使用深度学习阅读和分类扫描文档
作者|小白
来源|小白学视觉
收集数据
首先,我们要做的第一件事是创建一个简单的数据集,这样我们就可以测试我们工作流程的每一部分。理想情况下,我们的数据集将包含各种易读性和时间段的扫描文档,以及每个文档所属的高级主题。我找不到具有这些精确规格的数据集,所以我开始构建自己的数据集。我决定的高层次话题是政府、信件、吸烟和专利,随机的选择这些主要是因为每个地区都有各种各样的扫描文件。
我从这些来源中的每一个中挑选了 20 个左右的大小合适的文档,并将它们放入由主题定义的单独文件夹中。
经过将近一整天的搜索和编目所有图像后,我们将它们全部调整为 600x800 并将它们转换为 PNG 格式。
简单的调整大小和转换脚本如下:
from PIL import Imageimg_folder = r'F:\Data\Imagery\OCR' # Folder containing topic folders (i.e "News", "Letters" ..etc.)for subfol in os.listdir(img_folder): # For each of the topic folderssfpath = os.path.join(img_folder, subfol)
for imgfile in os.listdir(sfpath): # Get all images in the topicimgpath = os.path.join(sfpath, imgfile)img = Image.open(imgpath) # Read in the image with Pillowimg = img.resize((600,800)) # Resize the imagenewip = imgpath[0:-4] + ".png" # Convert to PNGimg.save(newip) # Save
构建OCR管道
光学字符识别是从图像中提取文字的过程。这通常是通过机器学习模型完成的,最常见的是通过包含卷积神经网络的管道来完成。虽然我们可以为我们的应用程序训练自定义 OCR 模型,但它需要更多的训练数据和计算资源。相反,我们将使用出色的 Microsoft 计算机视觉 API,其中包括专门用于 OCR 的特定模块。API 调用将使用图像(作为 PIL 图像)并输出几位信息,包括图像上文本的位置/方向作为以及文本本身。以下函数将接收一个 PIL 图像列表并输出一个大小相等的提取文本列表:
def image_to_text(imglist, ndocs=10):
'''Take in a list of PIL images and return a list of extracted text using OCR'''headers = {
# Request headers
'Content-Type': 'application/octet-stream',
'Ocp-Apim-Subscription-Key': 'YOUR_KEY_HERE',}params = urllib.parse.urlencode({
# Request parameters
'language': 'en',
'detectOrientation ': 'true',})outtext = []docnum = 0for cropped_image in imglist:print("Processing document -- ", str(docnum))
# Cropped image must have both height and width > 50 px to run Computer Vision API
#if (cropped_image.height or cropped_image.width) < 50:
# cropped_images_ocr.append("N/A")
# continueocr_image = cropped_imageimgByteArr = io.BytesIO()ocr_image.save(imgByteArr, format='PNG')imgByteArr = imgByteArr.getvalue()try:conn = http.client.HTTPSConnection('westus.api.cognitive.microsoft.com')conn.request("POST", "/vision/v1.0/ocr?%s" % params, imgByteArr, headers)response = conn.getresponse()data = json.loads(response.read().decode("utf-8"))curr_text = []
for r in data['regions']:
for l in r['lines']:
for w in l['words']:curr_text.append(str(w['text']))conn.close()except Exception as e:print("Could not process imageouttext.append(' '.join(curr_text))docnum += 1return(outtext)
后期处理
由于在某些情况下我们可能希望在这里结束我们的工作流程,而不是仅仅将提取的文本作为一个巨大的列表保存在内存中,我们还可以将提取的文本写入与原始输入文件同名的单个 txt 文件中。微软的OCR技术虽然不错,但偶尔也会出错。我们可以使用 SpellChecker 模块减少其中的一些错误,以下脚本接受输入和输出文件夹,读取输入文件夹中的所有扫描文档,使用我们的 OCR 脚本读取它们,运行拼写检查并纠正拼写错误的单词,最后将原始txt文件导出目录。
'''
Read in a list of scanned images (as .png files > 50x50px) and output a set of .txt files containing the text content of these scans
'''from functions import preprocess, image_to_text
from PIL import Image
import os
from spellchecker import SpellChecker
import matplotlib.pyplot as pltINPUT_FOLDER = r'F:\Data\Imagery\OCR2\Images'
