OpenCV下车牌定位算法实现代码
转自:http://blog.csdn.net/heihei723/archive/2006/05/14/728046.aspx#FeedBack
车牌定位算法在车牌识别技术中占有很重要地位,一个车牌识别系统的识别率往往取决于车牌定位的成功率及准确度。
车牌定位有很多种算法,从最简单的来,车牌在图像中一般被认为是长方形,由于图像摄取角度不同也可能是四边形。我们可以使用OpenCV中的实例: C:/Program Files/OpenCV/samples/c.squares.c 这是一个搜索图片中矩形的一个算法。我们只要稍微修改一下就可以实现定位车牌。
在这个实例中使用了canny算法进行边缘检测,然后二值化,接着用cvFindContours搜索轮廓,最后从找到的轮廓中根据角点的个数,角的度数和轮廓大小确定,矩形位置。以下是效果图:
这个算法可以找到一些车牌位置,但在复杂噪声背景下,或者车牌图像灰度与背景相差不大就很难定位车牌
所以我们需要寻找更好的定位算法。下面是squares的代码:
#ifdef _CH_
#pragma package <opencv>
#endif
#ifndef _EiC
#include "cv.h"
#include "highgui.h"
#include <stdio.h>
#include <math.h>
#include <string.h>
#endif
int thresh = 50;
IplImage* img = 0;
IplImage* img0 = 0;
CvMemStorage* storage = 0;
CvPoint pt[4];
const char* wndname = "Square Detection Demo";
// helper function:
// finds a cosine of angle between vectors
// from pt0->pt1 and from pt0->pt2
double angle( CvPoint* pt1, CvPoint* pt2, CvPoint* pt0 )
{
double dx1 = pt1->x - pt0->x;
double dy1 = pt1->y - pt0->y;
double dx2 = pt2->x - pt0->x;
double dy2 = pt2->y - pt0->y;
return (dx1*dx2 + dy1*dy2)/sqrt((dx1*dx1 + dy1*dy1)*(dx2*dx2 + dy2*dy2) + 1e-10);
}
// returns sequence of squares detected on the image.
// the sequence is stored in the specified memory storage
CvSeq* findSquares4( IplImage* img, CvMemStorage* storage )
{
CvSeq* contours;
int i, c, l, N = 11;
CvSize sz = cvSize( img->width & -2, img->height & -2 );
IplImage* timg = cvCloneImage( img ); // make a copy of input image
IplImage* gray = cvCreateImage( sz, 8, 1 );
IplImage* pyr = cvCreateImage( cvSize(sz.width/2, sz.height/2), 8, 3 );
IplImage* tgray;
CvSeq* result;
double s, t;
// create empty sequence that will contain points -
// 4 points per square (the square's vertices)
CvSeq* squares = cvCreateSeq( 0, sizeof(CvSeq), sizeof(CvPoint), storage );
// select the maximum ROI in the image
// with the width and height divisible by 2
cvSetImageROI( timg, cvRect( 0, 0, sz.width, sz.height ));
// down-scale and upscale the image to filter out the noise
cvPyrDown( timg, pyr, 7 );
cvPyrUp( pyr, timg, 7 );
tgray = cvCreateImage( sz, 8, 1 );
// find squares in every color plane of the image
for( c = 0; c < 3; c++ )
{
// extract the c-th color plane
cvSetImageCOI( timg, c+1 );
cvCopy( timg, tgray, 0 );
// try several threshold levels
for( l = 0; l < N; l++ )
{
// hack: use Canny instead of zero threshold level.
// Canny helps to catch squares with gradient shading
if( l == 0 )
{
// apply Canny. Take the upper threshold from slider
// and set the lower to 0 (which forces edges merging)
cvCanny( tgray, gray,60, 180, 3 );
// dilate canny output to remove potential
// holes between edge segments
cvDilate( gray, gray, 0, 1 );
}
else
{
// apply threshold if l!=0:
// tgray(x,y) = gray(x,y) < (l+1)*255/N ? 255 : 0
//cvThreshold( tgray, gray, (l+1)*255/N, 255, CV_THRESH_BINARY );
cvThreshold( tgray, gray, 50, 255, CV_THRESH_BINARY );
}
// find contours and store them all as a list
cvFindContours( gray, storage, &contours, sizeof(CvContour),
CV_RETR_LIST, CV_CHAIN_APPROX_SIMPLE, cvPoint(0,0) );
// test each contour
while( contours )
{
// approximate contour with accuracy proportional
// to the contour perimeter
result = cvApproxPoly( contours, sizeof(CvContour), storage,
CV_POLY_APPROX_DP, cvContourPerimeter(contours)*0.02, 0 );
// square contours should have 4 vertices after approximation
// relatively large area (to filter out noisy contours)
// and be convex.
