决策树的C++实现(CART)
关于决策树的介绍可以参考: https://blog.csdn.net/fengbingchun/article/details/78880934
CART算法的决策树的Python实现可以参考: https://blog.csdn.net/fengbingchun/article/details/78881143
这里参考 https://machinelearningmastery.com/implement-decision-tree-algorithm-scratch-python/ 这篇文章的原有Python实现,使用C++实现了决策树的CART算法,测试数据集是Banknote Dataset,关于Banknote Dataset的介绍可以参考: https://blog.csdn.net/fengbingchun/article/details/78624358 。
decision_tree.hpp文件内容如下:
#ifndef FBC_NN_DECISION_TREE_HPP_
#define FBC_NN_DECISION_TREE_HPP_#include <vector>
#include <tuple>
#include <fstream>namespace ANN {
// referecne: https://machinelearningmastery.com/implement-decision-tree-algorithm-scratch-python/template<typename T>
class DecisionTree { // CART(Classification and Regression Trees)
public:DecisionTree() = default;~DecisionTree() { delete_tree(); }int init(const std::vector<std::vector<T>>& data, const std::vector<T>& classes);void set_max_depth(int max_depth) { this->max_depth = max_depth; }int get_max_depth() const { return max_depth; }void set_min_size(int min_size) { this->min_size = min_size; }int get_min_size() const { return min_size; }void train();int save_model(const char* name) const;int load_model(const char* name);T predict(const std::vector<T>& data) const;protected:typedef std::tuple<int, T, std::vector<std::vector<std::vector<T>>>> dictionary; // index of attribute, value of attribute, groups of datatypedef std::tuple<int, int, T, T, T> row_element; // flag, index, value, class_value_left, class_value_righttypedef struct binary_tree {dictionary dict;T class_value_left = (T)-1.f;T class_value_right = (T)-1.f;binary_tree* left = nullptr;binary_tree* right = nullptr;} binary_tree;// Calculate the Gini index for a split datasetT gini_index(const std::vector<std::vector<std::vector<T>>>& groups, const std::vector<T>& classes) const;// Select the best split point for a datasetdictionary get_split(const std::vector<std::vector<T>>& dataset) const;// Split a dataset based on an attribute and an attribute valuestd::vector<std::vector<std::vector<T>>> test_split(int index, T value, const std::vector<std::vector<T>>& dataset) const;// Create a terminal node valueT to_terminal(const std::vector<std::vector<T>>& group) const;// Create child splits for a node or make terminalvoid split(binary_tree* node, int depth);// Build a decision treevoid build_tree(const std::vector<std::vector<T>>& train);// Print a decision treevoid print_tree(const binary_tree* node, int depth = 0) const;// Make a prediction with a decision treeT predict(binary_tree* node, const std::vector<T>& data) const;// calculate accuracy percentagedouble accuracy_metric() const;void delete_tree();void delete_node(binary_tree* node);void write_node(const binary_tree* node, std::ofstream& file) const;void node_to_row_element(binary_tree* node, std::vector<row_element>& rows, int pos) const;int height_of_tree(const binary_tree* node) const;void row_element_to_node(binary_tree* node, const std::vector<row_element>& rows, int n, int pos);private:std::vector<std::vector<T>> src_data;binary_tree* tree = nullptr;int samples_num = 0;int feature_length = 0;int classes_num = 0;int max_depth = 10; // maximum tree depthint min_size = 10; // minimum node recordsint max_nodes = -1;