OUTPUT_FOLDER = r'F:\Research\OCR\Outputs\AllDocuments'## First, read in all the scanned document images into PIL images
scanned_docs_path = os.listdir(INPUT_FOLDER)
scanned_docs_path = [x for x in scanned_docs_path if x.endswith('.png')]
scanned_docs = [Image.open(os.path.join(INPUT_FOLDER, path)) for path in scanned_docs_path]## Second, utilize Microsoft CV API to extract text from these images using OCR
scanned_docs_text = image_to_text(scanned_docs)## Third, remove mis-spellings that might have occured from bad OCR readings
spell = SpellChecker()
for i in range(len(scanned_docs_text)):clean = scanned_docs_text[i]misspelled = spell.unknown(clean)clean = clean.split(" ")
for word in range(len(clean)):
if clean[word] in misspelled:clean[word] = spell.correction(clean[word])# Get the one `most likely` answerclean = ' '.join(clean)scanned_docs_text[i] = clean## Fourth, write the extracted text to individual .txt files with the same name as input files
for k in range(len(scanned_docs_text)): # For each scanned documenttext = scanned_docs_text[k]path = scanned_docs_path[k] # Get the corresponding input filenametext_file_path = path[:-4] + ".txt" # Create the output text filetext_file = open(text_file_path, "wt")n = text_file.write(text) # Write the text to the ouput text filetext_file.close()print("Done")
为建模准备文本
如果我们的扫描文档集足够大,将它们全部写入一个大文件夹会使它们难以分类,并且我们可能已经在文档中进行了某种隐式分组。如果我们大致了解我们拥有多少种不同的“类型”或文档主题,我们可以使用主题建模来帮助自动识别这些。这将为我们提供基础架构,以根据文档内容将 OCR 中识别的文本拆分为单独的文件夹,我们将使用该主题模型被称为LDA。为了运行这个模型,我们需要对我们的数据进行更多的预处理和组织,因此为了防止我们的脚本变得冗长和拥挤,我们将假设已经使用上述工作流程读取了扫描的文档并将其转换为 txt 文件. 然后主题模型将读入这些 txt 文件,将它们分类到我们指定的任意多个主题中,并将它们放入适当的文件夹中。
我们将从一个简单的函数开始,读取文件夹中所有输出的 txt 文件,并将它们读入包含 (filename, text) 的元组列表。
def read_and_return(foldername, fileext='.txt'):
'''Read all text files with fileext from foldername, and place them into a list of tuples as[(filename, text), ... , (filename, text)]'''allfiles = os.listdir(foldername)allfiles = [os.path.join(foldername, f) for f in allfiles if f.endswith(fileext)]alltext = []
for filename in allfiles:
with open(filename, 'r') as f:alltext.append((filename, f.read()))f.close()
return(alltext) # Returns list of tuples [(filename, text), ... (filename,text)]
接下来,我们需要确保所有无用的词(那些不能帮助我们区分特定文档主题的词)。我们将使用三种不同的方法来做到这一点:
删除停用词
去除标签、标点、数字和多个空格
TF-IDF 过滤
为了实现所有这些(以及我们的主题模型),我们将使用 Gensim 包。下面的脚本将对文本列表(上述函数的输出)运行必要的预处理步骤并训练 LDA 模型。
from gensim import corpora, models, similarities
from gensim.parsing.preprocessing import remove_stopwords, preprocess_stringdef preprocess(document):clean = remove_stopwords(document)clean = preprocess_string(document)
return(clean)def run_lda(textlist, num_topics=10,preprocess_docs=True):'''Train and return an LDA model against a list of documents'''