// Note: absolute value of an area is used because
// area may be positive or negative - in accordance with the
// contour orientation
if( result->total == 4 &&
fabs(cvContourArea(result,CV_WHOLE_SEQ)) > 1000 &&
cvCheckContourConvexity(result) )
{
s = 0;
for( i = 0; i < 5; i++ )
{
// find minimum angle between joint
// edges (maximum of cosine)
if( i >= 2 )
{
t = fabs(angle(
(CvPoint*)cvGetSeqElem( result, i ),
(CvPoint*)cvGetSeqElem( result, i-2 ),
(CvPoint*)cvGetSeqElem( result, i-1 )));
s = s > t ? s : t;
}
}
// if cosines of all angles are small
// (all angles are ~90 degree) then write quandrange
// vertices to resultant sequence
if( s < 0.3 )
for( i = 0; i < 4; i++ )
cvSeqPush( squares,
(CvPoint*)cvGetSeqElem( result, i ));
}
// take the next contour
contours = contours->h_next;
}
}
}
// release all the temporary images
cvReleaseImage( &gray );
cvReleaseImage( &pyr );
cvReleaseImage( &tgray );
cvReleaseImage( &timg );
return squares;
}
// the function draws all the squares in the image
void drawSquares( IplImage* img, CvSeq* squares )
{
CvSeqReader reader;
IplImage* cpy = cvCloneImage( img );
int i;
// initialize reader of the sequence
cvStartReadSeq( squares, &reader, 0 );
// read 4 sequence elements at a time (all vertices of a square)
for( i = 0; i < squares->total; i += 4 )
{
CvPoint* rect = pt;
int count = 4;
// read 4 vertices
memcpy( pt, reader.ptr, squares->elem_size );
CV_NEXT_SEQ_ELEM( squares->elem_size, reader );
memcpy( pt + 1, reader.ptr, squares->elem_size );
CV_NEXT_SEQ_ELEM( squares->elem_size, reader );
memcpy( pt + 2, reader.ptr, squares->elem_size );
CV_NEXT_SEQ_ELEM( squares->elem_size, reader );
memcpy( pt + 3, reader.ptr, squares->elem_size );
CV_NEXT_SEQ_ELEM( squares->elem_size, reader );
// draw the square as a closed polyline
cvPolyLine( cpy, &rect, &count, 1, 1, CV_RGB(0,255,0), 3, CV_AA, 0 );
}
// show the resultant image
cvShowImage( wndname, cpy );