};} // namespace ANN#endif // FBC_NN_DECISION_TREE_HPP_
decision_tree.cpp文件内容如下:
#include "decision_tree.hpp"
#include <set>
#include <algorithm>
#include <typeinfo>
#include <iterator>
#include "common.hpp"namespace ANN {template<typename T>
int DecisionTree<T>::init(const std::vector<std::vector<T>>& data, const std::vector<T>& classes)
{CHECK(data.size() != 0 && classes.size() != 0 && data[0].size() != 0);this->samples_num = data.size();this->classes_num = classes.size();this->feature_length = data[0].size() -1;for (int i = 0; i < this->samples_num; ++i) {this->src_data.emplace_back(data[i]);}return 0;
}template<typename T>
T DecisionTree<T>::gini_index(const std::vector<std::vector<std::vector<T>>>& groups, const std::vector<T>& classes) const
{// Gini calculation for a group// proportion = count(class_value) / count(rows)// gini_index = (1.0 - sum(proportion * proportion)) * (group_size/total_samples)// count all samples at split pointint instances = 0;int group_num = groups.size();for (int i = 0; i < group_num; ++i) {instances += groups[i].size();}// sum weighted Gini index for each groupT gini = (T)0.;for (int i = 0; i < group_num; ++i) {int size = groups[i].size();// avoid divide by zeroif (size == 0) continue;T score = (T)0.;// score the group based on the score for each classT p = (T)0.;for (int c = 0; c < classes.size(); ++c) {int count = 0;for (int t = 0; t < size; ++t) {if (groups[i][t][this->feature_length] == classes[c]) ++count;}T p = (float)count / size;score += p * p;}// weight the group score by its relative sizegini += (1. - score) * (float)size / instances;}return gini;
}template<typename T>
std::vector<std::vector<std::vector<T>>> DecisionTree<T>::test_split(int index, T value, const std::vector<std::vector<T>>& dataset) const
{std::vector<std::vector<std::vector<T>>> groups(2); // 0: left, 1: reightfor (int row = 0; row < dataset.size(); ++row) {if (dataset[row][index] < value) {groups[0].emplace_back(dataset[row]);} else {groups[1].emplace_back(dataset[row]);}}return groups;
}template<typename T>
std::tuple<int, T, std::vector<std::vector<std::vector<T>>>> DecisionTree<T>::get_split(const std::vector<std::vector<T>>& dataset) const
{std::vector<T> values;for (int i = 0; i < dataset.size(); ++i) {values.emplace_back(dataset[i][this->feature_length]);}std::set<T> vals(values.cbegin(), values.cend());std::vector<T> class_values(vals.cbegin(), vals.cend());int b_index = 999;T b_value = (T)999.;T b_score = (T)999.;std::vector<std::vector<std::vector<T>>> b_groups(2);for (int index = 0; index < this->feature_length; ++index) {for (int row = 0; row < dataset.size(); ++row) {std::vector<std::vector<std::vector<T>>> groups = test_split(index, dataset[row][index], dataset);T gini = gini_index(groups, class_values);if (gini < b_score) {b_index = index;b_value = dataset[row][index];b_score = gini;b_groups = groups;}}}// a new node: the index of the chosen attribute, the value of that attribute by which to split and the two groups of data split by the chosen split pointreturn std::make_tuple(b_index, b_value, b_groups);
