tensorflow 2
import tensorflow as tf
import numpy as npdef test1():#create datax_data=np.random.rand(100).astype(np.float32)y_data=x_data*0.1+0.3#create tensorflow structureWeights=tf.Variable(tf.random_uniform([1],-1.0,1.0)) #一维,范围[-1,1]biases=tf.Variable(tf.zeros([1]))y=Weights*x_data+biasesloss=tf.reduce_mean(tf.square(y-y_data))#建立优化器,减小误差,提高参数准确度,每次迭代都会优化optimizer=tf.train.GradientDescentOptimizer(0.5) #学习效率<1train=optimizer.minimize(loss)#初始化变量init=tf.global_variables_initializer()with tf.Session() as sess:sess.run(init)#trainfor step in range(201):sess.run(train)if step%20==0:print(step,sess.run(Weights),sess.run(biases))def test2():node1 = tf.constant(3.0, dtype=tf.float32)node2 = tf.constant(4.0)# also tf.float32 implicitlyprint(node1, node2)sess = tf.Session()print(sess.run([node1, node2]))node3 = tf.add(node1, node2)print("node3:", node3)print("sess.run(node3):", sess.run(node3))a = tf.placeholder(tf.float32)b = tf.placeholder(tf.float32)adder_node = a + b # + provides a shortcut for tf.add(a, b)print(sess.run(adder_node, {a:3, b:4.5}))print(sess.run(adder_node, {a: [1,3], b: [2,4]}))add_and_triple = adder_node *3.print(sess.run(add_and_triple, {a:3, b:4.5}))def test3():W = tf.Variable([.3], dtype=tf.float32)b = tf.Variable([-.3], dtype=tf.float32)x = tf.placeholder(tf.float32)linear_model = W*x + b init = tf.global_variables_initializer()sess = tf.Session()sess.run(init)print(sess.run(linear_model, {x: [1,2,3,4]}))print(sess.run(linear_model, {x: [[1,2],[3,4]]}))y = tf.placeholder(tf.float32)squared_deltas = tf.square(linear_model - y)loss = tf.reduce_sum(squared_deltas)loss1 = tf.reduce_mean(squared_deltas)print(sess.run(linear_model, {x:[1,2,3,4]}))print(sess.run(squared_deltas, {x: [1,2,3,4], y: [0, -1, -2, -3]}))print(sess.run(loss, {x: [1,2,3,4], y: [0, -1, -2, -3]}))print(sess.run(loss/4, {x: [1,2,3,4], y: [0, -1, -2, -3]}))print(sess.run(loss1, {x: [1,2,3,4], y: [0, -1, -2, -3]}))def test4():b = tf.Variable([-.3], dtype=tf.float32)fixb = tf.assign(b, [1.])init = tf.global_variables_initializer()sess = tf.Session()sess.run(init)print(sess.run(fixb))def test5():import tensorflow as tfmnist = tf.keras.datasets.mnist(x_train, y_train),(x_test, y_test) = mnist.load_data()print(type(x_train))print(type(y_train))print(type(x_test))print(type(y_test))x_train, x_test = x_train / 255.0, x_test / 255.0model = tf.keras.models.Sequential([tf.keras.layers.Flatten(input_shape=(28, 28)),tf.keras.layers.Dense(512, activation=tf.nn.relu),tf.keras.layers.Dropout(0.2),tf.keras.layers.Dense(10, activation=tf.nn.softmax)])model.compile(optimizer='adam',loss='sparse_categorical_crossentropy',metrics=['accuracy'])model.fit(x_train, y_train, epochs=5)model.evaluate(x_test, y_test)def test6():# 给定type,tf大部分只能处理float32数据input1 = tf.placeholder(tf.float32)input2 = tf.placeholder(tf.float32)# Tensorflow 1.0 修改版# tf.mul---tf.multiply# tf.sub---tf.subtract# tf.neg---tf.negativeoutput = tf.multiply(input1, input2)with tf.Session() as sess:# placeholder在sess.run()的时候传入值print(sess.run(output, feed_dict={input1: [7.], input2: [2.]