cvReleaseImage( &cpy );
}
void on_trackbar( int a )
{
if( img )
drawSquares( img, findSquares4( img, storage ) );
}
char* names[] = { "pic1.png", "pic2.png", "pic3.png",
"pic4.png", "pic5.png", "pic6.png", 0 };
int main(int argc, char** argv)
{
int i, c;
// create memory storage that will contain all the dynamic data
storage = cvCreateMemStorage(0);
for( i = 0; names[i] != 0; i++ )
{
// load i-th image
img0 = cvLoadImage( names[i], 1 );
if( !img0 )
{
printf("Couldn't load %s/n", names[i] );
continue;
}
img = cvCloneImage( img0 );
// create window and a trackbar (slider) with parent "image" and set callback
// (the slider regulates upper threshold, passed to Canny edge detector)
cvNamedWindow( wndname,0 );
cvCreateTrackbar( "canny thresh", wndname, &thresh, 1000, on_trackbar );
// force the image processing
on_trackbar(0);
// wait for key.
// Also the function cvWaitKey takes care of event processing
c = cvWaitKey(0);
// release both images
cvReleaseImage( &img );
cvReleaseImage( &img0 );
// clear memory storage - reset free space position
cvClearMemStorage( storage );
if( c == 27 )
break;
}
cvDestroyWindow( wndname );
return 0;
}
#ifdef _EiC
main(1,"squares.c");
#endif
前面介绍了用OpenCV的squares实例定位车牌的算法,效果不是很理想。车牌定位的方法有很多种,这里我们从汽车图像的纹理特征入手,找出车牌不同于背景的特征是车牌定位的关键。观察多幅汽车图片我们会发现车身和背景的纹理多为横向纹理,而车牌字符则为竖向纹理,基于这个纹理特征我们可以区别处车牌位置。车牌的定位我们可以分为如下几个步骤:
1预处理
图像的预处理主要是为了后续处理的需要进行一些滤波和梯度增强的处理,以滤除噪声和垂直方向上的增强。
2 Sobel垂直方向边缘检测并2值化
一般的边缘检测的方法检测到的边缘信息含有大量的无用信息,这里我们使用Sobel边缘检测算子对图像进行垂直边缘检测,由于车牌字符的竖向纹理特征我们可以检测到车牌字符,对得到的边缘图像进行2值化,排除一些噪声并增强字符边缘。
3形态学变换
汽车图像还存在一些竖向的纹理(如车身,车灯等)我们需要排除这些干扰信息,并把临近的字符边缘连通起来。这一阶段主要利用对图像进行形态学操作,即作闭合(先膨胀在腐蚀)开启(先腐蚀再膨胀)运算,膨胀可以把临近边缘连接成一个整体,腐蚀可以滤除一些细碎的边缘(建议使用3*1的结构进行操作)经过形态学变换可以滤除噪声边缘,得到车牌区域。
4筛选
大多数图像经过形态学变化后都可以得到满意的效果,但由于图像背景的一些特殊纹理,可能会留下一些没有滤除的边缘,也有可能字符间空隙较大,车牌区域出现分为几部分的状况,还有可能车身的一些标语等被误认为是车牌图像等,我们还需要进行聚类和筛选。
选取一个标准来判断连通域(如竖直方向两区域重合大于10像素,水平方向两区域距离小于10像素)在根据车牌的形状大小(宽,高)判断是否为车牌区。