}template<typename T>
T DecisionTree<T>::to_terminal(const std::vector<std::vector<T>>& group) const
{std::vector<T> values;for (int i = 0; i < group.size(); ++i) {values.emplace_back(group[i][this->feature_length]);}std::set<T> vals(values.cbegin(), values.cend());int max_count = -1, index = -1;for (int i = 0; i < vals.size(); ++i) {int count = std::count(values.cbegin(), values.cend(), *std::next(vals.cbegin(), i));if (max_count < count) {max_count = count;index = i;}}return *std::next(vals.cbegin(), index);
}template<typename T>
void DecisionTree<T>::split(binary_tree* node, int depth)
{std::vector<std::vector<T>> left = std::get<2>(node->dict)[0];std::vector<std::vector<T>> right = std::get<2>(node->dict)[1];std::get<2>(node->dict).clear();// check for a no splitif (left.size() == 0 || right.size() == 0) {for (int i = 0; i < right.size(); ++i) {left.emplace_back(right[i]);}node->class_value_left = node->class_value_right = to_terminal(left);return;}// check for max depthif (depth >= max_depth) {node->class_value_left = to_terminal(left);node->class_value_right = to_terminal(right);return;}// process left childif (left.size() <= min_size) {node->class_value_left = to_terminal(left);} else {dictionary dict = get_split(left);node->left = new binary_tree;node->left->dict = dict;split(node->left, depth+1);}// process right childif (right.size() <= min_size) {node->class_value_right = to_terminal(right);} else {dictionary dict = get_split(right);node->right = new binary_tree;node->right->dict = dict;split(node->right, depth+1);}
}template<typename T>
void DecisionTree<T>::build_tree(const std::vector<std::vector<T>>& train)
{// create root nodedictionary root = get_split(train);binary_tree* node = new binary_tree;node->dict = root;tree = node;split(node, 1);
}template<typename T>
void DecisionTree<T>::train()
{this->max_nodes = (1 << max_depth) - 1;build_tree(src_data);accuracy_metric();//binary_tree* tmp = tree;//print_tree(tmp);
}template<typename T>
T DecisionTree<T>::predict(const std::vector<T>& data) const
{if (!tree) {fprintf(stderr, "Error, tree is null\n");return -1111.f;}return predict(tree, data);
}template<typename T>
T DecisionTree<T>::predict(binary_tree* node, const std::vector<T>& data) const
{if (data[std::get<0>(node->dict)] < std::get<1>(node->dict)) {if (node->left) {return predict(node->left, data);} else {return node->class_value_left;}} else {if (node->right) {return predict(node->right, data);} else {return node->class_value_right;}}
}template<typename T>
int DecisionTree<T>::save_model(const char* name) const
