::Caffe::set_mode(caffe::Caffe::CPU);
#elsecaffe::Caffe::set_mode(caffe::Caffe::GPU);
#endif#ifdef _MSC_VERconst std::string filename{ "E:/GitCode/Caffe_Test/test_data/Net/lenet-5_mnist_windows_solver.prototxt" };
#elseconst std::string filename{ "test_data/Net/lenet-5_mnist_linux_solver.prototxt" };
#endifcaffe::SolverParameter solver_param;if (!caffe::ReadProtoFromTextFile(filename.c_str(), &solver_param)) {fprintf(stderr, "parse solver.prototxt fail\n");return -1;}mnist_convert(); // convert MNIST to LMDBcaffe::SGDSolver<float> solver(solver_param);solver.Solve();fprintf(stdout, "train finish\n");return 0;
}int lenet_5_mnist_test()
{
#ifdef CPU_ONLYcaffe::Caffe::set_mode(caffe::Caffe::CPU);
#elsecaffe::Caffe::set_mode(caffe::Caffe::GPU);
#endif#ifdef _MSC_VERconst std::string param_file{ "E:/GitCode/Caffe_Test/test_data/Net/lenet-5_mnist_windows_test.prototxt" };const std::string trained_filename{ "E:/GitCode/Caffe_Test/test_data/Net/lenet-5_mnist_iter_10000.caffemodel" };const std::string image_path{ "E:/GitCode/Caffe_Test/test_data/images/handwritten_digits/" };
#elseconst std::string param_file{ "test_data/Net/lenet-5_mnist_linux_test.prototxt" };const std::string trained_filename{ "test_data/Net/lenet-5_mnist_iter_10000.caffemodel" };const std::string image_path{ "test_data/images/handwritten_digits/" };
#endifcaffe::Net<float> caffe_net(param_file, caffe::TEST);caffe_net.CopyTrainedLayersFrom(trained_filename);const boost::shared_ptr<caffe::Blob<float> > blob_data_layer = caffe_net.blob_by_name("data");int image_channel_data_layer = blob_data_layer->channels();int image_height_data_layer = blob_data_layer->height();int image_width_data_layer = blob_data_layer->width();const std::vector<caffe::Blob<float>*> output_blobs = caffe_net.output_blobs();int require_blob_index{ -1 };const int digit_category_num{ 10 };for (int i = 0; i < output_blobs.size(); ++i) {if (output_blobs[i]->count() == digit_category_num)require_blob_index = i;}if (require_blob_index == -1) {fprintf(stderr, "ouput blob don't match\n");return -1;}std::vector<int> target{ 0, 1, 2, 3, 4, 5, 6, 7, 8, 9 };std::vector<int> result;for (auto num : target) {std::string str = std::to_string(num);str += ".png";str = image_path + str;cv::Mat mat = cv::imread(str.c_str(), 1);if (!mat.data) {fprintf(stderr, "load image error: %s\n", str.c_str());return -1;}if (image_channel_data_layer == 1)cv::cvtColor(mat, mat, CV_BGR2GRAY);else if (image_channel_data_layer == 4)cv::cvtColor(mat, mat, CV_BGR2BGRA);cv::resize(mat, mat, cv::Size(image_width_data_layer, image_height_data_layer));cv::bitwise_not(mat, mat);boost::shared_ptr<caffe::MemoryDataLayer<float> > memory_data_layer =boost::static_pointer_cast<caffe::MemoryDataLayer<float>>(caffe_net.layer_by_name("data"));mat.convertTo(mat, CV_32FC1, 0.00390625);float dummy_label[1] {0};memory_data_layer->Reset((float*)(mat.data), dummy_label, 1);float loss{ 0.0 };const std::vector<caffe::Blob<float>*>& results = caffe_net.ForwardPrefilled(&loss);const float* output = results[require_blob_index]->cpu_data();float tmp{ -1 };int pos{ -1 };for (int j = 0; j < 10; j++) {//fprintf(stdout, "Probability to be Number %d is: %.3f\n", j, output[j]);if (tmp < output[j]) {pos = j;tmp = output[j];}}result.push_back(pos);}for (auto i = 0; i < 10; i++)fprintf(stdout, "actual digit is: %d, result digit is: %d\n", target[i], result[i]);fprintf(stdout, "predict finish\n");return 0;
}
solver.prototxt文件内容如下:
# solver.prototxt是一个配置文件用来告知Caffe怎样对网络进行训练
# 其文件内的各字段名需要在caffe.proto的message SolverParameter中存在,否则会解析不成功net: "test_data/Net/lenet-5_mnist_linux_train.prototxt" # 训练网络文件名
test_iter: 100 # test_iter * test_batch_size = 测试图像总数量
test_interval: 500 # 指定执行多少次训练网络执行一次测试网络
base_lr: 0.01 # 学习率
lr_policy: "inv" # 学习策略, return base_lr * (1 + gamma * iter) ^ (- power)
momentum: 0.9 # 动量
weight_decay: 0.0005 # 权值衰减
gamma: 0.0001 # 学习率计算参数
power: 0.75 # 学习率计算参数
display: 100 # 指定训练多少次在屏幕上显示一次结果信息,如loss值等
max_iter: 10000 # 最多训练次数
snapshot: 5000 # 执行多少次训练保存一次中间结果
snapshot_prefix: "test_data/Net/lenet-5_mnist" # 结果保存位置前缀
solver_type: SGD # 随机梯度下降
train时的prototxt文件内容如下:
name: "LeNet-5"layer {name: "mnist"type: "Data"top: "data"top: "label"include {phase: TRAIN}transform_param {scale: 0.00390625}data_param {source: "test_data/MNIST/train"batch_size: 64backend: LMDB}
}
layer {name: "mnist"type: "Data"top: "data"top: "label"include {phase: TEST}transform_param {scale: 0.00390625}data_param {source: "test_data/MNIST/test"batch_size: 100backend: LMDB}
}
layer {name: "conv1"type: "Convolution"bottom: "data"top: "conv1"param {lr_mult: 1}param {lr_mult: 2}convolution_param {num_output: 20kernel_size: 5stride: 1weight_filler {type: "xavier"}bias_filler {type: "constant"}}
}
layer {name: "pool1"type: "Pooling"bottom: "conv1"top: "pool1"pooling_param {pool: MAXkernel_size: 2stride: 2}
}
layer {name: "conv2"type: "Convolution"bottom: "pool1"top: "conv2"param {lr_mult: 1}param {lr_mult: 2}convolution_param {num_output: 50kernel_size: 5stride: 1weight_filler {type: "xavier"}bias_filler {type: "constant"}}
}
layer {name: "pool2"type: "Pooling"bottom: "conv2"top: "pool2"pooling_param {pool: MAXkernel_size: 2stride: 2}
}
layer {name: "ip1"type: "InnerProduct"bottom: "pool2"top: "ip1"param {lr_mult: 1}param {lr_mult: 2}inner_product_param {num_output: 500weight_filler {type: "xavier"}bias_filler {type: "constant"}}