}))def add_layer(inputs,in_size,out_size,activation_function=None):#Weights是一个矩阵,[行,列]为[in_size,out_size]Weights=tf.Variable(tf.random_normal([in_size,out_size]))#正态分布#初始值推荐不为0,所以加上0.1,一行,out_size列biases=tf.Variable(tf.zeros([1,out_size])+0.1)#Weights*x+b的初始化的值,也就是未激活的值Wx_plus_b=tf.matmul(inputs,Weights)+biases#激活if activation_function is None:#激活函数为None,也就是线性函数outputs=Wx_plus_belse:outputs=activation_function(Wx_plus_b)return outputsdef test7():"""定义数据形式"""# (-1,1)之间,有300个单位,后面的是维度,x_data是有300行(300个例子)x_data=np.linspace(-1,1,300)[:,np.newaxis]# 加噪声,均值为0,方差为0.05,大小和x_data一样print(x_data.shape)noise=np.random.normal(0,0.05,x_data.shape)y_data=np.square(x_data)-0.5+noisexs=tf.placeholder(tf.float32,[None,1])ys=tf.placeholder(tf.float32,[None,1])"""建立网络"""#定义隐藏层,输入1个节点,输出10个节点l1=add_layer(xs,1,10,activation_function=tf.nn.relu)#定义输出层prediction=add_layer(l1,10,1,activation_function=None)"""预测"""#损失函数,算出的是每个例子的平方,要求和(reduction_indices=[1],按行求和),再求均值loss=tf.reduce_mean(tf.reduce_sum(tf.square(ys-prediction),reduction_indices=[1]))"""训练"""#优化算法,minimize(loss)以0.1的学习率对loss进行减小train_step=tf.train.GradientDescentOptimizer(0.1).minimize(loss)init=tf.global_variables_initializer()with tf.Session() as sess:sess.run(init)for i in range(1000):sess.run(train_step,feed_dict={xs:x_data,ys:y_data})if i%50==0:print(sess.run(loss,feed_dict={xs:x_data,ys:y_data}))def test8():import matplotlib.pylab as plt"""定义数据形式"""# (-1,1)之间,有300个单位,后面的是维度,x_data是有300行(300个例子)x_data=np.linspace(-1,1,300)[:,np.newaxis]# 加噪声,均值为0,方差为0.05,大小和x_data一样noise=np.random.normal(0,0.05,x_data.shape)y_data=np.square(x_data)-0.5+noisexs=tf.placeholder(tf.float32,[None,1])ys=tf.placeholder(tf.float32,[None,1])"""建立网络"""#定义隐藏层,输入1个节点,输出10个节点l1=add_layer(xs,1,10,activation_function=tf.nn.relu)#定义输出层prediction=add_layer(l1,10,1,activation_function=None)"""预测"""#损失函数,算出的是每个例子的平方,要求和(reduction_indices=[1],按行求和),再求均值loss=tf.reduce_mean(tf.reduce_sum(tf.square(ys-prediction),reduction_indices=[1]))"""训练"""#优化算法,minimize(loss)以0.1的学习率对loss进行减小train_step=tf.train.GradientDescentOptimizer(0.1).minimize(loss)init=tf.global_variables_initializer()with tf.Session() as sess:sess.run(init)fig=plt.figure()#连续性的画图ax=fig.add_subplot(1,1,1)ax.scatter(x_data,y_data)# 不暂停plt.ion()# plt.show()绘制一次就会暂停# plt.show() #也可以用plt.show(block=False)来取消暂停,但是python3.5以后提供了ion的功能,更方便for i in range(1000):sess.run(train_step,feed_dict={xs:x_data,ys:y_data})if i%50==0:# print(sess.run(loss,feed_dict={xs:x_data,ys:y_data}))#尝试先抹除,后绘制第二条线#第一次没有线,会报错,try就会忽略错误,然后紧接着执行下面的步骤try:# 画出一条后抹除掉,去除第一个线段,但是只有一个,也就是抹除当前的线段ax.lines.remove(lines[0])except Exception:passprediction_value=sess.run(prediction,feed_dict={xs:x_data})lines=ax.plot(x_data,prediction_value,'r-',lw=5) #lw线宽# 暂停0.1splt.pause(0.1)#test1()
#test2()
#test3()
#test4()
#test5()
#test6()
#test7()
test8()
相关文章:
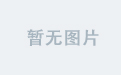
PCB多层线路板打样难点
PCB多层板无论从设计上还是制造上来说,都比单双层板要复杂,一不小心就会遇到一些问题,那在PCB多层线路板打样中我们要规避哪些难点呢? 1、层间对准的难点 由于多层电路板中层数众多,用户对PCB层的校准要求越来越…
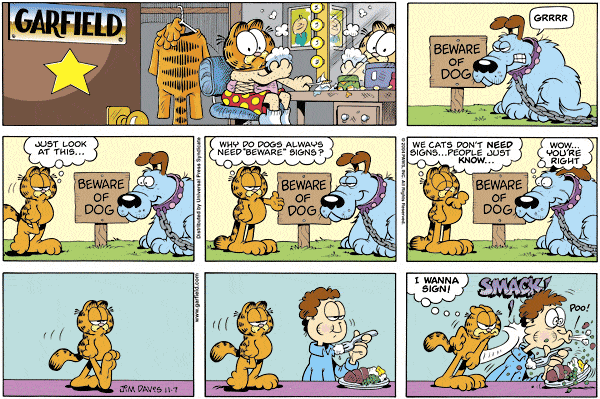
GARFIELD@11-07-2004
Vanity Fair转载于:https://www.cnblogs.com/rexhost/archive/2004/11/07/61286.html
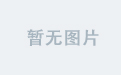
python文件读写1
# -*- coding: utf-8 -*-# read txt file def readTextFile(file):f open(file, r)# 尽可能多的读取文件的内容,一般会将整个文件内容都会读取context f.read() print(context)f.close()def readTextFileByLines(file):f open(file, "r")lines f.read…