if preprocess_docs:doc_text = [preprocess(d) for d in textlist]dictionary = corpora.Dictionary(doc_text)corpus = [dictionary.doc2bow(text) for text in doc_text]tfidf = models.tfidfmodel.TfidfModel(corpus)transformed_tfidf = tfidf[corpus]lda = models.ldamulticore.LdaMulticore(transformed_tfidf, num_topics=num_topics, id2word=dictionary)return(lda, dictionary)
使用模型对文档进行分类
一旦我们训练了我们的 LDA 模型,我们就可以使用它来将我们的训练文档集(以及可能出现的未来文档)分类为主题,然后将它们放入适当的文件夹中。
对新的文本字符串使用经过训练的 LDA 模型需要一些麻烦,所有的复杂性都包含在下面的函数中:
def find_topic(textlist, dictionary, lda):
'''https://stackoverflow.com/questions/16262016/how-to-predict-the-topic-of-a-new-query-using-a-trained-lda-model-using-gensimFor each query ( document in the test file) , tokenize the query, create a feature vector just like how it was done while trainingand create text_corpus'''text_corpus = []for query in textlist:temp_doc = tokenize(query.strip())current_doc = []temp_doc = list(temp_doc)
for word in range(len(temp_doc)):current_doc.append(temp_doc[word])text_corpus.append(current_doc)
'''For each feature vector text, lda[doc_bow] gives the topicdistribution, which can be sorted in descending order to print the very first topic'''tops = []
for text in text_corpus:doc_bow = dictionary.doc2bow(text)topics = sorted(lda[doc_bow],key=lambda x:x[1],reverse=True)[0]tops.append(topics)
return(tops)
最后,我们需要另一种方法来根据主题索引获取主题的实际名称:
def topic_label(ldamodel, topicnum):alltopics = ldamodel.show_topics(formatted=False)topic = alltopics[topicnum]topic = dict(topic[1])
return(max(topic, key=lambda key: topic[key]))
现在,我们可以将上面编写的所有函数粘贴到一个接受输入文件夹、输出文件夹和主题计数的脚本中。该脚本将读取输入文件夹中所有扫描的文档图像,将它们写入txt 文件,构建LDA 模型以查找文档中的高级主题,并根据文档主题将输出的txt 文件归类到文件夹中。
#################################################################
# This script takes in an input folder of scanned documents #
# and reads these documents, seperates them into topics #
# and outputs raw .txt files into the output folder, seperated #
# by topic #
#################################################################import os
from PIL import Image
import base64
import http.client, urllib.request, urllib.parse, urllib.error, base64
import io
import json
import requests
import urllib
from gensim import corpora, models, similarities
from gensim.utils import tokenize
from gensim.parsing.preprocessing import remove_stopwords, preprocess_string
import http
import shutil
import tqdmdef filter_for_english(text):dict_url = 'https://raw.githubusercontent.com/first20hours/' \
'google-10000-english/master/20k.txt'dict_words = set(requests.get(dict_url).text.splitlines())english_words = tokenize(text)english_words = [w for w in english_words if w in list(dict_words)]english_words = [w for w in english_words if (len(w)>1 or w.lower()=='i')]
return(' '.join(english_words))def preprocess(document):clean = filter_for_english(document)clean = remove_stopwords(clean)clean = preprocess_string(clean) # Remove non-english wordsreturn(clean)def read_and_return(foldername, fileext='.txt', delete_after_read=False):allfiles = os.listdir(foldername)allfiles = [os.path.join(foldername, f) for f in allfiles if f.endswith(fileext)]alltext = []