}
layer {name: "relu1"type: "ReLU"bottom: "ip1"top: "ip1"
}
layer {name: "ip2"type: "InnerProduct"bottom: "ip1"top: "ip2"param {lr_mult: 1}param {lr_mult: 2}inner_product_param {num_output: 10weight_filler {type: "xavier"}bias_filler {type: "constant"}}
}
layer {name: "accuracy"type: "Accuracy"bottom: "ip2"bottom: "label"top: "accuracy"include {phase: TEST}
}
layer {name: "loss"type: "SoftmaxWithLoss"bottom: "ip2"bottom: "label"top: "loss"
}
train.prototxt可视化结果如下:
test时的test.prototxt文件内容如下:
name: "LeNet-5"layer {name: "data"type: "MemoryData"top: "data" #top: "label"memory_data_param {batch_size: 1channels: 1height: 28width: 28}transform_param {scale: 0.00390625}
}
layer {name: "conv1"type: "Convolution"bottom: "data"top: "conv1"param {lr_mult: 1}param {lr_mult: 2}convolution_param {num_output: 20kernel_size: 5stride: 1weight_filler {type: "xavier"}bias_filler {type: "constant"}}
}
layer {name: "pool1"type: "Pooling"bottom: "conv1"top: "pool1"pooling_param {pool: MAXkernel_size: 2stride: 2}
}
layer {name: "conv2"type: "Convolution"bottom: "pool1"top: "conv2"param {lr_mult: 1}param {lr_mult: 2}convolution_param {num_output: 50kernel_size: 5stride: 1weight_filler {type: "xavier"}bias_filler {type: "constant"}}
}
layer {name: "pool2"type: "Pooling"bottom: "conv2"top: "pool2"pooling_param {pool: MAXkernel_size: 2stride: 2}
}
layer {name: "ip1"type: "InnerProduct"bottom: "pool2"top: "ip1"param {lr_mult: 1}param {lr_mult: 2}inner_product_param {num_output: 500weight_filler {type: "xavier"}bias_filler {type: "constant"}}
}
layer {name: "relu1"type: "ReLU"bottom: "ip1"top: "ip1"
}
layer {name: "ip2"type: "InnerProduct"bottom: "ip1"top: "ip2"param {lr_mult: 1}param {lr_mult: 2}inner_product_param {num_output: 10weight_filler {type: "xavier"}bias_filler {type: "constant"}}