程序运行效果图如下:
相关文章:
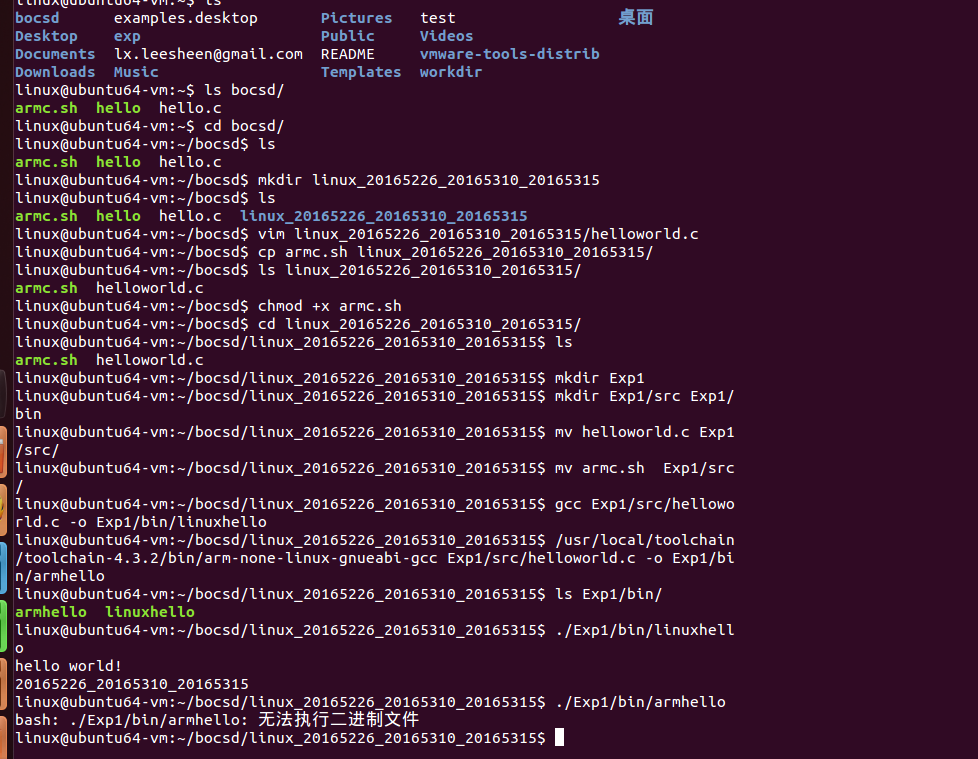
2018-2019-1 20165310 20165315 20165226 实验一 开发环境的熟悉
2018-2019-1 20165226 20165310 20165315 实验一 开发环境的熟悉 目录 一、实验目的 二、实验步骤 三、实验过程中遇到的问题及解决 四、实验感想 一、实验目的 熟悉Linux开发环境并学会Linux开发环境的配置和使用,熟悉arm箱的使用,熟悉以下知识点&#…
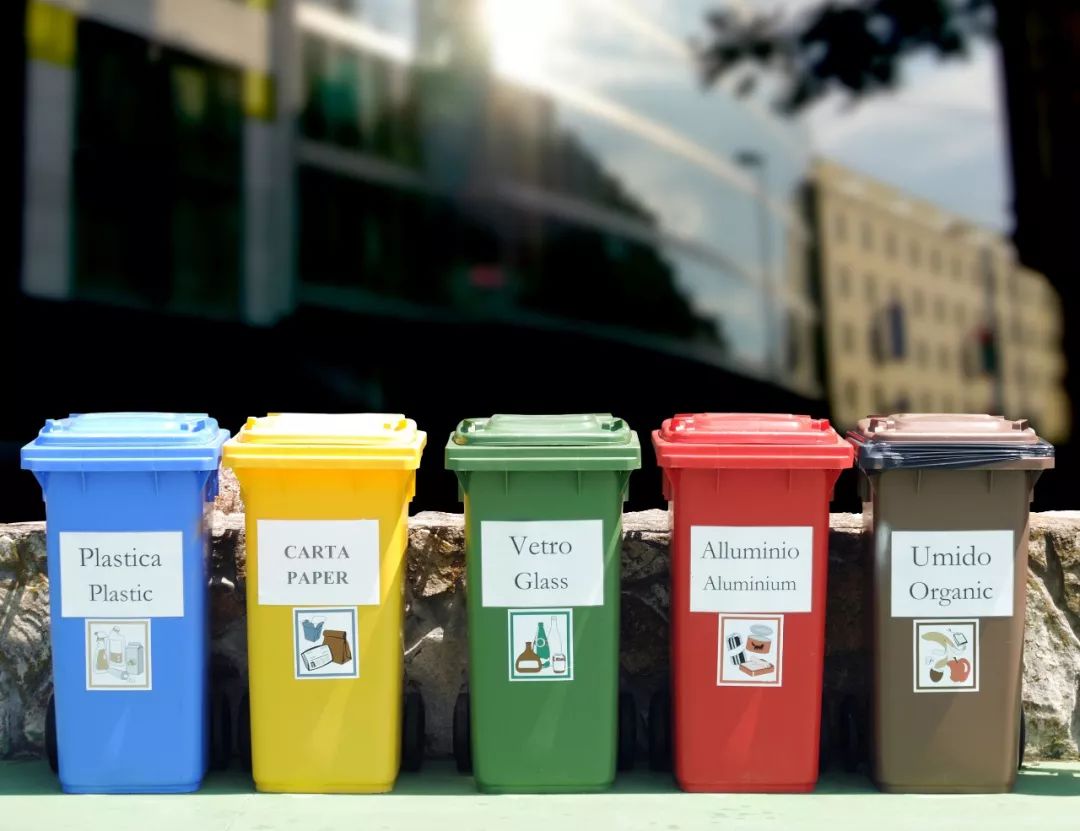
上海居民被垃圾分类逼疯!这款垃圾自动分类器也许能帮上忙
作者 | 视说君来源 | 授权转载自视说AI(ID:techtalkai)让垃圾自动分类近期垃圾分类成为了一个热门话题,原来直接一次性扔掉的垃圾,现在都需要分门别类进行投放。从今年7月1日起,新的《上海市生活垃圾管理条例》正式开始…
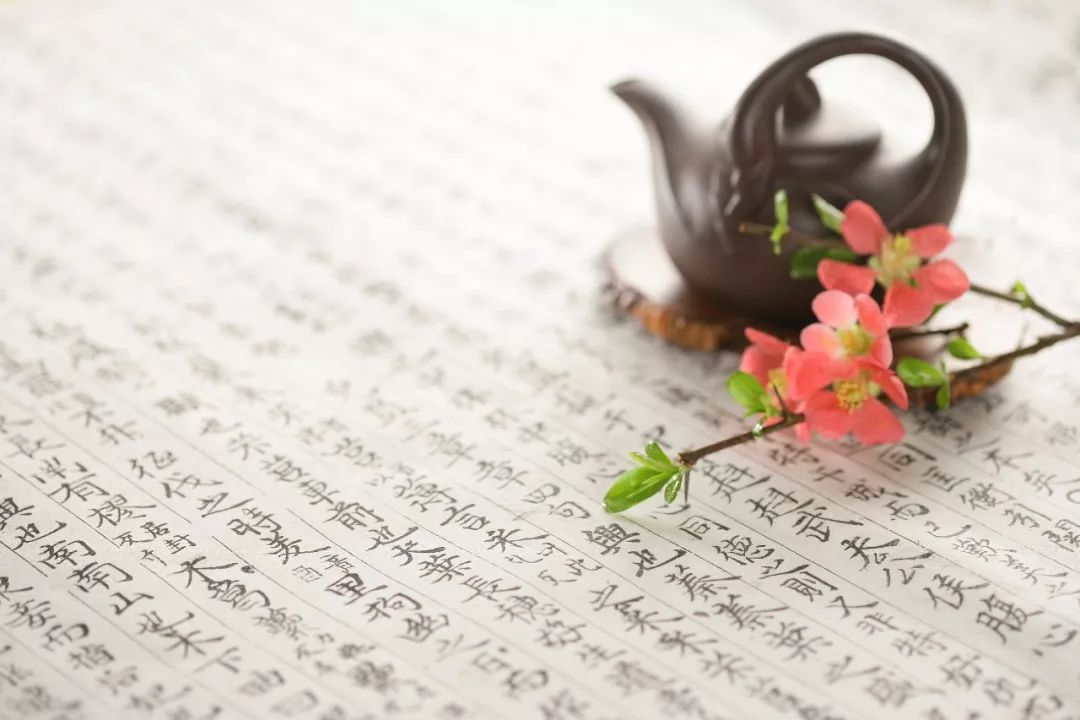
虽被BERT碾压,但还是有必要谈谈BERT时代与后时代的NLP
作者 | 吴金龙,爱因互动技术合伙人,算法负责人来源 | 授权转载自AINLP(ID:nlpjob)2018年是NLP的收获大年,模型预训练技术终于被批量成功应用于多项NLP任务。之前搞NLP的人一直羡慕搞CV的人,在ImageNet上训练…
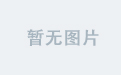
C++ 简单读写文本文件、统计文件的行数、读取文件数据到数组
转自:http://hi.baidu.com/ctralt/blog/item/cde79fec87f841302697911c.html fstream提供了三个类,用来实现c对文件的操作。(文件的创建、读、写)。ifstream -- 从已有的文件读 ofstream -- 向文件写内容 fstream - 打开文件供读写…

Exchange 2007迁移2010时的公用文件夹多个公用树错误
近期在项目中,客户Exchange 从2007迁移到2010,Microsoft Exchange从2007开始就逐渐弱化了公用文件夹,outlook 2007和2010都不需要使用到公用文件夹了。但客户现状存在90%的outlook 2003客户端,因此需要使用到公用文件夹。在Exchan…
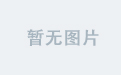
约瑟夫环问题的两种解法(详解)
约瑟夫环问题的两种解法(详解) 题目: Josephus有过的故事:39 个犹太人与Josephus及他的朋友躲到一个洞中,39个犹太人决定宁愿死也不要被敌人抓。于是决定了自杀方式,41个人排成一个圆圈,由第1个…
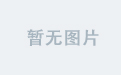
Linux下多播的配置【十全十美】
单播地址标识单个IP接口,广播地址标识某个子网的所有IP接口。多播地址表示某一组IP接口,单播和广播是寻址方案中的两个极端(要么单个要么全部),多播则意在两者之间提供一种折中方案。多播是用于建立分布式系统的重要工具,例如&…
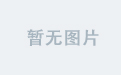
CvSeq相关函数
转自:http://hi.baidu.com/pengjun/blog/item/a72fc8ea030e79d4d439c906.html 函数原型说明CvSeq* cvCreateSeq(int seq_flags,int header_size,int elem_size,CvMemStorage* storage)功能:创建一序列 参数:seq_flags为序列的符号标志。如果序…

10月份机房技术指标
下载syslinux,dhcp,http,tftp-serveryum -y install syslinux dhcp httpd tftp-serveryum -y install system-config-kickstart挂载sr0是镜像用system-config-kickstart工具来生成一个自动的安装的配置文件ip填自己的ip地址。目录填挂载光盘的…
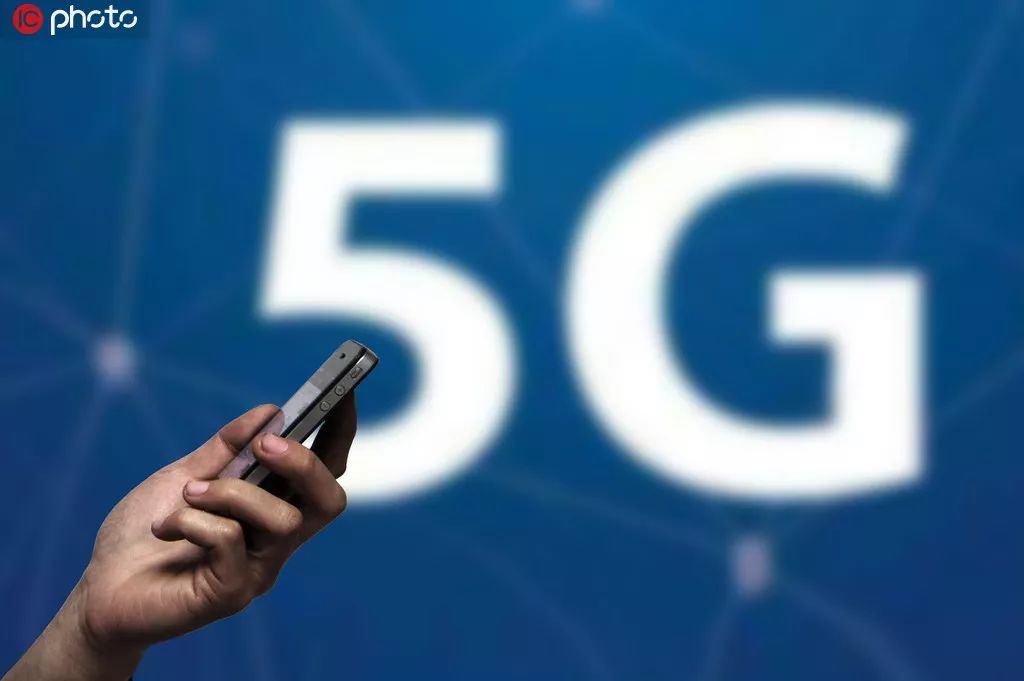
5G时代,微软又走对了一步棋!