{std::ofstream file(name, std::ios::out);if (!file.is_open()) {fprintf(stderr, "open file fail: %s\n", name);return -1;}file<<max_depth<<","<<min_size<<std::endl;binary_tree* tmp = tree;int depth = height_of_tree(tmp);CHECK(max_depth == depth);tmp = tree;write_node(tmp, file);file.close();return 0;
}template<typename T>
void DecisionTree<T>::write_node(const binary_tree* node, std::ofstream& file) const
{/*if (!node) return;write_node(node->left, file);file<<std::get<0>(node->dict)<<","<<std::get<1>(node->dict)<<","<<node->class_value_left<<","<<node->class_value_right<<std::endl;write_node(node->right, file);*///typedef std::tuple<int, int, T, T, T> row; // flag, index, value, class_value_left, class_value_rightstd::vector<row_element> vec(this->max_nodes, std::make_tuple(-1, -1, (T)-1.f, (T)-1.f, (T)-1.f));binary_tree* tmp = const_cast<binary_tree*>(node);node_to_row_element(tmp, vec, 0);for (const auto& row : vec) {file<<std::get<0>(row)<<","<<std::get<1>(row)<<","<<std::get<2>(row)<<","<<std::get<3>(row)<<","<<std::get<4>(row)<<std::endl;}
}template<typename T>
void DecisionTree<T>::node_to_row_element(binary_tree* node, std::vector<row_element>& rows, int pos) const
{if (!node) return;rows[pos] = std::make_tuple(0, std::get<0>(node->dict), std::get<1>(node->dict), node->class_value_left, node->class_value_right); // 0: have node, -1: no nodeif (node->left) node_to_row_element(node->left, rows, 2*pos+1);if (node->right) node_to_row_element(node->right, rows, 2*pos+2);
}template<typename T>
int DecisionTree<T>::height_of_tree(const binary_tree* node) const
{if (!node)return 0;elsereturn std::max(height_of_tree(node->left), height_of_tree(node->right)) + 1;
}template<typename T>
int DecisionTree<T>::load_model(const char* name)
{std::ifstream file(name, std::ios::in);if (!file.is_open()) {fprintf(stderr, "open file fail: %s\n", name);return -1;}std::string line, cell;std::getline(file, line);std::stringstream line_stream(line);std::vector<int> vec;int count = 0;while (std::getline(line_stream, cell, ',')) {vec.emplace_back(std::stoi(cell));}CHECK(vec.size() == 2);max_depth = vec[0];min_size = vec[1];max_nodes = (1 << max_depth) - 1;std::vector<row_element> rows(max_nodes);if (typeid(float).name() == typeid(T).name()) {while (std::getline(file, line)) {std::stringstream line_stream2(line);std::vector<T> vec2;while(std::getline(line_stream2, cell, ',')) {vec2.emplace_back(std::stof(cell));}CHECK(vec2.size() == 5);rows[count] = std::make_tuple((int)vec2[0], (int)vec2[1], vec2[2], vec2[3], vec2[4]);//fprintf(stderr, "%d, %d, %f, %f, %f\n", std::get<0>(rows[count]), std::get<1>(rows[count]), std::get<2>(rows[count]), std::get<3>(rows[count]), std::get<4>(rows[count]));++count;}} else { // doublewhile (std::getline(file, line)) {std::stringstream line_stream2(line);std::vector<T> vec2;while(std::getline(line_stream2, cell, ',')) {vec2.emplace_back(std::stod(cell));}CHECK(vec2.size() == 5);rows[count] = std::make_tuple((int)vec2[0], (int)vec2[1], vec2[2], vec2[3], vec[4]);++count;}}CHECK(max_nodes == count);CHECK(std::get<0>(rows[0]) != -1);binary_tree* tmp = new binary_tree;std::vector<std::vector<std::vector<T>>> dump;tmp->dict = std::make_tuple(std::get<1>(rows[0]), std::get<2>(rows[0]), dump);tmp->class_value_left = std::get<3>(rows[0]);tmp->class_value_right = std::get<4>(rows[0]);tree = tmp;row_element_to_node(tmp, rows, max_nodes, 0);file.close();return 0;