}
layer {name: "prob"type: "Softmax"bottom: "ip2"top: "prob"
}
test.prototxt可视化结果如下:
train时测试代码执行结果如下:
test是测试代码执行结果如下:
GitHub:https://github.com/fengbingchun/Caffe_Test
相关文章:
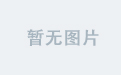
根据经纬度获取用户当前位置信息
根据上篇文章获取的经纬度获取用户当前的位置信息 //获取用户所在位置信息ADDRESS func getUserAddress() { let latitude : CLLocationDegrees LATITUDES! let longitude : CLLocationDegrees LONGITUDES! print("latitude:\(latitude)") print("longitude…
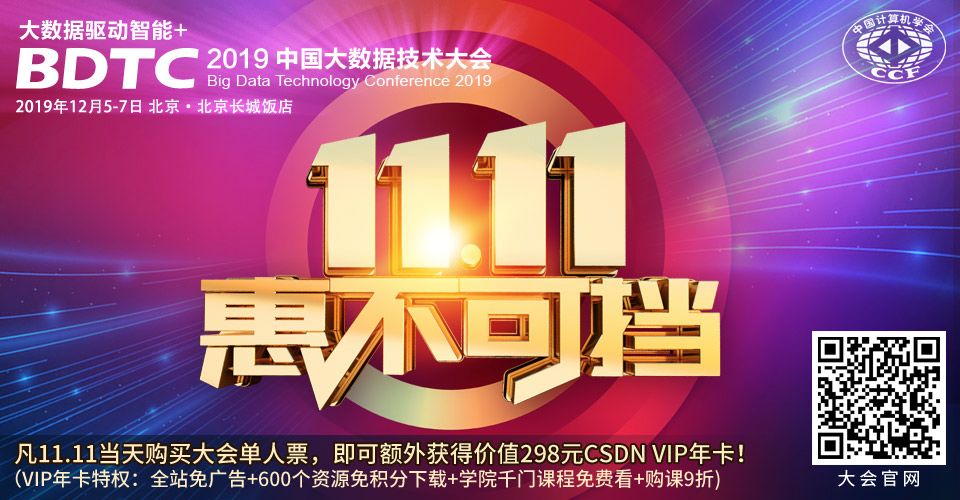
刷了几千道算法题,我私藏的刷题网站都在这里了
作者 | Rocky0429 来源 | Python空间(ID: Devtogether)遥想当年,机缘巧合入了 ACM 的坑,周边巨擘林立,从此过上了"天天被虐似死狗"的生活...然而我是谁,我可是死狗中的战斗鸡,智力不够…
js实现点击li标签弹出其索引值
据说这是一道笔试题,以下是代码,没什么要文字叙述的,就是点击哪个<li>弹出哪个<li>的索引值即可: <html> <head> <style> li{width:50px;height:30px;margin:5px;float:left;text-align: center;li…
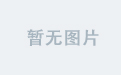
定时器开启和关闭
写程序时遇见了定时器,需要写入数据库用户的经纬 ,还要读取,写好之后发现很费电 总结原因: 1:地图定位耗电(这个根据程序要求,不能关闭,需要实时定位,很无奈ÿ…
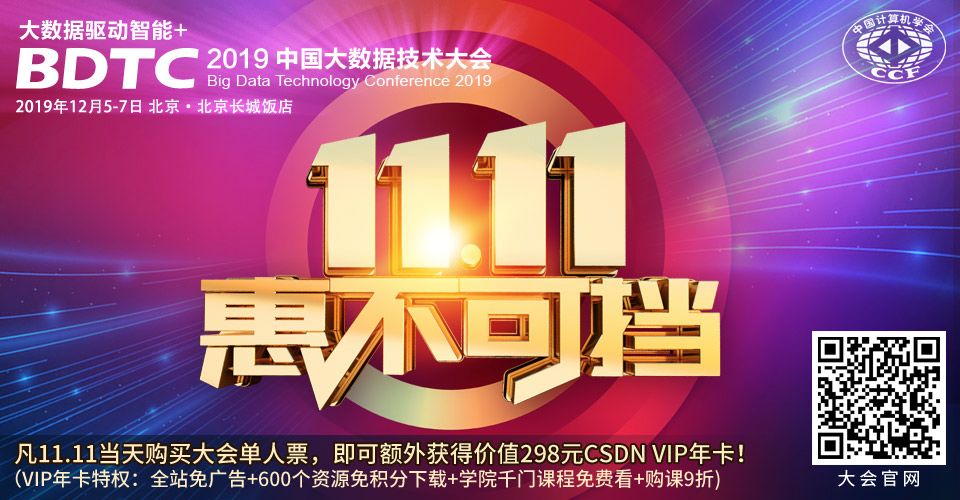
一览群智胡健:在中国完全照搬Palantir模式,这不现实
作者 | Just出品 | AI科技大本营(ID:rgznai100)神秘的硅谷大数据挖掘公司 Palantir 是国内众多创业公司看齐的标杆,其业务是为政府和金融领域的大客户提供数据分析服务,帮助客户作出判断,甚至“预知未来”,…
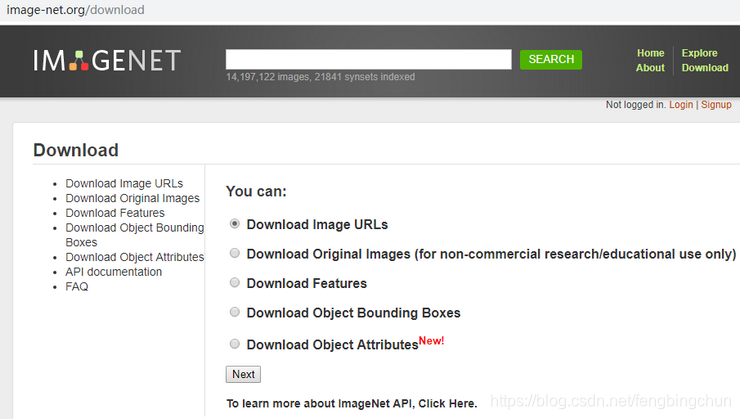
ImageNet图像数据集介绍
ImageNet图像数据集始于2009年,当时李飞飞教授等在CVPR2009上发表了一篇名为《ImageNet: A Large-Scale Hierarchical Image Database》的论文,之后就是基于ImageNet数据集的7届ImageNet挑战赛(2010年开始),2017年后,ImageNet由Ka…
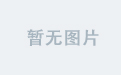
cocos2dx 场景的切换
我们知道cocos2dx中可以由多个场景组成,那么我是如何来切换场景的呢首先我们先新建一个新的场景类,我推荐的方式是,在你工程的目录中找到一个classes的文件夹,里面有AppDelegate.cpp和AppDelegate.h还有HelloWorldScene.cpp和Hell…
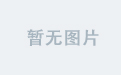
IOS 后台挂起程序 当程序到后台后,继续完成定位任务