2019年4月,CSDN采访微软(中国)首席技术官韦青,期间谈到5G。他认为,5G绝对是一个划时代的革命性突破,但是这个突破不止于现在所说的“5G”通讯技术,它为未来以“万物互联”为基础的智能社会开创了…

6426C Lab3 部署证书和管理注册
共有4个练习:练习1:配置证书模板练习2:配置自动注册练习3:管理证书 Revocation练习4:配置Key Recovery练习1:任务1:复制、安装和手动注册一个证书1. 转到HQDC1.contoso.com服务器,添…
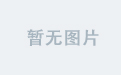
CreateStructuringElementEx
转自:http://baike.baidu.com/view/4819443.htm CreateStructuringElementEx 创建结构元素 IplConvKernel* cvCreateStructuringElementEx( int cols, int rows, int anchor_x, int anchor_y, int shape, int* valuesNULL ); cols 结构元素的列数目 rows 结构…
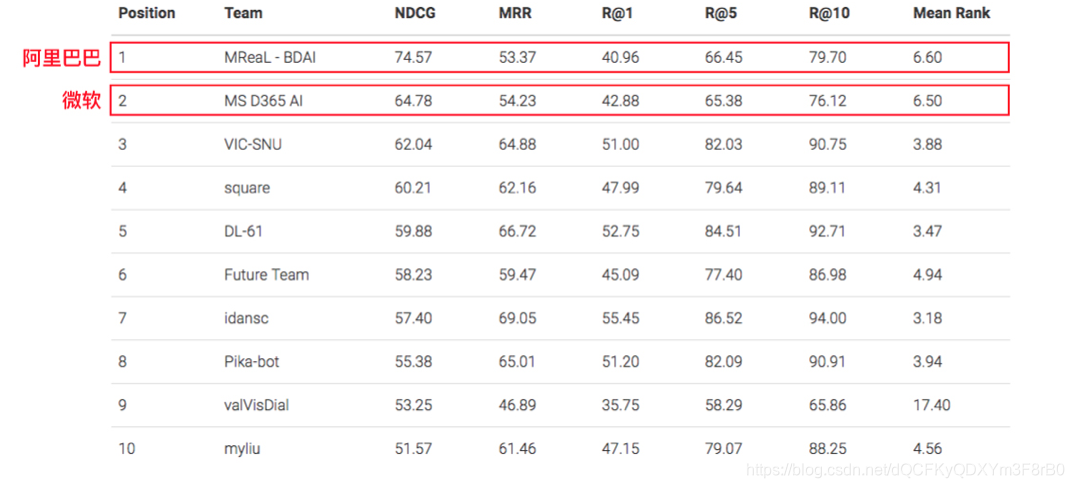
阿里AI再摘一冠,大幅提高视觉对话世界纪录
近日, 在第二届视觉对话挑战赛Visual Dialogue Challenge中,阿里AI击败了微软、首尔大学等十支参赛队伍,获得冠军。 (阿里AI在视觉对话竞赛中得冠)视觉对话竞赛由美国佐治亚理工大学、Facebook人工智能实验室ÿ…

OSChina 周一乱弹 —— 嫂子我帮你们照顾放心吧
2019独角兽企业重金招聘Python工程师标准>>> Osc乱弹歌单(2018)请戳(这里) 【今日歌曲】 clouddyy :#每日一歌# 《绿光 - 孙燕姿》 《绿光》 - 孙燕姿 手机党少年们想听歌,请使劲儿戳࿰…
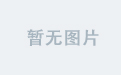
十一月工作小记--上线前的冲刺
加班不是目的,重要的是找到加班的意义。尽管程序猿们有很多个不愿意,他们却依然要面对加班的现实。加班就是程序猿们生活中的一张牌,既然不能决定这张牌是什么,那就想想如何去打好这张牌吧。本月,我们的生活依然是那么…
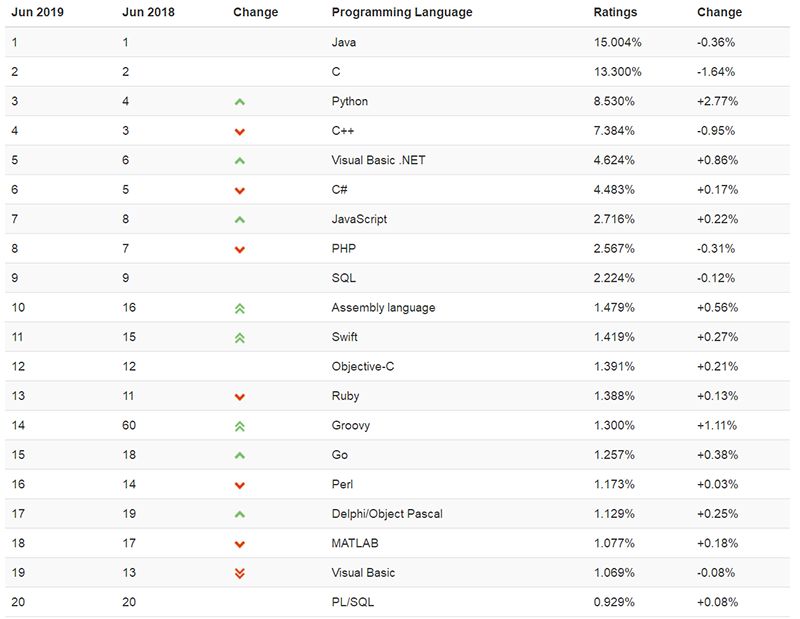
Java跌落神坛,Python继续夺冠....凭啥?