jfinal框架下使用c3P0连接池连接sql server 2008
2019独角兽企业重金招聘Python工程师标准>>> 闲话少说 进入正题 首先是工程需要的jar包 然后是c3p0的配置文件。我是这样配置的 仅供参考 jdbcDriver com.microsoft.sqlserver.jdbc.SQLServerDriver jdbcUrl jdbc:sqlserver://localhost:7777;databaseNametest us…
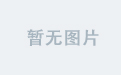
mongodb插入文档时不传ObjectId
type BookExt struct {ID bson.ObjectId bson:"_id"Title string bson:"title"SubTitle string bson:"subTitle"Author string bson:"author" } 以上结构体,在通过此结构体对象作为参数传入Insert插入…

[问题]DotNet 项目如何实现在构建时 Build 号自动增加?
[问题]DotNet 项目如何实现在构建时 Build 号自动增加? 继续昨天的问题,今天在Google上找了一下,没有找到很好的方案。目前找到的解决方案有以下几种:1.使用一个地三方的 VS.Net 插件,实现在编译时 Build 号自动增加&a…

编写程序记录文件位置
当我们编写程序是会注意到,首先是配置一些函数的结构体。 所以我们就要找到下面的界面,然后打开FWLB中.c文件下面所对应的.h文件,这样就能查找到相应的结构体。下图为我所找到的中断的结构体、 然后就是查找相对应的中断向量。具体就是打开 还…
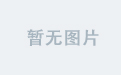
mnist数据集保存为图片
#coding: utf-8 from tensorflow.examples.tutorials.mnist import input_data import scipy.misc import os import numpy as np# 读取MNIST数据集。如果不存在会事先下载。 mnist input_data.read_data_sets("MNIST_data/", one_hotTrue)# 我们把原始图片保存在MN…
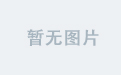
Python3数据分析与挖掘建模实战
<div>课程地址:http://icourse8.com/Python3_shujufenxi.html</div>复制代码第1章 课程介绍【赠送相关电子书随堂代码】 第2章 数据获取 第3章 单因子探索分析与数据可视化 第4章 多因子探索分析 第5章 预处理理论 第6章 挖掘建模 第7章 模型评估 第8章…
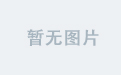
tensorflow生成对抗网络
import tensorflow as tf import numpy as np import os from tensorflow.examples.tutorials.mnist import input_data from matplotlib import pyplot as pltBATCH_SIZE 64 UNITS_SIZE 128 LEARNING_RATE 0.001 EPOCH 300 SMOOTH 0.1print("mnist手写体生成对抗网络…
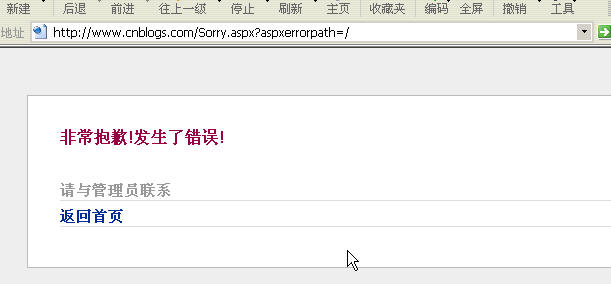
博客园今天早上是不是出现什么问题了?