for filename in allfiles:
with open(filename, 'r') as f:alltext.append((filename, f.read()))f.close()
if delete_after_read:os.remove(filename)
return(alltext) # Returns list of tuples [(filename, text), ... (filename,text)]def image_to_text(imglist, ndocs=10):
'''Take in a list of PIL images and return a list of extracted text'''headers = {
# Request headers
'Content-Type': 'application/octet-stream',
'Ocp-Apim-Subscription-Key': '89279deb653049078dd18b1b116777ea',}params = urllib.parse.urlencode({
# Request parameters
'language': 'en',
'detectOrientation ': 'true',})outtext = []docnum = 0for cropped_image in tqdm.tqdm(imglist, total=len(imglist)):
# Cropped image must have both height and width > 50 px to run Computer Vision API
#if (cropped_image.height or cropped_image.width) < 50:
# cropped_images_ocr.append("N/A")
# continueocr_image = cropped_imageimgByteArr = io.BytesIO()ocr_image.save(imgByteArr, format='PNG')imgByteArr = imgByteArr.getvalue()try:conn = http.client.HTTPSConnection('westus.api.cognitive.microsoft.com')conn.request("POST", "/vision/v1.0/ocr?%s" % params, imgByteArr, headers)response = conn.getresponse()data = json.loads(response.read().decode("utf-8"))curr_text = []
for r in data['regions']:
for l in r['lines']:
for w in l['words']:curr_text.append(str(w['text']))conn.close()
except Exception as e:print("[Errno {0}] {1}".format(e.errno, e.strerror))outtext.append(' '.join(curr_text))docnum += 1return(outtext)def run_lda(textlist, num_topics=10,return_model=False,preprocess_docs=True):'''Train and return an LDA model against a list of documents'''
if preprocess_docs:doc_text = [preprocess(d) for d in textlist]dictionary = corpora.Dictionary(doc_text)corpus = [dictionary.doc2bow(text) for text in doc_text]tfidf = models.tfidfmodel.TfidfModel(corpus)transformed_tfidf = tfidf[corpus]lda = models.ldamulticore.LdaMulticore(transformed_tfidf, num_topics=num_topics, id2word=dictionary)input_doc_topics = lda.get_document_topics(corpus)return(lda, dictionary)def find_topic(text, dictionary, lda):
'''https://stackoverflow.com/questions/16262016/how-to-predict-the-topic-of-a-new-query-using-a-trained-lda-model-using-gensimFor each query ( document in the test file) , tokenize the query, create a feature vector just like how it was done while trainingand create text_corpus'''text_corpus = []for query in text:temp_doc = tokenize(query.strip())current_doc = []temp_doc = list(temp_doc)
for word in range(len(temp_doc)):current_doc.append(temp_doc[word])text_corpus.append(current_doc)
'''For each feature vector text, lda[doc_bow] gives the topicdistribution, which can be sorted in descending order to print the very first topic'''tops = []
for text in text_corpus:doc_bow = dictionary.doc2bow(text)topics = sorted(lda[doc_bow],key=lambda x:x[1],reverse=True)[0]tops.append(topics)