// 当应用程序掉到后台时,执行该方法 - (void)applicationDidEnterBackground:(UIApplication *)application { } 当一个 iOS 应用被送到后台,它的主线程会被暂停。你用 NSThread 的 detachNewThreadSelector:toTar get:withObject:类方法创建的线程也被挂起了。 我…
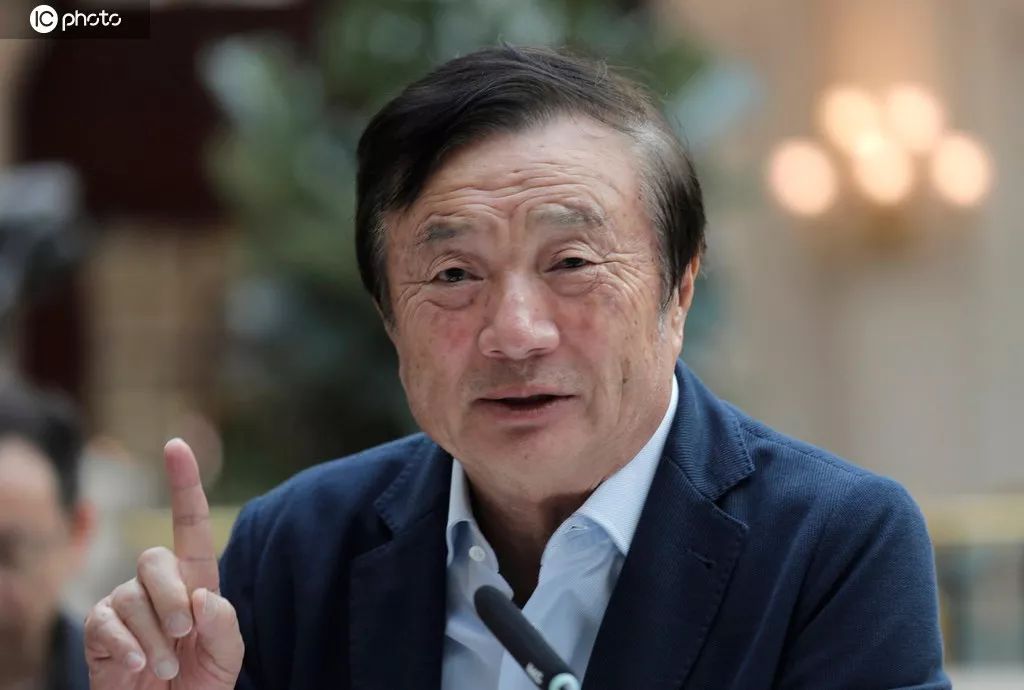
任正非:华为5G是瞎猫碰死老鼠
喜欢话糙理不糙的任正非,又飙金句。11月6日,在和彭博社记者对话时,谈到华为5G,他说:“回顾这个过程,我们也没有什么必胜的信心,有时候也是瞎猫碰上了死老鼠,刚好碰上世界是这个需求。…
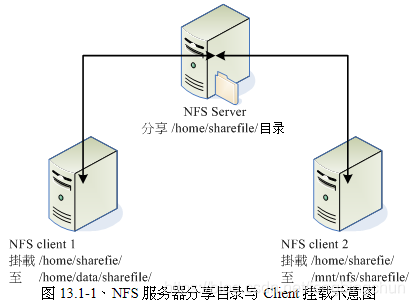
网络文件系统(NFS)简介
网络文件系统(Network File System, NFS)是一种分布式文件系统协议,最初由Sun Microsystems公司开发,并于1984年发布。其功能旨在允许客户端主机可以像访问本地存储一样通过网络访问服务器端文件。NFS和其他许多协议一样,是基于开放网络运算远…
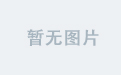
JAVA Static方法与单例模式的理解
最近用sonar测评代码质量的时候,发现一个问题,工程中一些util类,以前写的static方法都提示最好用单例的方式进行改正。为此,我仔细想了想,发现还是很有道理的。这里谈谈我个人对static方法与单例模式的理解。所谓单例模…
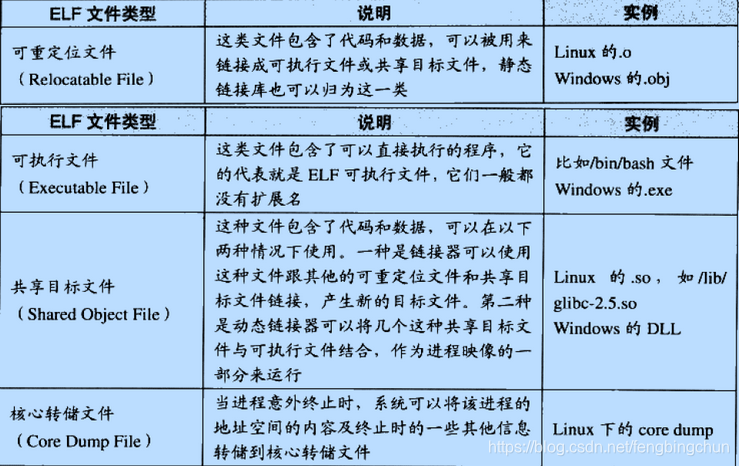
程序员的自我修养--链接、装载与库笔记:目标文件里有什么
编译器编译源代码后生成的文件叫做目标文件。目标文件从结构上讲,它是已经编译后的可执行文件格式,只是还没有经过链接的过程,其中可能有些符号或有些地址还没有被调整。其实它本身就是按照可执行文件格式存储的,只是跟真正的可执…
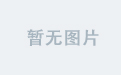
swift 中拨电话的实现
//MARK:_一键报警设置//MARK: - 弹出视图func createView() {var alertView : UIAlertView?alertView UIAlertView(title: "110", message: "", delegate: self, cancelButtonTitle: "取消", otherButtonTitles: "呼叫")alertView?…
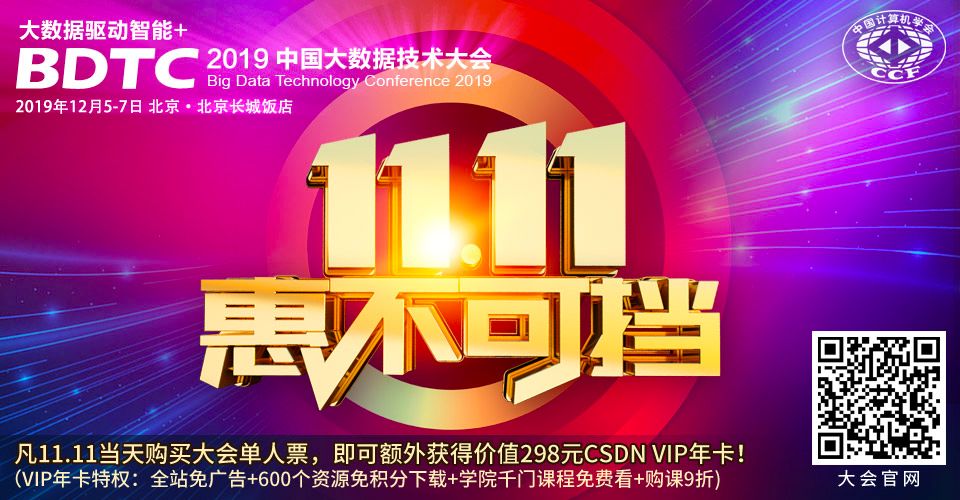
T5,一个探索迁移学习边界的模型
作者 | Ajit Rajasekharan译者 | 夕颜出品 | AI科技大本营(ID:rgznai100)【导读】10月,Google 在《Exploring the Limits of Transfer Learning with a Unified Text-to-Text Transformer》这篇论文中提出了一个最新的预训练模型 T5ÿ…
【Chat】实验 -- 实现 C/C++下TCP, 服务器/客户端 多人聊天室
本次实验利用TCP/IP, 语言环境为 C/C 利用套接字Socket编程,以及线程处理, 实现Server/CLient 之间多人的聊天系统的基本功能。 结果大致如: 下面贴上代码(参考参考...) Server 部分: 1 /* TCPdtd.cpp - main, TCPdayt…
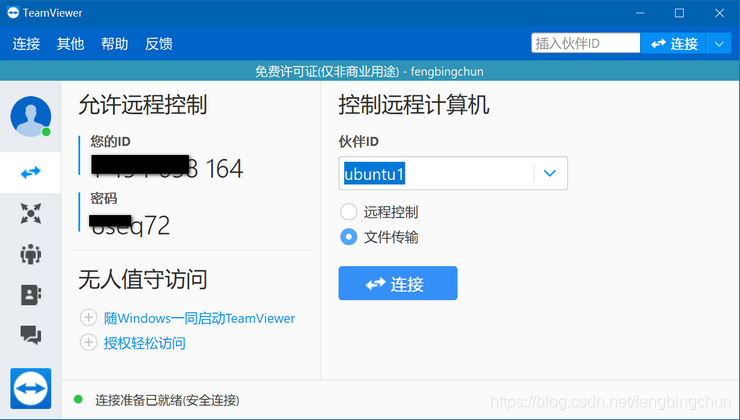
TeamViewer介绍:远程控制计算机
TeamViewer是一个可以远程控制计算机的程序,它也可以进行远程文件传输。TeamViewer支持的平台比较多,如Windows, Mac, Linux, ChromeOs, Android, iOS等,最新发布版本为14.x,它有个人免费和商业付费两种。只要对方告诉你他的TeamV…
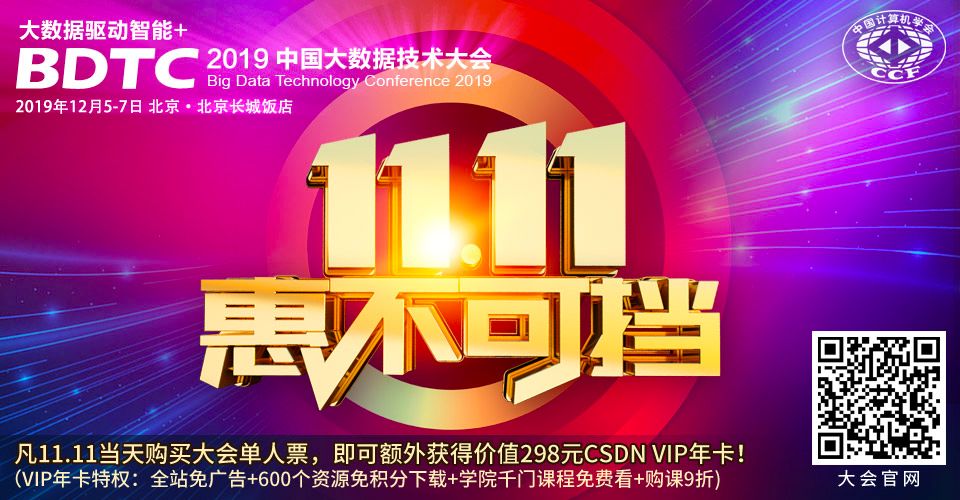
PyTorch攻势凶猛,程序员正在抛弃TensorFlow?