}template<typename T>
void DecisionTree<T>::row_element_to_node(binary_tree* node, const std::vector<row_element>& rows, int n, int pos)
{if (!node || n == 0) return;int new_pos = 2 * pos + 1;if (new_pos < n && std::get<0>(rows[new_pos]) != -1) {node->left = new binary_tree;std::vector<std::vector<std::vector<T>>> dump;node->left->dict = std::make_tuple(std::get<1>(rows[new_pos]), std::get<2>(rows[new_pos]), dump);node->left->class_value_left = std::get<3>(rows[new_pos]);node->left->class_value_right = std::get<4>(rows[new_pos]);row_element_to_node(node->left, rows, n, new_pos);}new_pos = 2 * pos + 2;if (new_pos < n && std::get<0>(rows[new_pos]) != -1) {node->right = new binary_tree;std::vector<std::vector<std::vector<T>>> dump;node->right->dict = std::make_tuple(std::get<1>(rows[new_pos]), std::get<2>(rows[new_pos]), dump);node->right->class_value_left = std::get<3>(rows[new_pos]);node->right->class_value_right = std::get<4>(rows[new_pos]);row_element_to_node(node->right, rows, n, new_pos);}
}template<typename T>
void DecisionTree<T>::delete_tree()
{delete_node(tree);
}template<typename T>
void DecisionTree<T>::delete_node(binary_tree* node)
{if (node->left) delete_node(node->left);if (node->right) delete_node(node->right);delete node;
}template<typename T>
double DecisionTree<T>::accuracy_metric() const
{int correct = 0;for (int i = 0; i < this->samples_num; ++i) {T predicted = predict(tree, src_data[i]);if (predicted == src_data[i][this->feature_length])++correct;}double accuracy = correct / (double)samples_num * 100.;fprintf(stdout, "train accuracy: %f\n", accuracy);return accuracy;
}template<typename T>
void DecisionTree<T>::print_tree(const binary_tree* node, int depth) const
{if (node) {std::string blank = " ";for (int i = 0; i < depth; ++i) blank += blank;fprintf(stdout, "%s[X%d < %.3f]\n", blank.c_str(), std::get<0>(node->dict)+1, std::get<1>(node->dict));if (!node->left || !node->right)blank += blank;if (!node->left)fprintf(stdout, "%s[%.1f]\n", blank.c_str(), node->class_value_left);else print_tree(node->left, depth+1);if (!node->right)fprintf(stdout, "%s[%.1f]\n", blank.c_str(), node->class_value_right);elseprint_tree(node->right, depth+1);}
}template class DecisionTree<float>;
template class DecisionTree<double>;} // namespace ANN
对外提供两个接口,一个是test_decision_tree_train用于训练,一个是test_decision_tree_predict用于测试,其code如下:
// =============================== decision tree ==============================
int test_decision_tree_train()
{// small dataset test/*const std::vector<std::vector<float>> data{ { 2.771244718f, 1.784783929f, 0.f },{ 1.728571309f, 1.169761413f, 0.f },{ 3.678319846f, 2.81281357f, 0.f },{ 3.961043357f, 2.61995032f, 0.f },{ 2.999208922f, 2.209014212f, 0.f },{ 7.497545867f, 3.162953546f, 1.f },{ 9.00220326f, 3.339047188f, 1.f },{ 7.444542326f, 0.476683375f, 1.f },{ 10.12493903f, 3.234550982f, 1.f },{ 6.642287351f, 3.319983761f, 1.f } };const std::vector<float> classes{ 0.f, 1.f };ANN::DecisionTree<float> dt;dt.init(data, classes);dt.set_max_depth(3);dt.set_min_size(1);dt.train();