return(tops)def topic_label(ldamodel, topicnum):alltopics = ldamodel.show_topics(formatted=False)topic = alltopics[topicnum]topic = dict(topic[1])
import operator
return(max(topic, key=lambda key: topic[key]))INPUT_FOLDER = r'F:/Research/OCR/Outputs/AllDocuments'
OUTPUT_FOLDER = r'F:/Research/OCR/Outputs/AllDocumentsByTopic'
TOPICS = 4if __name__ == '__main__':print("Reading scanned documents")
## First, read in all the scanned document images into PIL imagesscanned_docs_fol = r'F:/Research/OCR/Outputs/AllDocuments'scanned_docs_path = os.listdir(scanned_docs_fol)scanned_docs_path = [os.path.join(scanned_docs_fol, p) for p in scanned_docs_path]scanned_docs = [Image.open(x) for x in scanned_docs_path if x.endswith('.png')]## Second, utilize Microsoft CV API to extract text from these images using OCRscanned_docs_text = image_to_text(scanned_docs)print("Post-processing extracted text")
## Third, remove mis-spellings that might have occured from bad OCR readingsspell = SpellChecker()
for i in range(len(scanned_docs_text)):clean = scanned_docs_text[i]misspelled = spell.unknown(clean)clean = clean.split(" ")
for word in range(len(clean)):
if clean[word] in misspelled:clean[word] = spell.correction(clean[word])# Get the one `most likely` answerclean = ' '.join(clean)scanned_docs_text[i] = cleanprint("Writing read text into files")
## Fourth, write the extracted text to individual .txt files with the same name as input files
for k in range(len(scanned_docs_text)): # For each scanned documenttext = scanned_docs_text[k]text = filter_for_english(text)path = scanned_docs_path[k] # Get the corresponding input filenamepath = path.split("\\")[-1]text_file_path = OUTPUT_FOLDER + "//" + path[0:-4] + ".txt" # Create the output text filetext_file = open(text_file_path, "wt")n = text_file.write(text) # Write the text to the ouput text filetext_file.close()# First, read all the output .txt filesprint("Reading files")texts = read_and_return(OUTPUT_FOLDER)print("Building LDA topic model")
# Second, train the LDA model (pre-processing is internally done)print("Preprocessing Text")textlist = [t[1] for t in texts]ldamodel, dictionary = run_lda(textlist, num_topics=TOPICS)# Third, extract the top topic for each documentprint("Extracting Topics")topics = []
for t in texts:topics.append((t[0], find_topic([t[1]], dictionary, ldamodel)))# Convert topics to topic names
for i in range(len(topics)):topnum = topics[i][1][0][0]
#print(topnum)topics[i][1][0] = topic_label(ldamodel, topnum)
# [(filename, topic), ..., (filename, topic)]# Create folders for the topicsprint("Copying Documents into Topic Folders")foundtopics = []
for t in topics:foundtopics+= t[1]foundtopics = set(foundtopics)topicfolders = [os.path.join(OUTPUT_FOLDER, f) for f in foundtopics]topicfolders = set(topicfolders)[os.makedirs(m) for m in topicfolders]# Copy files into appropriate topic folders