来源 | The Gradient译者 | 夕颜出品 | AI科技大本营(ID:rgznai100)自 2012 年深度学习重新获得重视以来,许多机器学习框架便争相成为研究人员和行业从业人员的新宠。从早期的学术成果 Caffe 和 Theano ,到背靠庞大工业支持的 PyT…

swift 错误集合 ------持续更新中
从今天开始凡是在用swift中遇到的错误都会在本博客持续更新 便于自己学习和快速开发 2017.7.20 如果你的程序写的有进入后台的方法,例如我的博客中点击home进入后台持续定位的那篇文章,发信进入后台后定位没有按得定时器规定的时间走,这…
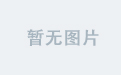
【转载】【贪心】各种覆盖问题
1、独立区间问题 在N个区间里找出最多的互不覆盖的区间 对结束点进行排序,然后从结束点最小的区间开始进行选择即可 2、覆盖区间问题 给一个大区间,再给出N个小区间,求出最少用多少个区间可以把大区间覆盖完 先选出开始的一个,然后…

使用Python3发送邮件测试代码
SMTP(Simple Mail Trasfer Protocol)即简单邮件传输协议,它是一组用于由源地址到目的地址传送邮件的规则,用它来控制信件的中转方式。Python3对SMTP的支持有smtplib和email两个模块,smtplib负责发送电子邮件, email负责组织邮件内…
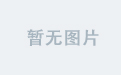
swift 通知中心 进入后台多久会通知用户关闭此功能
//添加本地通知 func addLocalNotification() { //定义本地通知对象 let notification : UILocalNotification UILocalNotification() //设置调用时间 notification.fireDate NSDate.init(timeIntervalSinceNow: 1800.0)//通知触发的时间,10s以后 notification.…
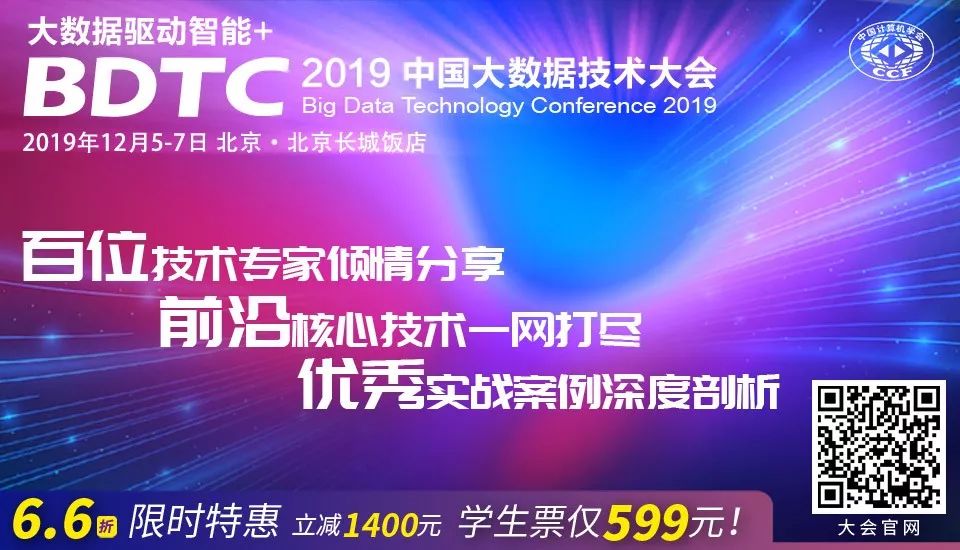
Python之父退休,C语言之父与世长辞,各大编程语言创始人现状盘点
作者 | 年素清 编辑 | 伍杏玲 来源 | 程序人生(ID:coder_life)从世界上第一台计算机(ENIAC) 于1946年2月在美国诞生至今的七十多年里,涌现出了许多优秀的计算机编程语言。程序员们在使用它们编写程序的时候,一定很好奇…
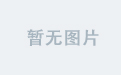
linux修正系统错误指令fsck和badblocks
fsck [-t文件系统][-ACay]装置名称-t 指定文件系统-A 扫描需要的装置-a 自动修复检查到有问题的扇区-y 与-a类似-C 在检查过程中,显示进度********************************************************** EXT2/EXT3额外选项功能:-f 强制检查-D 针对文件系…
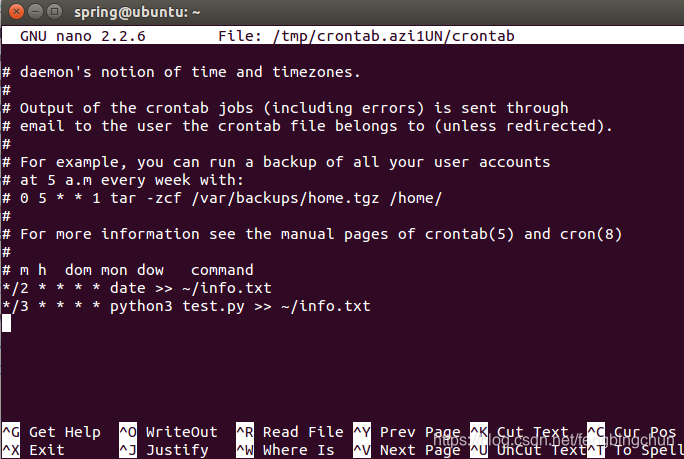
Ubuntu定时任务crontab命令介绍
通过Linux上的crontab命令,我们可以在规定的间隔时间执行指定的系统指令或脚本。时间间隔的单位可以是分钟、小时、日、月、周及以上的任意组合。 crontab默认在Ubuntu上是已经安装的,若未安装,则可执行以下命令进行安装: sudo …
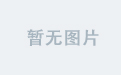
swift 进入后台或者点击home键是程序进入后台后,持续定位
进入后台的方法 import UIKit UIApplicationMain class AppDelegate: UIResponder, UIApplicationDelegate,CLLocationManagerDelegate { var locationManager : CLLocationManager? var window: UIWindow? var notificationDict NSDictionary() func applicationDidEnterBa…
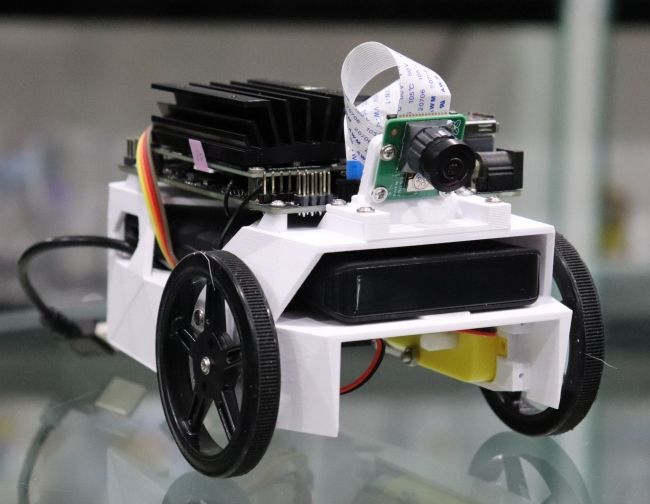
求助:我有一辆机器人小车,怎么让它跑起来,还会避障、目标跟踪、路径规划?...