#ifdef _MSC_VERconst char* model_name = "E:/GitCode/NN_Test/data/decision_tree.model";
#elseconst char* model_name = "data/decision_tree.model";
#endifdt.save_model(model_name);ANN::DecisionTree<float> dt2;dt2.load_model(model_name);const std::vector<std::vector<float>> test{{0.6f, 1.9f, 0.f}, {9.7f, 4.3f, 1.f}};for (const auto& row : test) {float ret = dt2.predict(row);fprintf(stdout, "predict result: %.1f, actural value: %.1f\n", ret, row[2]);} */// banknote authentication dataset
#ifdef _MSC_VERconst char* file_name = "E:/GitCode/NN_Test/data/database/BacknoteDataset/data_banknote_authentication.txt";
#elseconst char* file_name = "data/database/BacknoteDataset/data_banknote_authentication.txt";
#endifstd::vector<std::vector<float>> data;int ret = read_txt_file<float>(file_name, data, ',', 1372, 5);if (ret != 0) {fprintf(stderr, "parse txt file fail: %s\n", file_name);return -1;}//fprintf(stdout, "data size: rows: %d\n", data.size());const std::vector<float> classes{ 0.f, 1.f };ANN::DecisionTree<float> dt;dt.init(data, classes);dt.set_max_depth(6);dt.set_min_size(10);dt.train();
#ifdef _MSC_VERconst char* model_name = "E:/GitCode/NN_Test/data/decision_tree.model";
#elseconst char* model_name = "data/decision_tree.model";
#endifdt.save_model(model_name);return 0;
}int test_decision_tree_predict()
{
#ifdef _MSC_VERconst char* model_name = "E:/GitCode/NN_Test/data/decision_tree.model";
#elseconst char* model_name = "data/decision_tree.model";
#endifANN::DecisionTree<float> dt;dt.load_model(model_name);int max_depth = dt.get_max_depth();int min_size = dt.get_min_size();fprintf(stdout, "max_depth: %d, min_size: %d\n", max_depth, min_size);std::vector<std::vector<float>> test {{-2.5526,-7.3625,6.9255,-0.66811,1},{-4.5531,-12.5854,15.4417,-1.4983,1},{4.0948,-2.9674,2.3689,0.75429,0},{-1.0401,9.3987,0.85998,-5.3336,0},{1.0637,3.6957,-4.1594,-1.9379,1}};for (const auto& row : test) { float ret = dt.predict(row);fprintf(stdout, "predict result: %.1f, actual value: %.1f\n", ret, row[4]);}return 0;
}
训练接口执行结果如下:
测试接口执行结果如下:
训练时生成的模型decison_tree.model内容如下:
6,10
0,0,0.3223,-1,-1
0,1,7.6274,-1,-1
0,2,-4.3839,-1,-1
0,0,-0.39816,-1,-1
0,0,-4.2859,-1,-1
0,0,4.2164,-1,0
0,0,1.594,-1,-1
0,2,6.2204,-1,-1
0,1,5.8974,-1,-1
0,0,-5.4901,-1,1
0,0,-1.5768,-1,-1
0,0,0.47368,1,-1
-1,-1,-1,-1,-1
0,2,-2.2718,-1,-1
0,0,2.0421,-1,-1
0,1,7.3273,-1,1
0,1,-4.6062,-1,-1
0,2,3.1143,-1,-1
0,0,0.049175,0,0
0,0,-6.2003,1,1
-1,-1,-1,-1,-1
0,0,-2.7419,0,-1
0,0,-1.5768,0,0
-1,-1,-1,-1,-1
0,0,0.47368,1,1
-1,-1,-1,-1,-1
-1,-1,-1,-1,-1
0,1,7.6377,-1,0
0,3,0.097399,-1,-1
0,2,-2.3386,1,-1
0,0,3.6216,-1,-1
0,0,-1.3971,1,1
-1,-1,-1,-1,-1
0,0,-1.6677,1,1
0,0,-1.7781,0,0
0,0,-0.36506,1,1
0,3,1.547,0,1
-1,-1,-1,-1,-1
-1,-1,-1,-1,-1
-1,-1,-1,-1,-1