for t in topics:filename, topic = tsrc = filenamefilename = filename.split("\\")dest = os.path.join(OUTPUT_FOLDER, topic[0])dest = dest + "/" + filename[-1]copystr = "copy " + src + " " + destshutil.copyfile(src, dest)os.remove(src)print("Done")
本文代码Github链接:
https://github.com/ShairozS/Scan2Topic
往
期
回
顾
资讯
AI 考古比胡八一更高效
资讯
阿里云投入 20 亿发力操作系统
资讯
阿里发布云芯片倚天710
技术
ST-GCN 实现人体姿态行为分类
分享
点收藏
点点赞
点在看
相关文章:
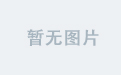
无聊的时候,冷死了(六)
阁下长得真是天生励志!好久没有听到有人能把牛吹得这么清新脱俗了!你出生时就丑的躲起来了,连你父母都不敢见你,你还怕有人举报你?你拉着一头猪逛街,很幸福的样子,我经过满怀同情的说࿱…
Java EE 开发环境搭建
下载安装Java EE SDK 版本:Java Platform,Enterprise Edition 7 SDK (with JDK 7u45) 下载页面: http://www.oracle.com/technetwork/java/javaee/downloads/java-ee-7-sdk-with-jdk-u45-2066865.html 文件名:java_ee_sdk-7-jdk7-windows.exe…
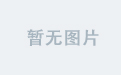
memcacheq 服务安装与原理
memcacheQ是一个单纯的分布式消息队列服务。它的安装依赖于BerkeleyDB 和 libevent,所以要先安装这BerkeleyDB和libevent: 一,BerkeleyDB 下载软件包,http://download.oracle.com/berkeley-db/db-5.0.21.tar.gz解压缩后ÿ…

AI 帮忙找 Bug ,英特尔开源代码编程工具 ControlFlag
整理 | 孙胜出品 | CSDN近日,英特尔开源了自动代码调试工具 ControlFlag 源代码,ControlFlag 源码现在可通过 GitHub 获得。据了解,ControlFlag 可用来帮助更多开发者自主检测代码错误,主要利用 AI 自动识别软件和固件代码中的错误…
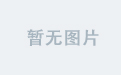
一次心惊肉跳的服务器误删文件的恢复过程
经历了两天不懈努力,终于恢复了一次误操作删除的生产服务器数据。对本次事故过程和解决办法记录在此,警醒自己,也提示别人莫犯此错。也希望遇到问题的朋友能找到一丝灵感解决问题。事故背景安排一个妹子在一台生产服务器上安装Oracle,妹子边研…
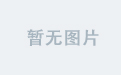
【vue】vue中ref用法
1.获取当前元素: 例子: <div class"pop pos-a" :style"{ left: pop_x px ,top: pop_y px}" ref"refName"><ul><li>编辑部门</li><li click"append()">添加子部门</li>&…
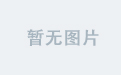
使用Gearman做分布式计算
通常,多语言多系统之间的集成是个大问题,一般来说,人们多半会采用WebService的方式来处理此类集成问题,但不管采用何种风格的WebService,如RPC风格,或者REST风格,其本身都有一定的复杂性。相比之…
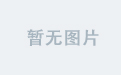
把数据库中有关枚举项值的数字字符串转换成文字字符串
原文:把数据库中有关枚举项值的数字字符串转换成文字字符串标题可能无法表达我的本意。比如,有这样一个枚举: public enum MyChoice{MyFirstChoice 0,MySecondChoice 1,MyThirdChoice 2} 数据库中,某表某字段保存值为"0,1,2"&…

又被 AI 抢饭碗?2457 亿参数规模,全球最大中文人工智能巨量模型 “源1.0”正式开源...
作者 | 伍杏玲 出品 | AI科技大本营(ID:rgznai100)输入:昔我往矣,杨柳依依。今我来思,雨雪霏霏。行道迟迟,载渴载饥。我心伤悲,莫知我哀!(以战争为题写一首诗)…

Java架构演进之路
2019独角兽企业重金招聘Python工程师标准>>> hello 转载于:https://my.oschina.net/mrpei123/blog/1605391
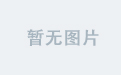
F5与NetScaler比较
F5 是基于Linux的,NetScaler 是基于BSD的。F5 的四层走的是硬件芯片,七层走的是软件,NetScaler 全部走的是软件。我测试的性能也是 F5比NetScaler强,在均不使用压缩的情况下,NetScaler比F5消耗更大的带宽。
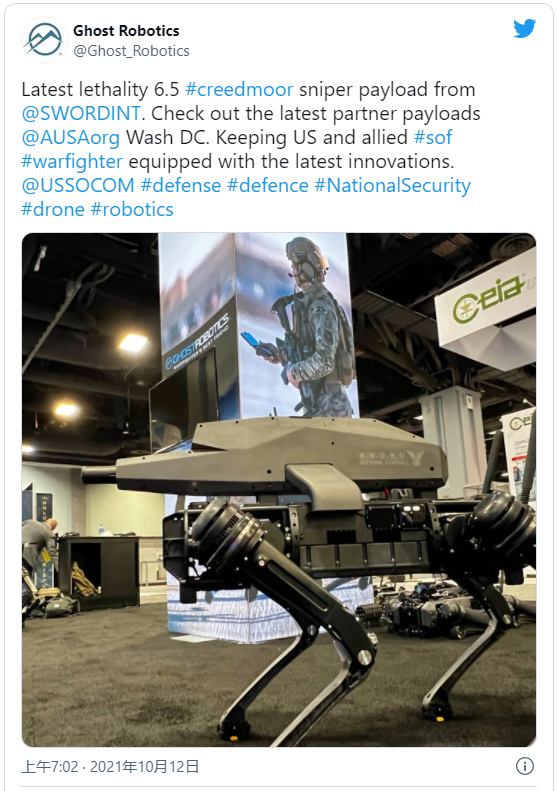
这个机器狗引起网友争议,「持枪机器狗」射程达1200米
编译 | 禾木木 出品 | AI科技大本营(ID:rgznai100) 如果提起自动机器狗,首先想到的应该是波士顿动力,自波士顿动力 Spot 推出以来,机器狗就解锁了很多应用场景。波士顿动力一直都禁止将机器狗武器化。 但是,…

nutch如何发布插件
为什么80%的码农都做不了架构师?>>> 1.修改插件,在原有的插件上修改,比如parse-html插件上修改。 2.修改插件之后,把第三方的包放到/nutch/runtime/local/lib下(经测试,只有在此目录下…
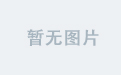
第 7 章 项目运作
comments powered by Disqus 原文出处:Netkiller 系列 手札 本文作者:陈景峯 转载请与作者联系,同时请务必标明文章原始出处和作者信息及本声明。

干货!整理了50个 Pandas 高频使用技巧,强烈建议收藏!