下面是我进我的blog后台管理和浏览博客园给出的提示。大约几分钟后恢复正常。转载于:https://www.cnblogs.com/freeyzh/archive/2004/12/01/71269.html

模态框获取id一直不变,都是同一个id值
2019独角兽企业重金招聘Python工程师标准>>> $(.refund-btn).click(function(){//此处必须是$(this),否则$(.refund-btn)重新获取,导致值一直不变var id $(this).attr(data-id);//var id $(.refund-btn).attr(data-id);错误,这样会导致一直…

标准功能模块组件 -- 内部联络单组件,内部邮件组件,提高多人异地协同办公效率...
为什么80%的码农都做不了架构师?>>> 未必什么功能都需要自己开发,我们不会自己开发一个数据库系统,也不会自己开发一个操作系统,同样我们每个功能模块都未必需要自己开发,自己开发最核心的模块,…

Microsoft patterns practices Enterprise Library released
一直关注这个东西,本来订阅了RSS,没想到GotDotNet上面的发布信息给清空了。 上周末发布的,今天才看到,刚刚下载了一个,下载还要求注册,真麻烦,现把地址共享,方便大家。 http://down…
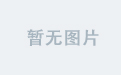
图论之拓扑排序 poj 2367 Genealogical tree
题目链接 http://poj.org/problem?id2367 题意就是给定一系列关系,按这些关系拓扑排序。 #include<cstdio> #include<cstring> #include<queue> #include<vector> #include<algorithm> using namespace std; const int maxn200; int…
算法基础知识科普:8大搜索算法之顺序搜索
基本概念和术语 搜索表(Search Table):是由同一类型的数据元素(或记录)构成的集合。 关键字(Key):是数据元素中某个数据项的值,用它可以标识一个数据元素。若此关键字…

foj2024
为什么80%的码农都做不了架构师?>>> http://acm.fzu.edu.cn/problem.php?pid2024 View Code #include < stdio.h > #include < string .h > #define M 1010 int c[M][M]; int f[M][M]; int min( int a, int b, int c){ int z …
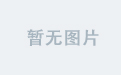
4701年新年快乐!
中华民族传统历法夏历(农历)采用的是干支纪年法,是世界上最古老的历法之一。干支即“六十甲子”,以60年为一循环。它的纪元开始相传可追溯到黄帝轩辕氏时代,按公元计算,第一个“甲子年”应是在公元前2697年…
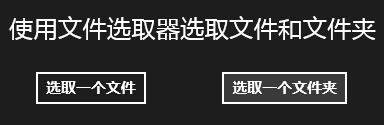
Win10系列:JavaScript访问文件和文件夹
在实际开发中经常会遇到访问文件的情况,因此学习与文件有关的操作对程序开发很有帮助,关于文件操作的一些基本技术,在前面章节中有专门基于C#语言的详细讲解,本节主要介绍如何使用HTML5和JavaScript开发具有文件操作功能的Windows…
算法基础知识科普:8大搜索算法之二分搜索
昨天介绍了对无序搜素表的顺序搜索方法,今天介绍对有序搜索表的二分搜索方法,“二分”在算法设计中是非常常用的一种思想,除了处理如下普通的搜索外,还用于搜索方程的解等工程领域。但二分法仍然有缺陷,待后面慢慢介绍…