-1,-1,-1,-1,-1
-1,-1,-1,-1,-1
-1,-1,-1,-1,-1
-1,-1,-1,-1,-1
0,0,-2.7419,0,0
-1,-1,-1,-1,-1
-1,-1,-1,-1,-1
-1,-1,-1,-1,-1
-1,-1,-1,-1,-1
-1,-1,-1,-1,-1
-1,-1,-1,-1,-1
-1,-1,-1,-1,-1
-1,-1,-1,-1,-1
-1,-1,-1,-1,-1
-1,-1,-1,-1,-1
0,0,1.0552,1,1
-1,-1,-1,-1,-1
0,0,0.4339,0,0
0,2,2.0013,1,0
-1,-1,-1,-1,-1
0,0,1.8993,0,0
0,0,3.4566,0,0
0,0,3.6216,0,0
GitHub: https://github.com/fengbingchun/NN_Test
相关文章:

iOS开发-由浅至深学习block
作者:Sindri的小巢(简书) 关于block 在iOS 4.0之后,block横空出世,它本身封装了一段代码并将这段代码当做变量,通过block()的方式进行回调。这不免让我们想到在C函数中,我们可以定义一个指向函数…
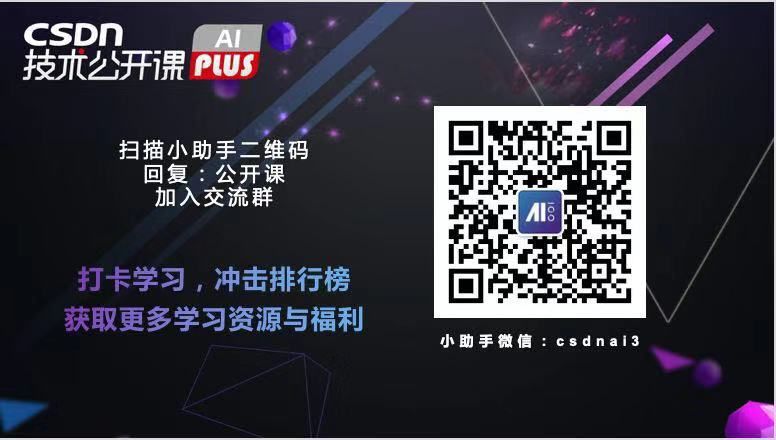
Google和微软分别提出分布式深度学习训练新框架:GPipe PipeDream
【进群了解最新免费公开课、技术沙龙信息】作者 | Jesus Rodriguez译者 | 陆离编辑 | Jane出品 | AI科技大本营(ID:rgznai100)【导读】微软和谷歌一直在致力于开发新的用于训练深度神经网络的模型,最近,谷歌和微软分别…

fragment 横竖屏 不重建
2019独角兽企业重金招聘Python工程师标准>>> android:configChanges"screenSize|orientation" 这样设置 切屏时都不会重新调用fragment里面的onCreateView了 转载于:https://my.oschina.net/u/1777508/blog/317811
二叉树简介及C++实现
二叉树是每个结点最多有两个子树的树结构,即结点的度最大为2。通常子树被称作”左子树”和”右子树”。二叉树是一个连通的无环图。 二叉树是递归定义的,其结点有左右子树之分,逻辑上二叉树有五种基本形态:(1)、空二叉树…

swift实现ios类似微信输入框跟随键盘弹出的效果
为什么要做这个效果 在聊天app,例如微信中,你会注意到一个效果,就是在你点击输入框时输入框会跟随键盘一起向上弹出,当你点击其他地方时,输入框又会跟随键盘一起向下收回,二者完全无缝连接,那么…
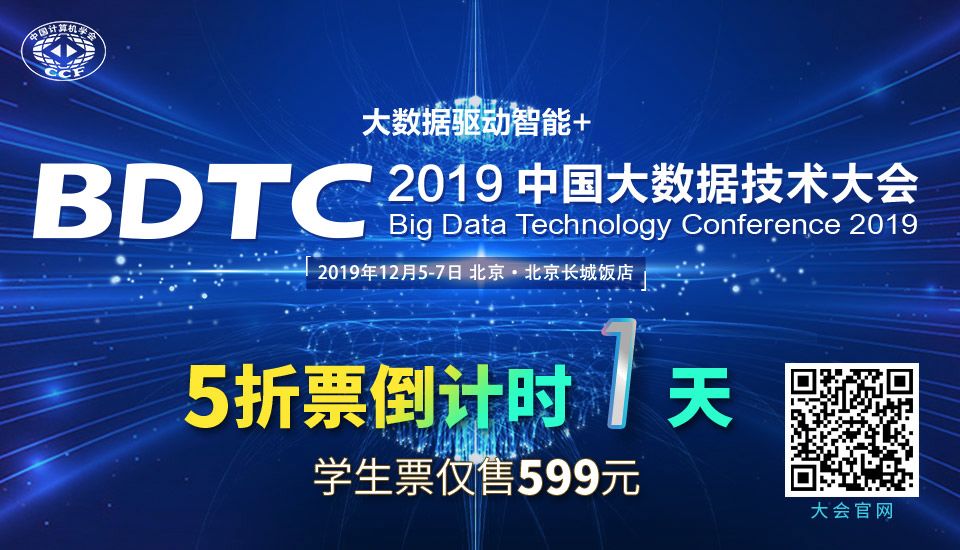
行人被遮挡问题怎么破?百度提出PGFA新方法,发布Occluded-DukeMTMC大型数据集 | ICCV 2019...
作者 | Jiaxu Miao、Yu Wu、Ping Liu、Yuhang Ding、Yi Yang译者 | 刘畅编辑 | Jane出品 | AI科技大本营(ID:rgznai100)【导语】在以人搜人的场景中,行人会经常被各种物体遮挡。之前的行人再识别(re-id)方法…

WinAPI: Arc - 绘制弧线
为什么80%的码农都做不了架构师?>>> //声明: Arc(DC: HDC; {设备环境句柄}X1, Y1, X2, Y2, X3, Y3, X4, Y4: Integer {四个坐标点} ): BOOL;//举例: procedure TForm1.FormPaint(Sender: TObject); constx1 10;y1 10;…

提高C++性能的编程技术笔记:跟踪实例+测试代码
当提高性能时,我们必须记住以下几点: (1). 内存不是无限大的。虚拟内存系统使得内存看起来是无限的,而事实上并非如此。 (2). 内存访问开销不是均衡的。对缓存、主内存和磁盘的访问开销不在同一个数量级之上。 (3). 我们的程序没有专用的CPUÿ…
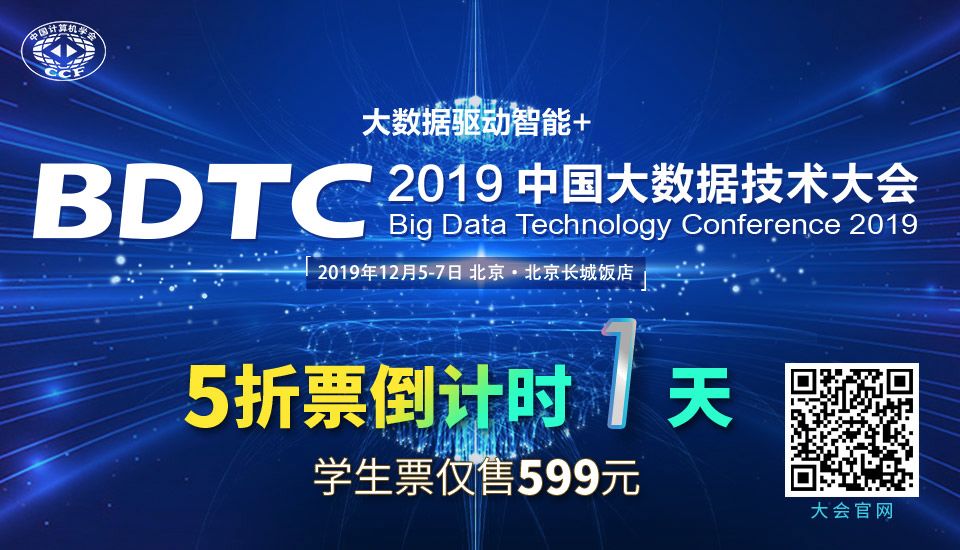
2019年不可错过的45个AI开源工具,你想要的都在这里
整理 | Jane 出品 | AI科技大本营(ID:rgznai100)一个好工具,能提高开发效率,优化项目研发过程,无论是企业还是开发者个人都在寻求适合自己的开发工具。但是,选择正确的工具并不容易,有时这甚至是…

swift中delegate与block的反向传值
swift.jpg入门级 此处只简单举例并不深究,深究我也深究不来。对于初学者来说delegate或block都不是一下子能理解的,所以我的建议和体会就是,理不理解咱先不说,我先把这个格式记住,对就是格式,delegate或blo…

Direct2D (15) : 剪辑
为什么80%的码农都做不了架构师?>>> 绘制在 RenderTarget.PushAxisAlignedClip() 与 RenderTarget.PopAxisAlignedClip() 之间的内容将被指定的矩形剪辑。 uses Direct2D, D2D1;procedure TForm1.FormPaint(Sender: TObject); varcvs: TDirect2DCanvas;…
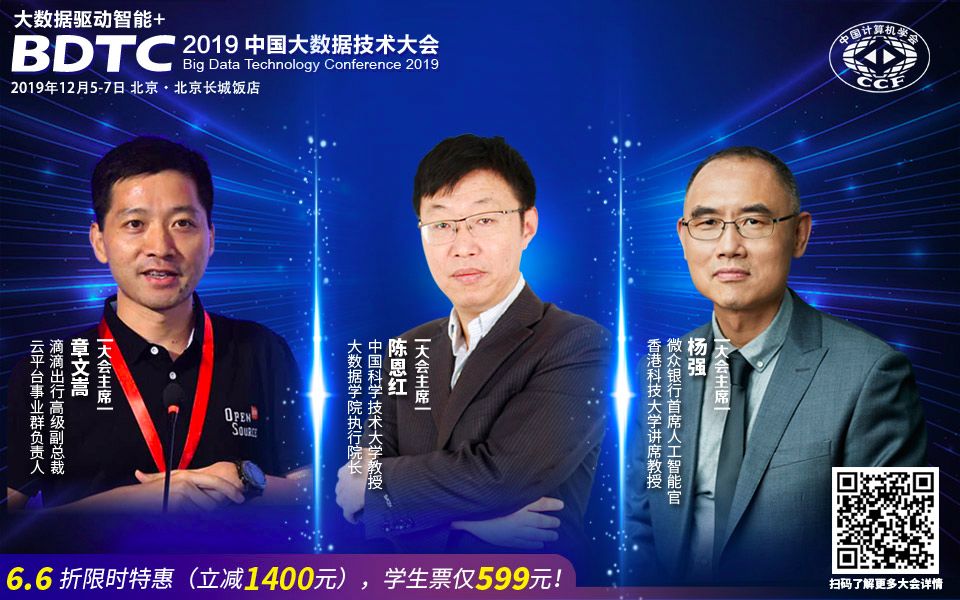
女朋友啥时候怒了?Keras识别面部表情挽救你的膝盖
作者 | 叶圣出品 | AI科技大本营(ID:rgznai100)【导读】随着计算机和AI新技术及其涉及自然科学的飞速发展,整个社会上的管理系统高度大大提升,人们对类似人与人之间的交流日渐疲劳而希望有机器的理解。计算机系统和机械人如果需要…
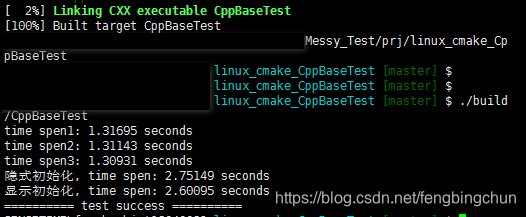
提高C++性能的编程技术笔记:构造函数和析构函数+测试代码
对象的创建和销毁往往会造成性能的损失。在继承层次中,对象的创建将引起其先辈的创建。对象的销毁也是如此。其次,对象相关的开销与对象本身的派生链的长度和复杂性相关。所创建的对象(以及其后销毁的对象)的数量与派生的复杂度成正比。 并不是说继承根…
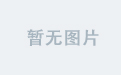
swim 中一行代码解决收回键盘
//点击空白收回键盘 override func touchesBegan(touches: Set<UITouch>, withEvent event: UIEvent?) { view.endEditing(true) }

WinAPI: SetRect 及初始化矩形的几种办法
为什么80%的码农都做不了架构师?>>> 本例分别用五种办法初始化了同样的一个矩形, 运行效果图: unit Unit1;interfaceusesWindows, Messages, SysUtils, Variants, Classes, Graphics, Controls, Forms,Dialogs, StdCtrls;typeTForm1 class(TForm)Butto…