作者 | 俊欣来源 | 关于数据分析与可视化今天小编来分享在pandas当中经常会被用到的方法,篇幅可能有点长但是提供的都是干货,读者朋友们看完之后也可以点赞收藏,相信会对大家有所帮助,大致本文会讲述这些内容DataFrame初印象读取表…

CentOS的Gearman安装与使用无错版
通常,多语言多系统之间的集成是个大问题,一般来说,人们多半会采用WebService的方式来处理此类集成问题,但不管采用何种风格的WebService,如RPC风格,或者REST风格,其本身都有一定的复杂性。相比之…
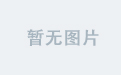
putty或xshell上用vi/vim小键盘无法使用的解决方法
在putty或xshell上用vi/vim的时候,开NumLock时按小键盘上的数字键并不能输入数字,而是出现一个字母然后换行(实际上是命令模式上对应上下左右的键)。解决方法:putty:选项Terminal->Features里,找到Disable applicat…
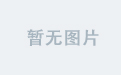
Sqoop数据分析引擎安装与使用
Sqoop数据分析引擎安装与使用>什么是Sqoop ?Sqoop 是一个开源的数据处理引擎,主要是通过 JDBC 为媒介, 在Hadoop(Hive)与 传统的关系型数据库(Oracle, MySQL,Postgres等)间进行数据的传递HDFS Hive HBase < JD…
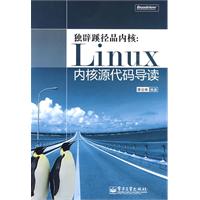
《独辟蹊径品内核:Linux内核源代码导读(china-pub首发)》的前言
我觉得作者讲的学习方法很好值得看看。 下面是本书作者所写: 几乎每一个操作系统内核的学习者在初学阶段都会感觉到难以入门。这是由于内核涉及到知识面非常广泛,需要学习者从根本上掌握大量的知识,这包括:程序编译,链…

95后架构师晒出工资单:狠补了这个,真香...