linux之shell脚本学习篇一
为什么80%的码农都做不了架构师?>>> 此文包含脚本服务请求,字符串截取,文件读写内容,打印内容换行。 #!/bin/bash retMsg""; while read LINE do echo "this is text: $LINE"; retMsg/usr/bin/cu…

Win10系列:JavaScript动画2
"重新定位"动画也是Windows动画库中的动画效果。"重新定位"动画的动画效果是指一个或一组元素移动到新的位置时,这些元素不是突然出现在新的位置,而是从一个位置移动到另一个位置。 创建"重新定位"动画可以使用WinJS.UI.A…
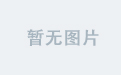
[转载]李开复先生给中国学生的第四封信:大学四年应是这样度过
今天,我回复了“开复学生网”开通以来的第1000个问题。关掉电脑后,始终有一封学生来信萦绕在我的脑海里,挥之不去: 开复老师: 就要毕业了。 回头看自己所谓的大学生活, 我想哭,不是因为离别&…
算法基础知识科普:8大搜索算法之插补搜索
二分法的不足在于,对于均匀分布的数据,缩小搜索范围的速度太慢,每次只能缩小原长度的1/2,我们希望缩小范围尽可能的快,即搜索的数据若离左端点近,搜索的区间尽量的靠近左端点,同理搜索的数据若离…

hdu(1596)
为什么80%的码农都做不了架构师?>>> dijkstra 1 #include " iostream " 2 using namespace std; 3 double map[ 1010 ][ 1010 ]; 4 int visit[ 1010 ]; 5 double used[ 1010 ]; 6 int k; 7 double _max 0 ; 8 int i…

使用ADO.NET 的最佳实践(zz)
数据访问:使用 ADO.NET 的最佳实践(ADO.NET 技术文档) 发布日期: 4/1/2004| 更新日期: 4/1/2004 摘要:编写 Microsoft ADO.NET 代码的最佳实践,以及对使用 ADO.NET 中可用对象的开发人员的建议。…
算法基础知识科普:8大搜索算法之二叉搜索树(上)
前几天,我们介绍了在顺序存储结构上构建的搜索算法,如二分搜素,插补搜索等,这种结构适合于静态搜索,但对于动态搜索会涉及到大量记录的移动导致效率的降低。这样我们自然会想是否能够利用链式的存储结构,这…
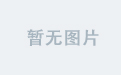
如何查看 oracle 官方文档
Concept 包含了 oracle 数据库里面的一些基本概念和原理, 比如 数据库逻辑结构, 物理结构, 实例结构, 优化器, 事务等. PDF 460页 Reference 包含了动态性能视图, 数据字典, 初始化参数等, 如果有参数不知道意思, 或者 v$视图字段信息模糊, 都可以从这里找到描述, 使用 html版的…
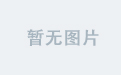
php开发面试题---攻击网站的常用手段有哪些,及如何预防(整理)
php开发面试题---攻击网站的常用手段有哪些,及如何预防(整理) 一、总结 一句话总结: 比较记忆:注意比较各种攻击的区别,比如csrf和xss,以及xss和sql,这样才能记住 1、Sql注入是什么&…

利用Event和MapFile进程共享信息
工作过程: 进程一, 建立映射文件,填写数据,并发出Event的信号; 进程二,打开映射文件,收到Event的信号时读取数据. #include <windows.h>#include <string.h>#include <iostream>usingnamespacestd; #defineFILE_SIZE 1024staticHANDLE hMapFile; staticLPVOI…