编程语言流行指数(PYPL)排行榜近日公布了2019年6月份榜单。相比 5 月编程语言榜单,Python 不仅超过了 C,成功占据第三名位置,还以 2.77% 的涨幅成为增速最快的编程语言,与此同时,拥有 8.53% 份额的 Python 达到了 TIOB…
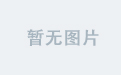
opencv实现二值图像细化的算法
转自:http://blog.csdn.net/byxdaz/archive/2010/06/02/5642669.aspx 细化算法通常和骨骼化、骨架化算法是相同的意思,也就是thin算法或者skeleton算法。虽然很多图像处理的教材上不是这么写的,具体原因可以看这篇论文,Louisa Lam…
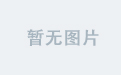
@芥末的糖----------《管理系统后台架构逻辑》
mongo逻辑 //1.创建mongoose对象链接数据库,并暴露 var mongoose require(mongoose) mongoose.connect(mongodb://localhost:27017/lagou, {useNewUrlParser: true })var db mongoose.connection db.on(error, console.error.bind(console, connection error:)) d…
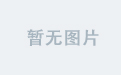
PHP函数之无极分类
无极分类属于现在比较难攻克的一关,现在就把代码贴出来,有需要的朋友可以根据实际需要扩展一下。 //假设分类关系为“ 地球”(id为1,父id为0),“国家”(id为2,父id为1)&a…
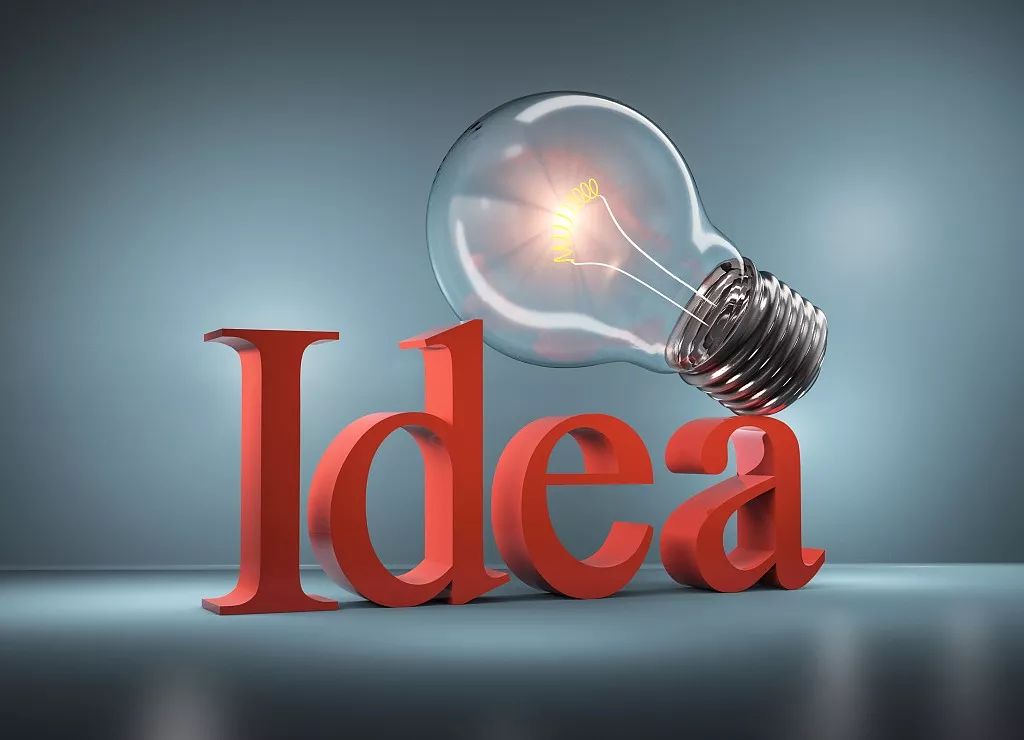
我发现了一个非常酷的软件,用自然语言编程!