经常会有很多人说:“不是谁都可以成为架构师的。”“我们公司用的就是那点东西,不需要会太多。”“技术够用就行了。”…其实他们说的不错,但我也总觉得,程序员可以是一个非常热血的职业。即使不是人人都可以成为架构师࿰…

趣味图形之 余弦函数cos与直线相交(另一种相交)
高中的时候做的,前两天看了看,挺好玩的。只想说,当初的代码风格,,,,咳咳,算不上风骚!#include <math.h> #include <stdio.h> int main (void) {double y;int…
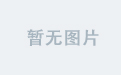
AI时代:推荐引擎正在塑造人类
We shape our tools and afterwards our tools shape us. ------Marshall McLuhan 麦克卢汉说:“我们塑造了工具,反过来工具也在塑造我们。” 我本人不反感AI,也相信人工智能会开创一个伟大的时代,但是我们要思考一些东西…
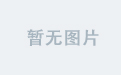
mogileFS 分布式存储-安装手记
环境是centos 呃,装个玩意儿走了好多弯路,以为依赖太多的包河模块,搞了很久. 后来发现其实安装可以简化的,yum没有mogilefs,可以通过epel来安装. 第一种安装方法,用epel # rpm -Uvh http://download.fedora.redhat.com/pub/epel/5/i386/epel-release-5-3.noarch.rpm # yum…
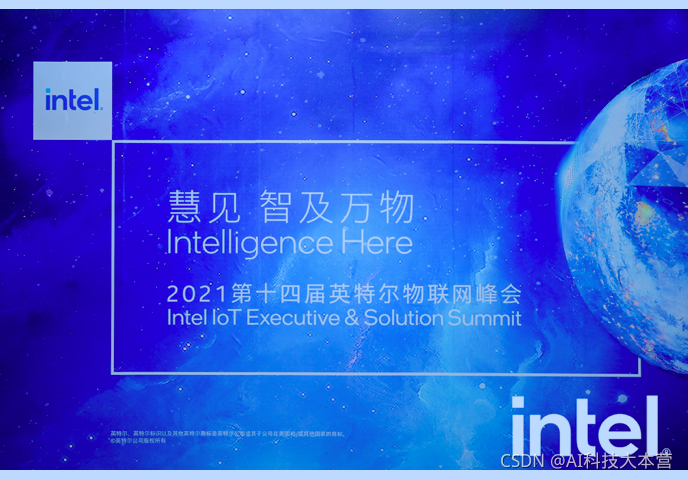
英特尔成立物联网视频事业部,这届IESS还揭露了哪些信息?
随着5G技术的深入发展与落地,物联网已然成为当下炙手可热的技术话题。当万物相互连接,一个潜力丝毫不亚于互联网的市场就此诞生。驱动互联网的可能是网络,可能是算力,也可能是无数个开发者的开源和共享。那么驱动物联网的力量究竟…

JS数字转换成货币格式
2019独角兽企业重金招聘Python工程师标准>>> // Extend the default Number object with a formatMoney() method:// usage: someVar.formatMoney(decimalPlaces, symbol, thousandsSeparator, decimalSeparator)// defaults: (2, "$", ",", &q…
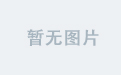
CentOS 部署 flask项目
原文地址 最近在学习 python,使用 flask 实现了个个人博客程序,完了想部署到服务器上。因为是新手,一路磕磕绊绊最终把它基本搞定。网上资料对新手感觉都不太友好,都是零零碎碎的,所以我整理了一下,一方面作…
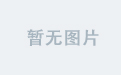
Linux系统JDK安装和配置
以下步骤均为root登录状态下进行执行。 一、卸载JDK Linux会自带JDK,如果不使用自带版本的话需要卸载。 1、卸载系统自带的jdk版本 查看自带的jdk #rpm -qa | grep gcj 看到如下信息: libgcj-4.1.2-44.el5 java-1.4.2-gcj-compat-1.4.2.0-40jpp.1…

4000字,详解 Python 操作 MySQL 数据库!
作者 | 黄伟呢出品 | 数据分析与统计学之美本文的重点,就是教会大家,如何用Python来操作MySQL数据库。1. 通用步骤其实,这里有一个通用步骤,都是写死了的,大家照做就行。# 1. 导入相关库 import pymysql# 2. 链接MySQL…

php跨域共享session
、 $gb_DBHOSTname "127.0.0.1"; //主机的名称或是IP地址 02 $gb_DBname "dbname"; //数据库名称 03 $gb_DBuser "username"; //数据库用户名称 04 $gb_DBpass "pwd"; //数据库密码 05 $gb_COOKIE_DOMAIN .a.com; 06 $SESS_DBH …
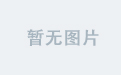
centos6 防火墙iptables操作整理
使用语句 前言: iptables的启动文件位置再: /etc/init.d/iptables , srevice iptables调用的就是这里的执行文件 查看防火墙状态 service iptables status开启防火墙 service iptables start 关闭防火墙 service iptables stop永久关闭防火墙(开机不启动) chkconfig i…