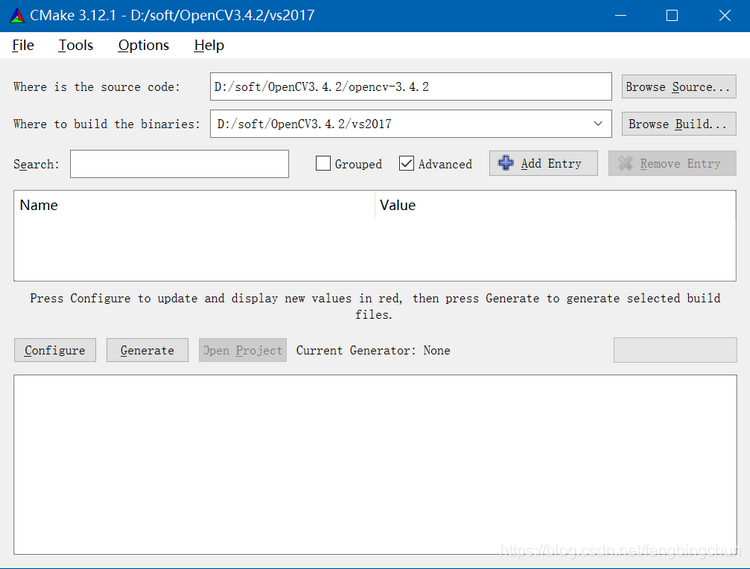
Windows10上使用VS2017编译OpenCV3.4.2+OpenCV_Contrib3.4.2+Python3.6.2操作步骤
1. 从https://github.com/opencv/opencv/releases 下载opencv-3.4.2.zip并解压缩到D:\soft\OpenCV3.4.2\opencv-3.4.2目录下; 2. 从https://github.com/opencv/opencv_contrib/releases 下载opencv_contrib-3.4.zip并解压缩到D:\soft\OpenCV3.4.2\opencv_contrib-3…
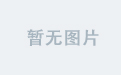
swift 跳转网页写法
var alert : UIAlertView UIAlertView.init(title: "公安出入境网上办事平台", message: "目前您可以使用网页版进行出入境业务预约与查询,是否进入公安出入境办事平台?", delegate: nil, cancelButtonTitle: "取消", o…
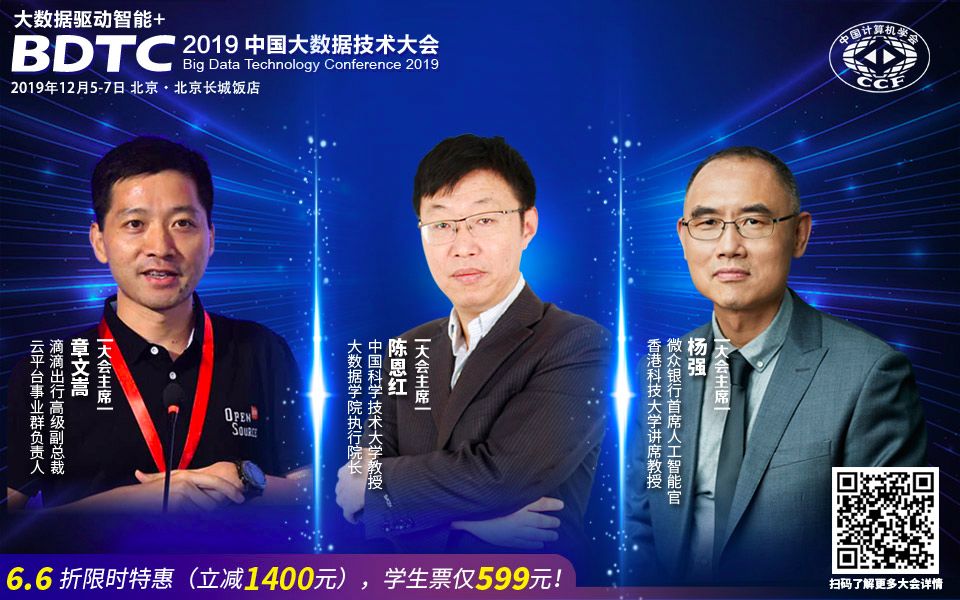
智能边缘计算:计算模式的再次轮回
作者 | 刘云新来源 | 微软研究院AI头条(ID:MSRAsia)【导读】人工智能的蓬勃发展离不开云计算所带来的强大算力,然而随着物联网以及硬件的快速发展,边缘计算正受到越来越多的关注。未来,智能边缘计算将与智能云计算互为…

WinAPI: 钩子回调函数之 SysMsgFilterProc
为什么80%的码农都做不了架构师?>>> SysMsgFilterProc(nCode: Integer; {}wParam: WPARAM; {}lParam: LPARAM {} ): LRESULT; {}//待续...转载于:https://my.oschina.net/hermer/blog/319736

提高C++性能的编程技术笔记:虚函数、返回值优化+测试代码
虚函数:在以下几个方面,虚函数可能会造成性能损失:构造函数必须初始化vptr(虚函数表);虚函数是通过指针间接调用的,所以必须先得到指向虚函数表的指针,然后再获得正确的函数偏移量;内联是在编译…
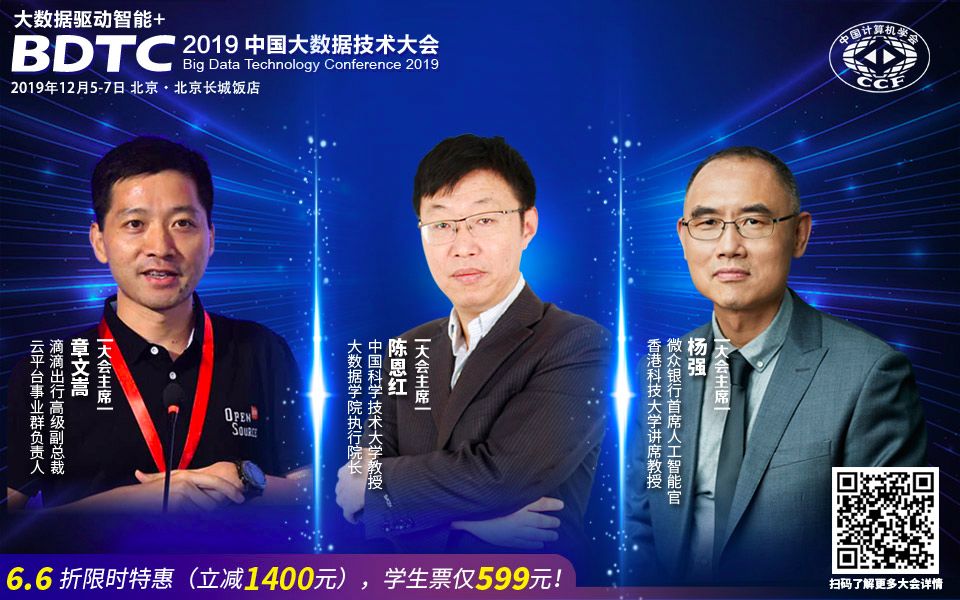
ICCV 2019 | 无需数据集的Student Networks
译者 | 李杰 出品 | AI科技大本营(ID:rgznai100)本文是华为诺亚方舟实验室联合北京大学和悉尼大学在ICCV2019的工作。摘要在计算机视觉任务中,为了将预训练的深度神经网络模型应用到各种移动设备上,学习一个轻便的网络越来越重要。…
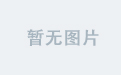
oc中特殊字符的判断方法
-(BOOL)isSpacesExists { // NSString *_string [NSString stringWithFormat:"123 456"]; NSRange _range [self rangeOfString:" "]; if (_range.location ! NSNotFound) { //有空格 return YES; }else { //没有空格 return NO; } } -(BOOL)i…

理解 Delphi 的类(十) - 深入方法[23] - 重载
为什么80%的码农都做不了架构师?>>> {下面的函数重名, 但参数不一样, 此类情况必须加 overload 指示字;调用时, 会根据参数的类型和个数来决定调用哪一个;这就是重载. }function MyFun(s: string): string; overload; beginResult : 参数是一个字符串: …

玩转ios友盟远程推送,16年5月图文防坑版
最近有个程序员妹子在做远程推送的时候遇到了困难,求助本帅。尽管本帅也是多彩的绘图工具,从没做过远程推送,但是本着互相帮助,共同进步的原则,本帅还是掩饰了自己的彩笔身份,耗时三天(休息时间…
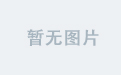
提高C++性能的编程技术笔记:临时对象+测试代码
类型不匹配:一般情况是指当需要X类型的对象时提供的却是其它类型的对象。编译器需要以某种方式将提供的类型转换成要求的X类型。这一过程可能会产生临时对象。 按值传递:创建和销毁临时对象的代价是比较高的。倘若可以,我们应该按指针或者引…
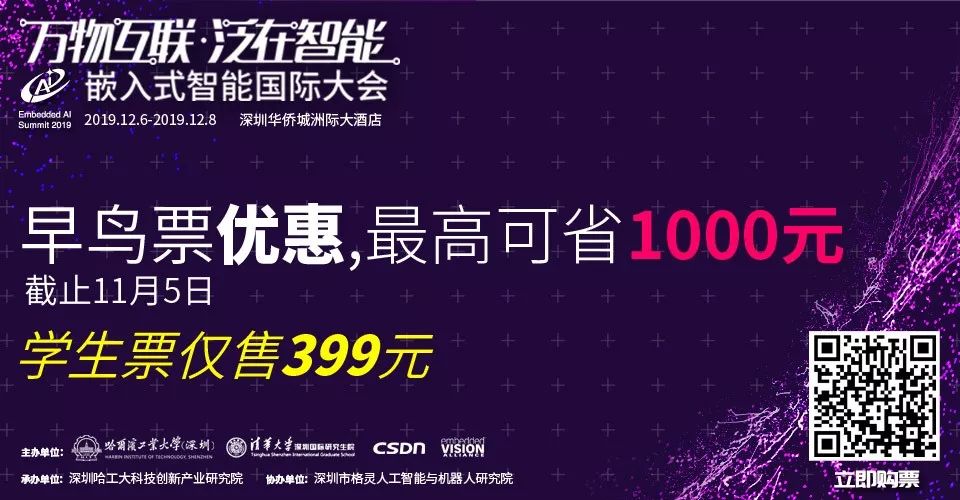
北美欧洲顶级大咖齐聚,在这里读懂 AIoT 未来!
2019 嵌入式智能国际大会即将来袭!购票官网:https://dwz.cn/z1jHouwE随着海量移动设备的时代到来,以传统数据中心运行的人工智能计算正在受到前所未有的挑战。在这一背景下,聚焦于在远离数据中心的互联网边缘进行人工智能运算的「…
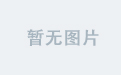
c# 关闭软件 进程 杀死进程
c# 关闭软件 进程 杀死进程 foreach (System.Diagnostics.Process p in System.Diagnostics.Process.GetProcessesByName("Server")){p.Kill();} 转载于:https://www.cnblogs.com/lxctboy/p/3999053.html

提高C++性能的编程技术笔记:单线程内存池+测试代码
频繁地分配和回收内存会严重地降低程序的性能。性能降低的原因在于默认的内存管理是通用的。应用程序可能会以某种特定的方式使用内存,并且为不需要的功能付出性能上的代价。通过开发专用的内存管理器可以解决这个问题。对专用内存管理器的设计可以从多个角度考虑。…

【Swift】 GETPOST请求 网络缓存的简单处理
GET & POST 的对比 源码: https://github.com/SpongeBob-GitHub/Get-Post.git 1. URL - GET 所有的参数都包含在 URL 中 1. 如果需要添加参数,脚本后面使用 ? 2. 参数格式:值对 参数名值 3. 如果有多个参数,使用 & 连接 …
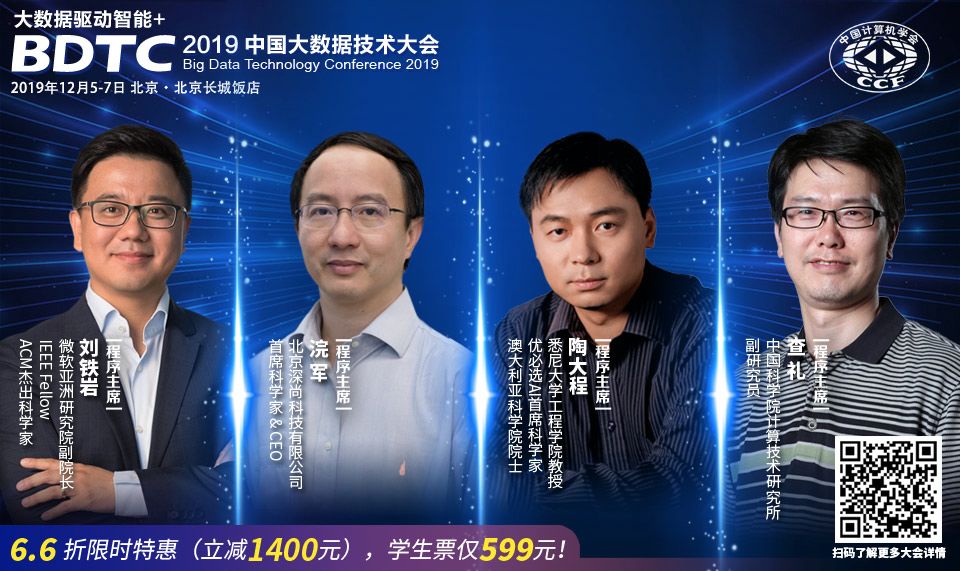
深度CTR预估模型的演化之路2019最新进展
作者 | 锅逗逗来源 | 深度传送门(ID: deep_deliver)导读:本文主要介绍深度CTR经典预估模型的演化之路以及在2019工业界的最新进展。介绍在计算广告和推荐系统中,点击率(Click Through Rate,以下简称CTR&…