作者 | 刘欣,前IBM架构师,用15年的技术工作经验去总结提炼,以故事讲解技术本质,让大家看过以后有一种“原来如此”的感觉。来源 | 码农翻身(公众号id:coderising)周六晚上10点半, 张…
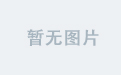
Matlab中去除exe执行时文件的DOS窗口的方法
转自:http://www.matlabsky.com/thread-547-1-1.html 方法1在command window中输入如下命令: cd(prefdir) edit compopts.bat 此时compopts.bat打开,在文件最后添加 A.VC环境下: set LINKFLAGS%LINKFLAGS%/SUBSYSTEM:WINDOWS /ENT…
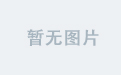
ubuntu14.04 升级gcc的方法
Ubuntu12..4版本也可正常安装。 1、添加软件源 sudo add-apt-repository ppa:ubuntu-toolchain-r/test sudo apt-get update2、安装gcc高版本,gcc4.8,gcc4.9,gcc5 gcc4.8 sudo apt-get upgrade sudo apt-get install gcc-4.8 g-4.8gcc4.9 sud…
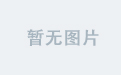
Java 基础【04】Swing 组件事件注册
聪明出于勤奋,天才在于积累。——华罗庚 对上次的三个问题的个人理解: 1) 程序首先是从main函数开始执行的,假设main 函数不是 static ,就要先实例化这个类,然后调用 main 方法,这似乎是不现实…
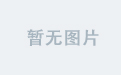
VC++ 隐藏控制台程序窗口
转自:http://hi.baidu.com/sicceer/blog/item/d9c35a810d15c4c8bc3e1ec8.html 设置 #pragma comment( linker, "/subsystem:/ "windows/ " /entry:/ "mainCRTStartup/ " " ) // 设置入口地址 这样就ok了 在控制台程序中隐藏控制台窗口…
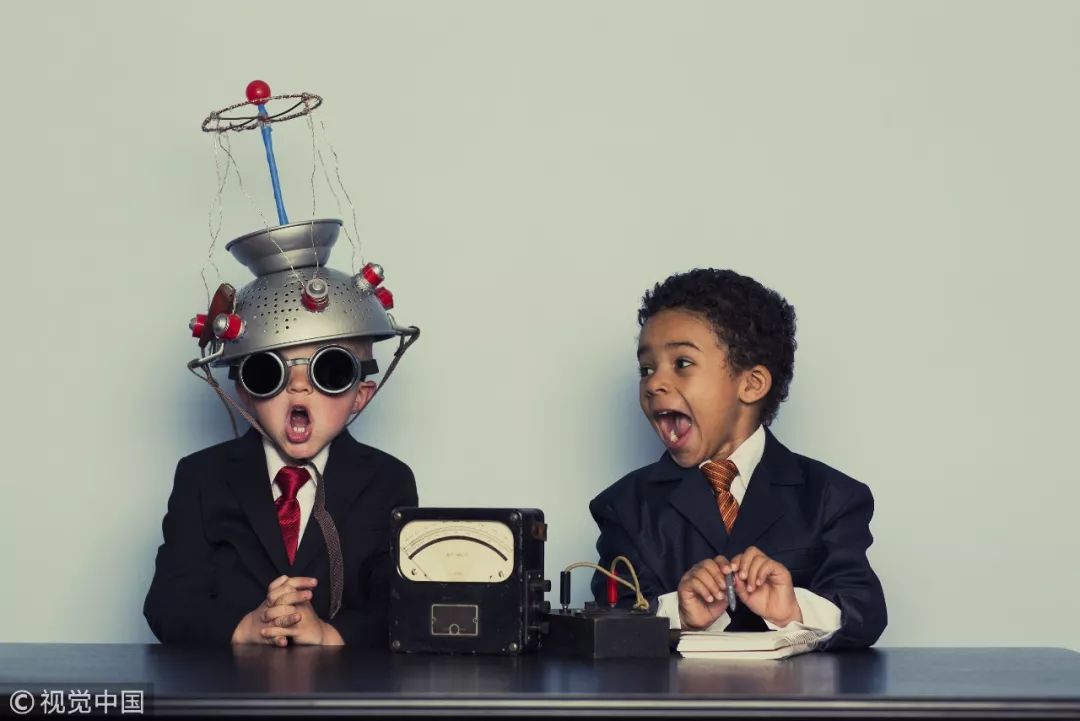
深度学习原来还可以这么学!
最近身边很多朋友在讨论人工智能,讨论人工智能在我们生活中的应用,随之而来就开始讨论深度学习技术,但是由于深度学习的涉及面比较广,对数学的要求比较高,所以想学也不太敢学,生怕认真学了却没学会。其实可…
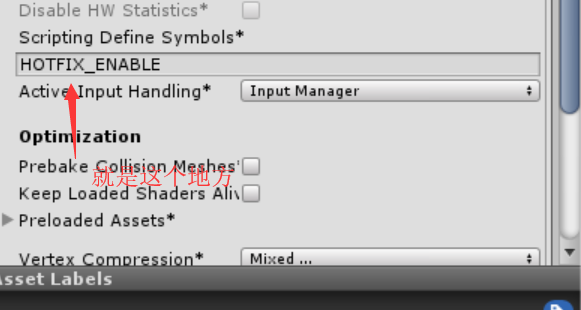
016-热更新之FishingJoy一
我们在完成对xlua的学习后,现在我们在接下来的几天中,将会用一个案例来学习一下xlua的使用。请大家不用担心,这个课件的使用是基于xlua而开发的。因为我们在这个部分是为了使用xlua,所以我们只在已经做到的案例上进行xlua的学习。…
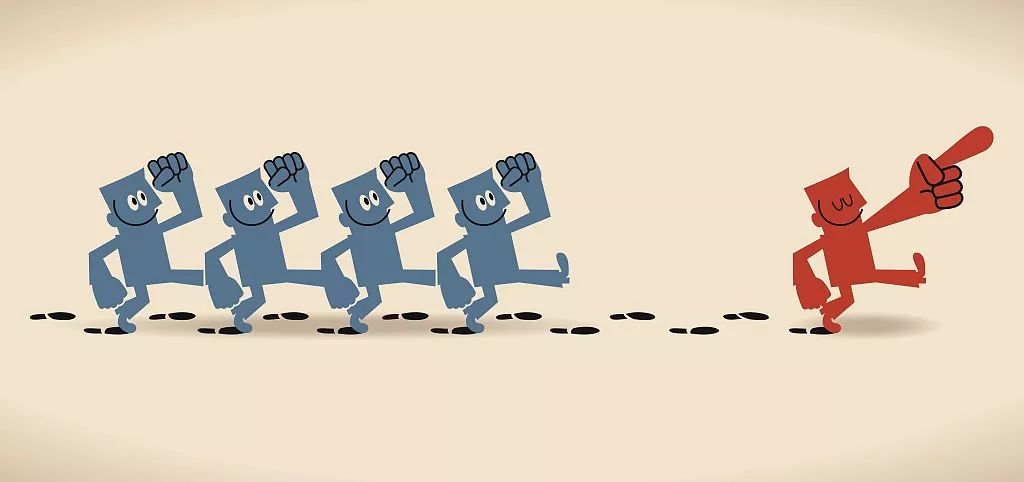
从0到1 | 手把手教你如何使用哈工大NLP工具——PyLTP!