也许,你曾见过能灵活地绕开障碍物的它在桌子边缘“疯狂试探”的它它是谁?没错,它就是是英伟达推出的一款入门级人工智能小车——Jetbot ,估计对机器人,尤其是对车械感兴趣的朋友们一定对它不陌生。组装完成后能够通过摄…
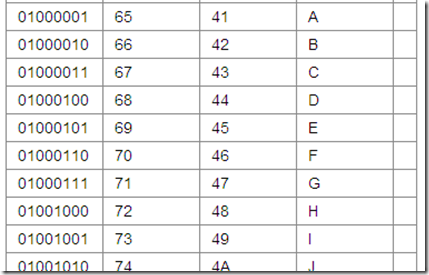
Python-常用字符串转换实例
当字符串是:\u4e2d\u56fd >>>s[\u4e2d\u56fd,\u6e05\u534e\u5927\u5b66]>>>strs[0].decode(unicode_escape) #.encode("EUC_KR")>>>print str 中国 当字符串是: >>>print unichr(19996) 东 ord()支持unicode&…

什么是静态UItableView
iOS开发UI篇—简单介绍静态单元格的使用 iOS开发UI篇—简单介绍静态单元格的使用 一、实现效果与说明 说明:观察上面的展示效果,可以发现整个界面是由一个tableview来展示的,上面的数据都是固定的,且几乎不会改变。 要完成上面的…

Python3中PyMongo使用举例
MongoDB是一个基于分布式文件存储的开源数据库,由C语言编写,与平台无关,旨在为WEB应用提供可扩展的高性能数据存储解决方案。MongoDB是一个介于关系数据库和非关系数据库之间的产品,是非关系数据库中功能最丰富,最像关…
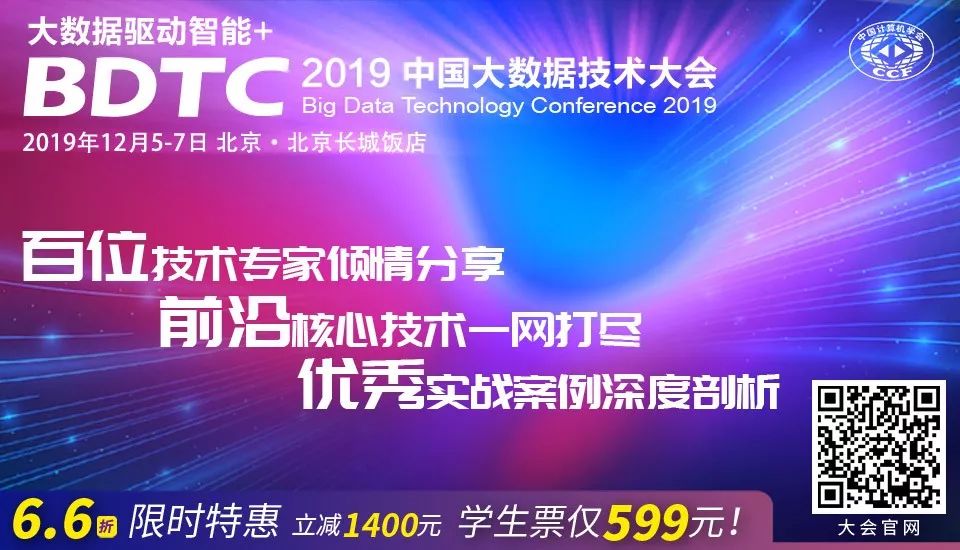
PyTorch踩过的12坑 | CSDN博文精选
作者 | hyk_1996 来源 | CSDN博客1. nn.Module.cuda() 和 Tensor.cuda() 的作用效果差异无论是对于模型还是数据,cuda()函数都能实现从CPU到GPU的内存迁移,但是他们的作用效果有所不同。对于nn.Module:model model.cuda() model.cuda() 上面两句能够达到…