作者 | 杨秀璋来源 | CSDN 博客(CSDN id:Eastmount)(本文经作者授权,此系列文章整理后微信平台首发于AI科技大本营)【导语】此文是作者基于 Python 构建知识图谱的系列实践教程,具有一定创新性和…
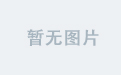
PL/SQL Developer远程访问Oracle数据库
安装oracle对应的版本 ,在oracle的安装目录找到oracle\product\11.2.0\dbhome_1\NETWORK\ADMIN\tnsnames.ora这个文件添加上数据库访问的串 LWZC (DESCRIPTION (ADDRESS (PROTOCOL TCP)(HOST [服务器地址])(PORT 1521))(CONNECT_DATA (SERVER DEDICATED)(SE…
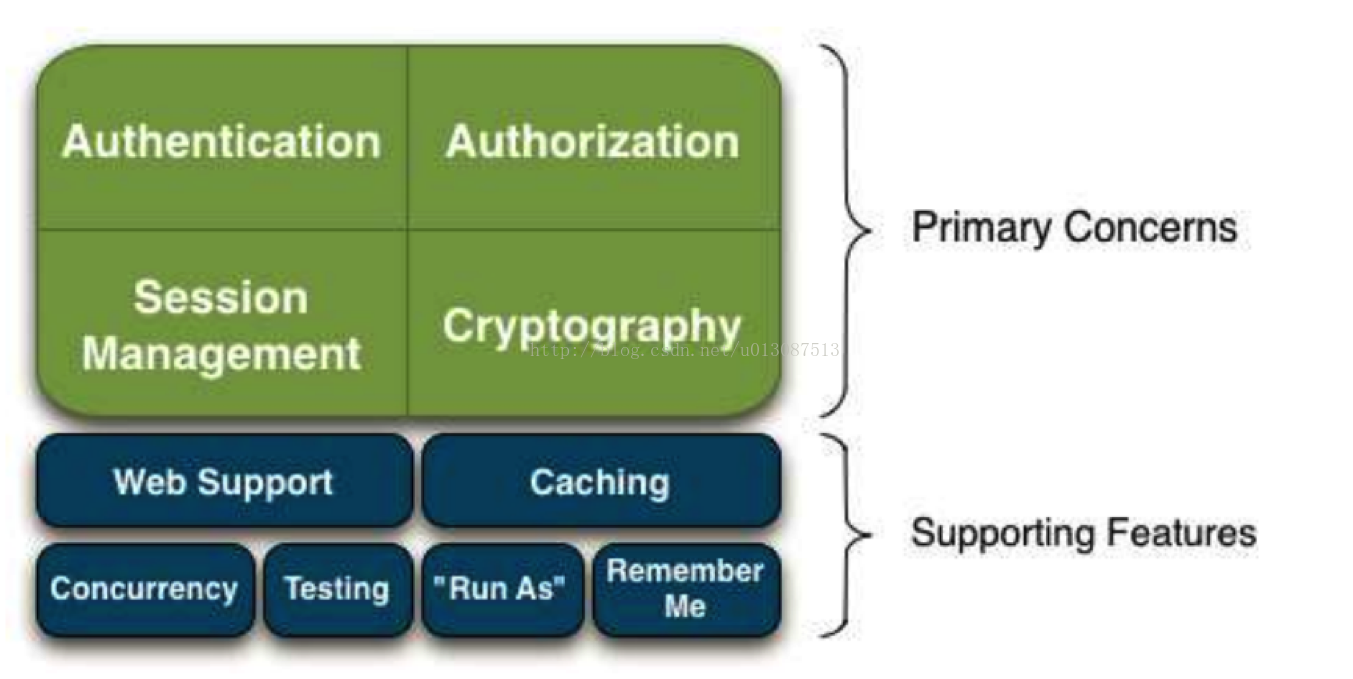
基于shiro的权限设计
shiro介绍 Apache shiro是一个权限控制框架,它将安全认证抽取出来,实现用户身份认证,权限授权,加密,会话管理等功能,是一个通用的安全认证框架,而且还可以用于分布式集群。功能如下 1.验证用户 …
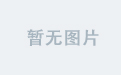
C++ 中隐藏DOS调用的命令行窗口
转自:http://hi.baidu.com/jackyho2000/blog/item/b5c5fabdd3b4db0019d81fbb.html 我演示了一下在MFC程序中怎么应用DOS的dir的命令,可是我们遇到了需要解决的问题,首先就是文件dir.txt的残留问题,其实这个问题很简单,…